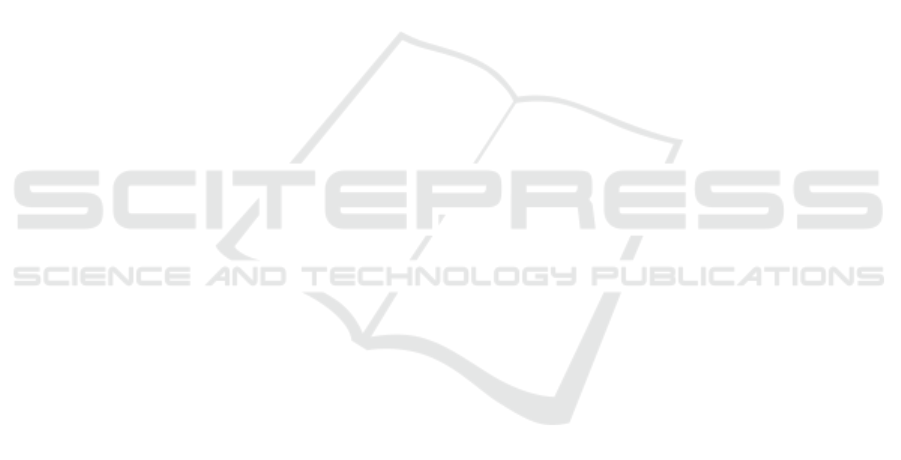
REFERENCES
Aken, J. E. (2004). Management research based on the
paradigm of the design sciences: The quest for field-
tested and grounded technological rules. Journal of
Management Studies, 41(2):219–246.
Alves, G. M. R. and Maciel, A. M. A. (2023). Survey on
Data Ingestion for AutoML (S). In Proceedings of
the International Conference on Software Engineering
and Knowledge Engineering, pages 411–414.
Caroli, P. (2018). Lean Inception: como alinhar pessoas e
construir o produto certo. Editora Caroli.
Chen, Y.-W., Song, Q., and Hu, X. (2021). Techniques for
Automated Machine Learning. ACM SIGKDD Explo-
rations Newsletter, 22(2):35–50.
da Silva, R. (2020). Desenvolvimento de uma Soluc¸
˜
ao
de Aprendizado de M
´
aquina Automatizado Integr
´
avel
a M
´
ultiplos Ambientes Virtuais de Aprendizagem.
Master’s thesis, Universidade de Pernambuco, Recife.
Elshawi, R., Maher, M., and Sakr, S. (2019). Automated
Machine Learning: State-of-The-Art and Open Chal-
lenges. arXiv:1906.02287 [cs, stat].
Garcia, V. C. (2010). RiSE reference model for software
reuse adoption in brazilian companies.
Gonc¸alves, A. F., Maciel, A. M., and Rodrigues, R. L.
(2017). Development of a data mining education
framework for visualization of data in distance learn-
ing environments. International Conferences on Soft-
ware Engineering and Knowledge Engineering.
H. R. Mac
ˆ
edo, P., B. Santos, W., and M. A. Maciel, A.
(2021). An
´
alise de Perfis de Engajamento de Estu-
dantes de Ensino a Dist
ˆ
ancia. RENOTE, 18(2):326–
335.
Hapke, H. and Nelson, C. (2020). Building Machine Learn-
ing Pipelines: Automating model life cycles with ten-
sorflow. O’Reilly Media, Sebastopol, CA.
Hassenzahl, M., Platz, A., Burmester, M., and Lehner, K.
(2000). Hedonic and ergonomic quality aspects de-
termine a software’s appeal. In Proceedings of the
SIGCHI conference on Human Factors in Computing
Systems, pages 201–208, The Hague The Netherlands.
ACM.
Hlupic, T. and Punis, J. (2021). An Overview of Cur-
rent Trends in Data Ingestion and Integration. In
2021 44th International Convention on Information,
Communication and Electronic Technology (MIPRO),
pages 1265–1270, Opatija, Croatia. IEEE.
Hutter, F., Kotthoff, L., and Vanschoren, J., editors (2019).
Automated Machine Learning: Methods, Systems,
Challenges. The Springer Series on Challenges in
Machine Learning. Springer International Publishing,
Cham.
Ming Li and Smidts, C. (2003). A ranking of software en-
gineering measures based on expert opinion. IEEE
Transactions on Software Engineering, 29(9):811–
824.
Nagarajah, T. and Poravi, G. (2019). A Review on Au-
tomated Machine Learning (AutoML) Systems. In
2019 IEEE 5th International Conference for Conver-
gence in Technology (I2CT), pages 1–6, Bombay, In-
dia. IEEE.
Patel, J. (2020). The Democratization of Machine Learning
Features. In 2020 IEEE 21st International Confer-
ence on Information Reuse and Integration for Data
Science (IRI), pages 136–141, Las Vegas, NV, USA.
IEEE.
Ray, S., AlGhamdi, A., AlGhamdi, A., Alshouiliy, K., and
Agrawal, D. P. (2020). Selecting Features for Breast
Cancer Analysis and Prediction. In 2020 International
Conference on Advances in Computing and Communi-
cation Engineering (ICACCE), pages 1–6, Las Vegas,
NV, USA. IEEE.
Saurav, S. and Schwarz, P. (2016). A Machine-Learning
Approach to Automatic Detection of Delimiters in
Tabular Data Files. In 2016 IEEE 18th Inter-
national Conference on High Performance Com-
puting and Communications; IEEE 14th Interna-
tional Conference on Smart City; IEEE 2nd Inter-
national Conference on Data Science and Systems
(HPCC/SmartCity/DSS), pages 1501–1503, Sydney,
Australia. IEEE.
Tidwell, J., Brewer, C., and Valencia, A. (2020). Designing
interfaces: Patterns for effective interaction design.
O’Reilly Media, Sebastopol, CA.
Whalen, J. (2019). Design for How People Think: Using
Brain Science to Build Better Products. O’Reilly Me-
dia, Sebastopol, CA.
Z
¨
oller, M.-A. and Huber, M. F. (2021). Benchmark and
Survey of Automated Machine Learning Frameworks.
Journal of Artificial Intelligence Research, 70:409–
472.
ICEIS 2025 - 27th International Conference on Enterprise Information Systems
588