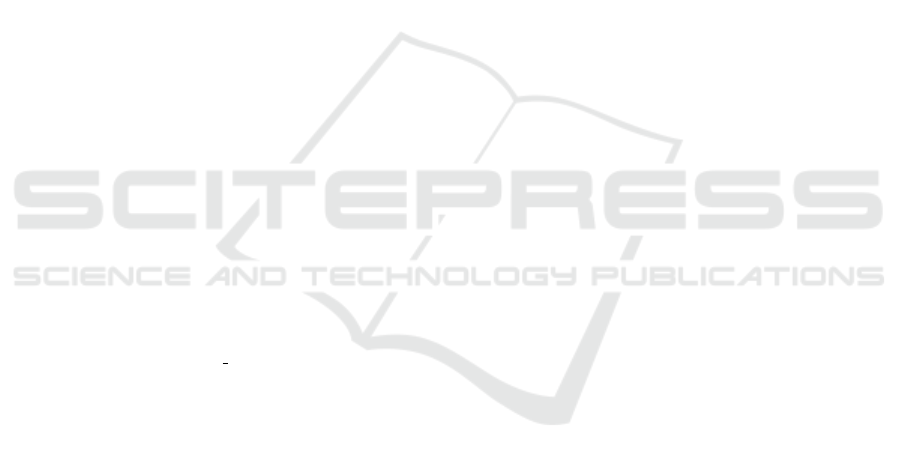
Within this paper it was obtained satisfactory re-
sults, making a comparison with related work it can
be seen that the results obtained are good. The find-
ings of this research contribute to the growing body
of knowledge about machine learning applications in
the medical field and provide a base for future studies
aimed at improving medical practices and improving
communication with patients.
Future work would consist of creating a bigger
data set and testing and validating the models cre-
ated in this paper on this new data set, respectively,
trying to check what performance could be obtained
with other ML approaches.
REFERENCES
Abadi, M., Agarwal, A., Barham, P., Brevdo, E., Chen, Z.,
Citro, C., Corrado, G. S., Davis, A., Dean, J., Devin,
M., Ghemawat, S., Goodfellow, I., Harp, A., Irving,
G., Isard, M., Jia, Y., Jozefowicz, R., Kaiser, L., Kud-
lur, M., Levenberg, J., Man
´
e, D., Monga, R., Moore,
S., Murray, D., Olah, C., Schuster, M., Shlens, J.,
Steiner, B., Sutskever, I., Talwar, K., Tucker, P., Van-
houcke, V., Vasudevan, V., Vi
´
egas, F., Vinyals, O.,
Warden, P., Wattenberg, M., Wicke, M., Yu, Y., and
Zheng, X. (2015). TensorFlow: Large-scale machine
learning on heterogeneous systems. Software avail-
able from tensorflow.org.
Almeida, L. B. (2020). Multilayer perceptrons. In Hand-
book of Neural Computation, pages C1–2. CRC Press.
Altamimi, I., Altamimi, A., Alhumimidi, A. S., Altamimi,
A., and Temsah, M.-H. (2023). Artificial intelligence
(ai) chatbots in medicine: a supplement, not a substi-
tute. Cureus, 15(6).
Anh, B. H. Q. (2024). Disease symptoms, @ONLINE.
Babu, A. and Boddu, S. B. (2024). Bert-based medical chat-
bot: Enhancing healthcare communication through
natural language understanding. Exploratory Re-
search in Clinical and Social Pharmacy, 13:100419.
Bali, M., Mohanty, S., Chatterjee, S., Sarma, M., and Pura-
vankara, R. (2019). Diabot: a predictive medical chat-
bot using ensemble learning. International Journal of
Recent Technology and Engineering, 8(2):6334–6340.
Bird, S., Klein, E., and Loper, E. (2009). Natural language
processing with Python: analyzing text with the natu-
ral language toolkit. ” O’Reilly Media, Inc.”.
Chakraborty, S., Paul, H., Ghatak, S., Pandey, S. K., Ku-
mar, A., Singh, K. U., and Shah, M. A. (2022). An
ai-based medical chatbot model for infectious disease
prediction. Ieee Access, 10:128469–128483.
Costa, V. G. and Pedreira, C. E. (2023). Recent advances
in decision trees: An updated survey. Artificial Intel-
ligence Review, 56(5):4765–4800.
Hapke, H., Howard, C., and Lane, H. (2019). Natural
Language Processing in Action: Understanding, an-
alyzing, and generating text with Python. Simon and
Schuster.
Karuna, G., Reddy, G. G., Sushmitha, J., Gayathri, B.,
Sharma, S. D., and Khatua, D. (2023). Helpi–an auto-
mated healthcare chatbot. In E3S Web of Conferences,
volume 430, page 01040. EDP Sciences.
Patil, P. (2024). Symptoms and diseases dataset @ON-
LINE.
Pedregosa, F., Varoquaux, G., Gramfort, A., Michel, V.,
Thirion, B., Grisel, O., Blondel, M., Prettenhofer,
P., Weiss, R., Dubourg, V., et al. (2011). Scikit-
learn: Machine learning in python. Journal of ma-
chine learning research, 12(Oct):2825–2830.
Polignano, M., Narducci, F., Iovine, A., Musto, C.,
De Gemmis, M., and Semeraro, G. (2020). Healthas-
sistantbot: a personal health assistant for the italian
language. IEEE Access, 8:107479–107497.
Probst, P., Wright, M. N., and Boulesteix, A.-L. (2019). Hy-
perparameters and tuning strategies for random for-
est. Wiley Interdisciplinary Reviews: data mining and
knowledge discovery, 9(3):e1301.
Reddy, E. M. K., Gurrala, A., Hasitha, V. B., and Kumar, K.
V. R. (2022). Introduction to naive bayes and a review
on its subtypes with applications. Bayesian reasoning
and gaussian processes for machine learning applica-
tions, pages 1–14.
Shedthi B, S., Shetty, V., Chadaga, R., Bhat, R., Bangera, P.,
and Kini K, P. (2023). Implementation of chatbot that
predicts an illness dynamically using machine learn-
ing techniques. International Journal of Engineering,
(Articles in Press).
Siddique, S. and Chow, J. C. (2021). Machine learning in
healthcare communication. Encyclopedia, 1(1):220–
239.
Tode, V., Gadge, H., Madane, S., Kachare, P., and Deokar,
A. (2021). A chatbot for medical purpose using deep
learning. International Journal of Engineering Re-
search & Technology.
Vasileiou, M. V. and Maglogiannis, I. G. (2022). The health
chatbots in telemedicine: Intelligent dialog system for
remote support. Journal of Healthcare Engineering,
2022(1):4876512.
Yuan, H. and Yu, S. (2021). Efficient symptom inquiring
and diagnosis via adaptive alignment of reinforcement
learning and classification.
Yuan, H. and Yu, S. (2024). Efficient symptom inquiring
and diagnosis via adaptive alignment of reinforcement
learning and classification. Artificial Intelligence in
Medicine, 148:102748.
Zabor, E. C., Reddy, C. A., Tendulkar, R. D., and Patil, S.
(2022). Logistic regression in clinical studies. In-
ternational Journal of Radiation Oncology* Biology*
Physics, 112(2):271–277.
Zhang, Y., Chen, Q., Yang, Z., Lin, H., and Lu, Z. (2019).
Biowordvec, improving biomedical word embeddings
with subword information and mesh. Scientific data,
6(1):52.
Medical Chatbot for Disease Prediction Using Machine Learning and Symptom Analysis
607