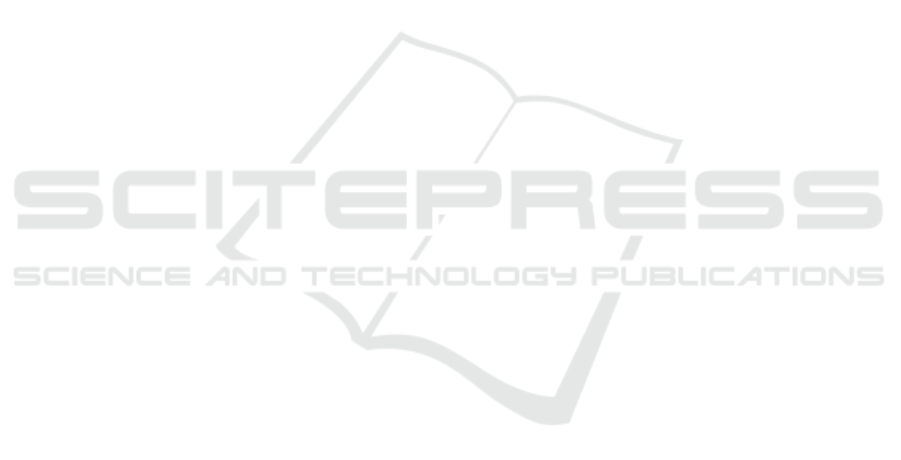
Ghalmane, Z., El Hassouni, M., Cherifi, C., and Cher-
ifi, H. (2018a). K-truss decomposition for modular
centrality. In 2018 9th International Symposium on
Signal, Image, Video and Communications (ISIVC),
pages 241–248. IEEE.
Ghalmane, Z., El Hassouni, M., and Cherifi, H. (2018b).
Betweenness centrality for networks with non-
overlapping community structure. In 2018 IEEE
workshop on complexity in engineering (COMPENG),
pages 1–5. IEEE.
GHALMANE, Z., Zghal, M., et al. (2023). Road networks:
A new filtering approach to extract backbones using
community structure.
Hamilton, W., Ying, Z., and Leskovec, J. (2017). Inductive
representation learning on large graphs. Advances in
neural information processing systems, 30.
Hopman, D., Koole, G., and Mei, R. V. D. (2021). A
machine learning approach to itinerary-level booking
prediction in competitive airline markets. Interna-
tional Journal of Revenue Management, 12(3-4):153–
191.
Iqbal, M., Zhang, K., Iqbal, S., and Tariq, I. (2018). A fast
and reliable dijkstra algorithm for online shortest path.
Int. J. Comput. Sci. Eng, 5(12):24–27.
Jana, D., Malama, S., Narasimhan, S., and Taciroglu, E.
(2023). Edge ranking of graphs in transportation net-
works using a graph neural network (gnn). arXiv
preprint arXiv:2303.17485.
Jiang, W. and Luo, J. (2022). Graph neural network for
traffic forecasting: A survey. Expert systems with ap-
plications, 207:117921.
Kipf, T. N. and Welling, M. (2016). Semi-supervised clas-
sification with graph convolutional networks. arXiv
preprint arXiv:1609.02907.
Latora, V., Nicosia, V., and Russo, G. (2017). Complex net-
works: principles, methods and applications. Cam-
bridge University Press.
Liu, H., Tong, Y., Zhang, P., Lu, X., Duan, J., and Xiong,
H. (2019). Hydra: A personalized and context-aware
multi-modal transportation recommendation system.
In Proceedings of the 25th ACM SIGKDD Interna-
tional Conference on Knowledge Discovery & Data
Mining, pages 2314–2324.
Liu, S. and Jiang, H. (2022). Personalized route recommen-
dation for ride-hailing with deep inverse reinforce-
ment learning and real-time traffic conditions. Trans-
portation Research Part E: Logistics and Transporta-
tion Review, 164:102780.
MOEZZI, R. Enhanced vehicle arrival time prediction with
graph neural networks (gnn) using gps data.
Shafique, S. and Ali, M. E. (2016). Recommending most
popular travel path within a region of interest from
historical trajectory data. In Proceedings of the 5th
ACM SIGSPATIAL International Workshop on Mobile
Geographic Information Systems, pages 2–11.
Shanmukhappa, T., Ho, I. W.-H., Chi, K. T., and Leung,
K. K. (2019). Recent development in public transport
network analysis from the complex network perspec-
tive. IEEE Circuits and Systems Magazine, 19(4):39–
65.
Sharma, A., Sharma, A., Nikashina, P., Gavrilenko, V., Tse-
lykh, A., Bozhenyuk, A., Masud, M., and Meshref, H.
(2023). A graph neural network (gnn)-based approach
for real-time estimation of traffic speed in sustainable
smart cities. Sustainability, 15(15):11893.
Song, C., Guan, W., and Ma, J. (2018). Potential travel
cost saving in urban public-transport networks using
smartphone guidance. Plos one, 13(5):e0197181.
Termos, M., Ghalmane, Z., Fadlallah, A., Jaber, A.,
Zghal, M., et al. (2023). Intrusion detection system
for iot based on complex networks and machine
learning. In 2023 IEEE Intl Conf on Depend-
able, Autonomic and Secure Computing, Intl Conf
on Pervasive Intelligence and Computing, Intl
Conf on Cloud and Big Data Computing, Intl
Conf on Cyber Science and Technology Congress
(DASC/PiCom/CBDCom/CyberSciTech), pages
0471–0477. IEEE.
Termos, M., Ghalmane, Z., Fadlallah, A., Jaber, A., Zghal,
M., et al. (2024). Gdlc: A new graph deep learn-
ing framework based on centrality measures for in-
trusion detection in iot networks. Internet of Things,
26:101214.
Wang, Y., Yin, H., Chen, H., Wo, T., Xu, J., and Zheng, K.
(2019). Origin-destination matrix prediction via graph
convolution: a new perspective of passenger demand
modeling. In Proceedings of the 25th ACM SIGKDD
international conference on knowledge discovery &
data mining, pages 1227–1235.
Wei, L.-Y., Zheng, Y., and Peng, W.-C. (2012). Con-
structing popular routes from uncertain trajectories. In
Proceedings of the 18th ACM SIGKDD international
conference on Knowledge discovery and data mining,
pages 195–203.
Yu, Y., Si, X., Hu, C., and Zhang, J. (2019). A review of
recurrent neural networks: Lstm cells and network ar-
chitectures. Neural computation, 31(7):1235–1270.
Yuan, J., Zheng, Y., Zhang, C., Xie, W., Xie, X., Sun, G.,
and Huang, Y. (2010). T-drive: driving directions
based on taxi trajectories. In Proceedings of the 18th
SIGSPATIAL International conference on advances in
geographic information systems, pages 99–108.
Zanin, M. and Lillo, F. (2013). Modelling the air transport
with complex networks: A short review. The Euro-
pean Physical Journal Special Topics, 215(1):5–21.
Zhang, S., Tong, H., Xu, J., and Maciejewski, R. (2019).
Graph convolutional networks: a comprehensive re-
view. Computational Social Networks, 6(1):1–23.
IoTBDS 2025 - 10th International Conference on Internet of Things, Big Data and Security
320