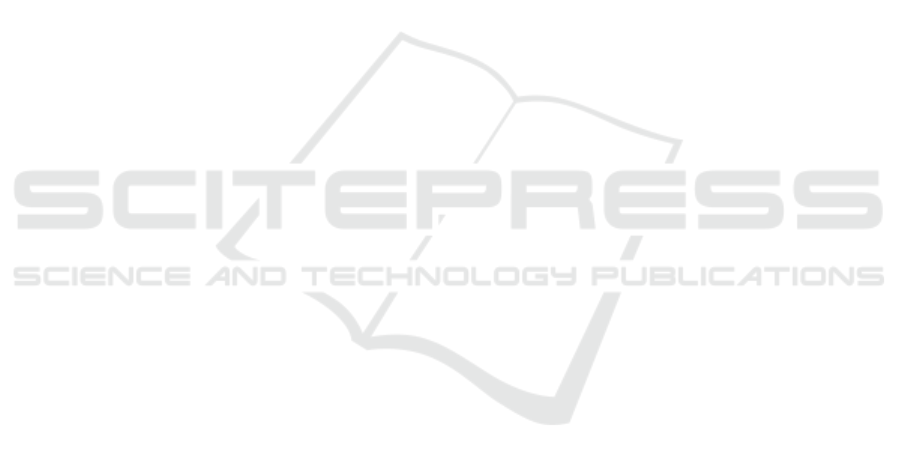
der to ensure that each GISB provides a fast frequency
support, while counteracting the presence of commu-
nication delays. The delay stability margin has been
found by means of an extensive simulation campaign,
which has also exploited the latin hypercube sampling
technique to prove the resilience of the proposed con-
troller with respect to parameters and delays uncer-
tainties.
REFERENCES
Andreotti, A., Caiazzo, B., Petrillo, A., and Santini,
S. (2021). Distributed robust finite-time secondary
control for stand-alone microgrids with time-varying
communication delays. IEEE Access, 9:59548–
59563.
Caiazzo, B., Lui, D. G., Petrillo, A., and Santini, S. (2022).
Cooperative finite-time control for autonomous ve-
hicles platoons with nonuniform v2v communication
delays. IFAC-PapersOnLine, 55(36):145–150.
Chen, C., Wang, J., and Kishore, S. (2014). A distributed
direct load control approach for large-scale residential
demand response. IEEE Transactions on Power Sys-
tems, 29(5):2219–2228.
Duan, Q., Zeng, K., Liu, J., Du, B., and Liao, P. (2022). Ag-
gregated control of smart building units for frequency
regulation in multi-region power systems. In 2022 7th
Asia Conference on Power and Electrical Engineering
(ACPEE), pages 1209–1214. IEEE.
Ge, X., Han, Q.-L., Ding, D., Zhang, X.-M., and Ning, B.
(2018). A survey on recent advances in distributed
sampled-data cooperative control of multi-agent sys-
tems. Neurocomputing, 275:1684–1701.
Helton, J. C. and Davis, F. J. (2003). Latin hypercube sam-
pling and the propagation of uncertainty in analyses
of complex systems. Reliability Engineering & Sys-
tem Safety, 81(1):23–69.
Liu, X., Li, Y., Lin, X., Guo, J., Shi, Y., and Shen, Y. (2022).
Dynamic bidding strategy for a demand response ag-
gregator in the frequency regulation market. Applied
Energy, 314:118998.
Wadi, M., Shobole, A., Elmasry, W., and Kucuk, I. (2024).
Load frequency control in smart grids: A review of
recent developments. Renewable and Sustainable En-
ergy Reviews, 189:114013.
Wang, Y., Tang, Y., Xu, Y., and Xu, Y. (2019a). A dis-
tributed control scheme of thermostatically controlled
loads for the building-microgrid community. IEEE
Transactions on Sustainable Energy, 11(1):350–360.
Wang, Y., Xu, Y., and Tang, Y. (2019b). Distributed ag-
gregation control of grid-interactive smart buildings
for power system frequency support. Applied energy,
251:113371.
Wu, Y., Wei, Z., Weng, J., Li, X., and Deng, R. H. (2017).
Resonance attacks on load frequency control of smart
grids. IEEE Transactions on Smart Grid, 9(5):4490–
4502.
Xia, M., Song, Y., and Chen, Q. (2019). Hierarchical con-
trol of thermostatically controlled loads oriented smart
buildings. Applied energy, 254:113493.
Xiao, H., Zhang, M., Zeng, L., Wu, G., Wu, C., and Wu,
C. (2023). Hierarchical control strategy of thermo-
statically controlled load considering multiple factors.
Energy and Buildings, 291:113148.
Yousef, H. A., Khalfan, A.-K., Albadi, M. H., and Hossein-
zadeh, N. (2014). Load frequency control of a multi-
area power system: An adaptive fuzzy logic approach.
IEEE transactions on power systems, 29(4):1822–
1830.
Zhang, C., Xu, Y., Li, Z., and Dong, Z. Y. (2018). Ro-
bustly coordinated operation of a multi-energy micro-
grid with flexible electric and thermal loads. IEEE
Transactions on Smart Grid, 10(3):2765–2775.
Zhao, H., Wu, Q., Huang, S., Zhang, H., Liu, Y., and Xue,
Y. (2016). Hierarchical control of thermostatically
controlled loads for primary frequency support. IEEE
Transactions on Smart Grid, 9(4):2986–2998.
Zhao, N., Yue, D., Dou, C., and Shi, T. (2023). Distributed
dynamic event-triggered cooperative control of mul-
tiple tcls and hess for improving frequency regula-
tion. IEEE Transactions on Industrial Informatics,
20(2):1539–1549.
Zheng, L., An, Z., Xia, Q., Kandula, R. P., Saeedifard, M.,
Divan, D., Grijalva, S., and Abdallah, C. T. (2021).
Distributed control of aggregated smart buildings for
frequency regulation. In 2021 IEEE Industry Applica-
tions Society Annual Meeting (IAS), pages 1–8. IEEE.
Grid Interactive Smart Buildings Coordination in Multi-Area Power Systems: A Delay-Robustness Analysis
55