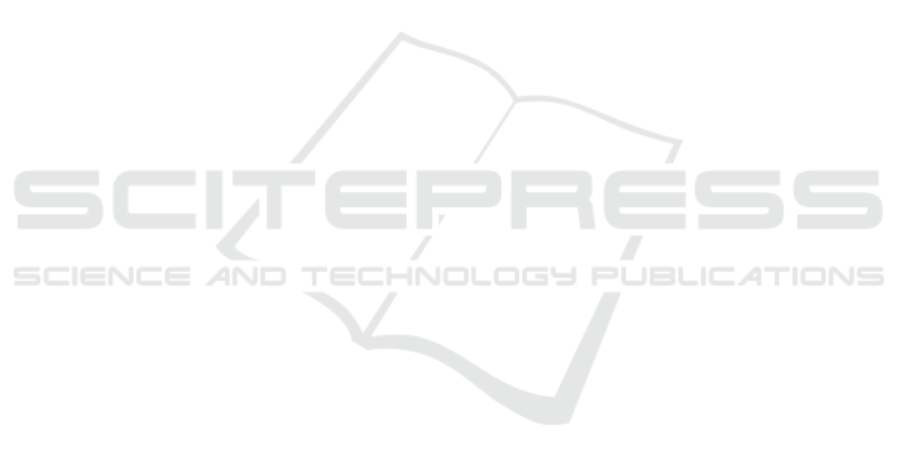
Chaum, D. and van Heyst, E. (1991). Group signatures.
In Davies, D. W., editor, EUROCRYPT 1991, volume
547 of LNCS, pages 257–265. Springer.
Chen, G. and Zhang, Y. (2023). Securing tees with veri-
fiable execution contracts. IEEE TDSC, 20(4):3222–
3237.
Chiang, H.-L., Chia, Y.-Y., Linand, H.-S., and Chen, C.-
H. (2016). The Implications of Tobacco Smoking
on Acute Postoperative Pain: A Prospective Obser-
vational Study. Pain Research and Management,
2016:9432493.
da Gama, M. B., Cartlidge, J., Polychroniadou, A., Smart,
N. P., and Alaoui, Y. T. (2022). Kicking-the-bucket:
Fast privacy-preserving trading using buckets. In
Eyal, I. and Garay, J. A., editors, FC 2022, volume
13411 of LNCS, pages 20–37. Springer.
Evans, D., Kolesnikov, V., and Rosulek, M. (2018). A prag-
matic introduction to secure multi-party computation.
Found. Trends Priv. Secur., 2(2-3):70–246.
Gentry, C. (2009). Fully homomorphic encryption using
ideal lattices. In Mitzenmacher, M., editor, STOC
2009, pages 169–178. ACM.
Geppert, T., Deml, S., Sturzenegger, D., and Ebert, N.
(2022). Trusted execution environments: Applications
and organizational challenges. Frontiers Comput. Sci.,
4.
Goldreich, O., Micali, S., and Wigderson, A. (1987). How
to play any mental game or A completeness theorem
for protocols with honest majority. In Aho, A. V., ed-
itor, STOC 1987, pages 218–229. ACM.
Goldwasser, S., Micali, S., and Rackoff, C. (1985). The
knowledge complexity of interactive proof-systems
(extended abstract). In Sedgewick, R., editor, STOC
1985, pages 291–304. ACM.
Groth, J. (2016). On the size of pairing-based non-
interactive arguments. In Fischlin, M. and Coron, J.,
editors, EUROCRYPT 2016, volume 9666 of LNCS,
pages 305–326. Springer.
Helminger, L. and Rechberger, C. (2022). Multi-party com-
putation in the gdpr. In Schiffner, S., Ziegler, S., and
Quesada Rodriguez, A., editors, Privacy Symposium
2022, pages 21–39. Springer.
Jordan, S., Fontaine, C., and Hendricks-Sturrup, R. (2022).
Selecting privacy-enhancing technologies for manag-
ing health data use. Front. Public Health, 10:814163.
Keller, M. (2020). MP-SPDZ: A versatile framework for
multi-party computation. In Ligatti, J., Ou, X., Katz,
J., and Vigna, G., editors, ACM CCS 2020, pages
1575–1590. ACM.
Kiss, P. (2022). Federated Learning of Artificial Neural
Networks.
Kone
ˇ
cný, J., McMahan, H. B., Yu, F. X., Richtárik, P.,
Suresh, A. T., and Bacon, D. (2016). Federated learn-
ing: Strategies for improving communication effi-
ciency. CoRR, abs/1610.05492.
Li, J., Li, X., and Zhang, C. (2022). Analysis on security
and privacy-preserving in federated learning. High-
lights in Science, Engineering and Technology, 4:349–
358.
Lind, J., Eyal, I., Pietzuch, P. R., and Sirer, E. G. (2016).
Teechan: Payment channels using trusted execution
environments. CoRR, abs/1612.07766.
Liu, T., Xie, X., and Zhang, Y. (2021). zkcnn: Zero knowl-
edge proofs for convolutional neural network predic-
tions and accuracy. In Kim, Y., Kim, J., Vigna, G., and
Shi, E., editors, ACM CCS 2021, pages 2968–2985.
ACM.
López-Alt, A., Tromer, E., and Vaikuntanathan, V. (2012).
On-the-fly multiparty computation on the cloud via
multikey fully homomorphic encryption. In Karloff,
H. J. and Pitassi, T., editors, STOC 2012, pages 1219–
1234. ACM.
Lorünser, T., Wohner, F., and Krenn, S. (2022a). A privacy-
preserving auction platform with public verifiability
for smart manufacturing. In Mori, P., Lenzini, G.,
and Furnell, S., editors, ICISSP 2022, pages 637–647.
SCITEPRESS.
Lorünser, T., Wohner, F., and Krenn, S. (2022b). A ver-
ifiable multiparty computation solver for the linear
assignment problem: And applications to air traffic
management. In Regazzoni, F. and van Dijk, M., edi-
tors, CCSW 2022, pages 41–51. ACM.
McMahan, B., Moore, E., Ramage, D., Hampson, S., and
y Arcas, B. A. (2017). Communication-efficient learn-
ing of deep networks from decentralized data. In
Singh, A. and Zhu, X. J., editors, AISTATS 2017,
volume 54 of Proceedings of Machine Learning Re-
search, pages 1273–1282. PMLR.
Moshawrab, M., Adda, M., Bouzouane, A., Ibrahim, H.,
and Raad, A. (2023). Reviewing federated learn-
ing aggregation algorithms; strategies, contributions,
limitations and future perspectives. Electronics,
12(10):2287.
Mosteiro-Sanchez, A., Barcelo, M., Astorga, J., and Urbi-
eta, A. (2022). Too many options: A survey of ABE
libraries for developers. CoRR, abs/2209.12742.
Muazu, T., Mao, Y., Muhammad, A. U., Ibrahim, M.,
Kumshe, U. M. M., and Samuel, O. (2024). A fed-
erated learning system with data fusion for health-
care using multi-party computation and additive secret
sharing. Comput. Commun., 216:168–182.
Munjal, K. and Bhatia, R. (2022). A systematic review
of homomorphic encryption and its contributions in
healthcare industry. Complex Intell Systems, 3:1–28.
Panzade, P., Takabi, D., and Cai, Z. (2024). Privacy-
preserving machine learning using functional encryp-
tion: Opportunities and challenges. IEEE Internet
Things J., 11(5):7436–7446.
Rivest, R. L., Shamir, A., and Tauman, Y. (2001). How to
leak a secret. In Boyd, C., editor, ASIACRYPT 2001,
volume 2248 of LNCS, pages 552–565. Springer.
Sabt, M., Achemlal, M., and Bouabdallah, A. (2015).
Trusted execution environment: What it is, and what
it is not. In 2015 IEEE Trustcom/BigDataSE/ISPA,
pages 57–64. IEEE.
Sahai, A. and Waters, B. (2005). Fuzzy identity-based en-
cryption. In Cramer, R., editor, EUROCRYPT 2005,
volume 3494 of LNCS, pages 457–473. Springer.
Protecting Privacy in Federated Time Series Analysis: A Pragmatic Technology Review for Application Developers
209