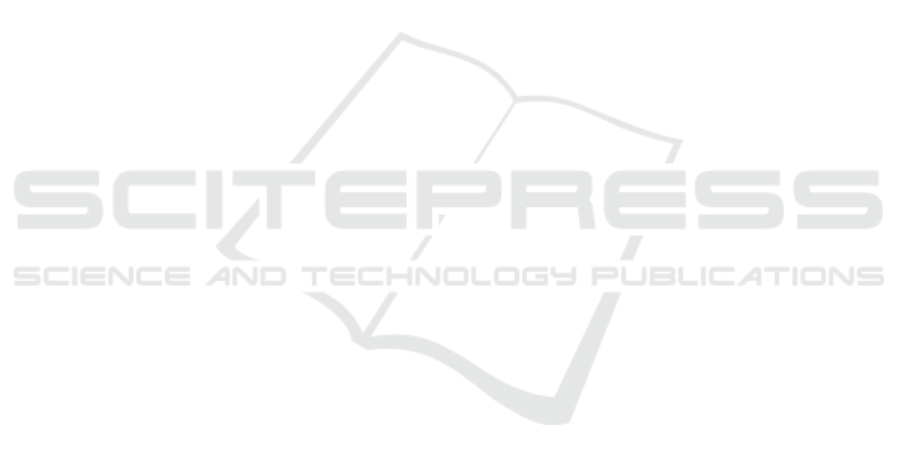
4 MDDT DURING SYSTEM
LIFECYCLE
In this section, we describe how the MDDT and the
system are co-evolving together beyond the design
phase. The overall lifecycle is given in Figure 3. It
shows the successive steps starting from the design
phase (see Section 3) to the operationalization and en-
hancement of the system. We did not mention the de-
commissioning and recycling of the system, which is
currently out of the scope of our study. The typical
process is organized as follows: the two first step Re-
quire and Model in Figure 3 corresponds to the first
modelling layer of Figure 2. The Execute phase, uses
the executable or simulated models. The two middle
layers in Figure 2 are used in order to explore design
choices and evaluate the system before it is in produc-
tion. A system and its DT support are deployed at the
beginning of the Align step of Figure 3. This step al-
lows for a progressive alignment of the MDDT to the
system, keeping it up to date according to integration
of operational data. Finally, the Exploit phase takes
place when the MDDT reaches an acceptable level of
fidelity to the system in operation: this level can be re-
lated to a margin of error in the frame of the sensor’s
known errors margin.
When it reached this level of maturity, the MDDT
can deliver its different services: monitoring, predic-
tion, enhancement of the system, etc. After that, it is
possible to enter a new cycle, by extending the system
(Extend phase in Figure 3) or to Export the system in
another context (e.g., another country with other elec-
tricity production profile). In our approach, the exten-
sion is systemically made under a modelling approach
(i.e., Model phase). In the following subsections, we
will focus on the phase that occurs after the design:
DT alignment and exploitation/operationalization, ex-
tension and transferability.
4.1 Alignment and Exploitation
Once we have reached a first deployment of the sys-
tem, we can officially talk about Digital twin: but the
design models of the previous phases should already
behave as close as possible to a real DT. In practice, it
will be necessary to refactor the DT models (keeping
in mind that the system may only be partially opera-
tional), some model flexibility (Sottet and Biri, 2016)
will be necessary to ensure that both the DT and the
physical system could operate together at any time,
even if they are not fully aligned. The Align phase
will consist in a progressive convergence of the twin
and the system based on the feedback from sensed
data. It will consist in analyzing the drifts (David
and Bork, 2023) that could occur between system and
data. The drift can show some valid discrepancy (i.e.,
the MDDT is not aligned with the system) or invalid
due to a sensor defect (Abbasi et al., 2024).
In the Exploit phase, the MDDT could monitor
the system according to data collection support con-
nected to its models. The MDDT relies largely on
models at run time, the models being the represen-
tation of the run-time events, keeping an image of
the state of the system. The complete usefulness of
MDDT as a decision-support tool could occur once
this stage is reached: notably providing realistic simu-
lation of potential future event (what if scenario), pre-
diction of error, optimization of the system, etc.
Ultimately, this progressive alignment all along
the exploitation will hopefully enhance the accuracy
of the MDDT models. but could also foster for a hy-
brid approach of model-driven and data-driven, e.g.,
by replacing a function by a learned model (Sottet
et al., 2022).
4.2 Extending the System
Once the system and the MDDT are aligned, after one
or more iteration(s) of the previous cycle, the system
can be expanded (represented in Figure 3 with pro-
gressive extensions of considered system in the cen-
ter of the cycles). In our example, we first design
the Hydrogen production (without considering green
energy) and try to assess the quantity for the direct
consumers (public transportation, factories) as well
as the potential return on investment. This first cy-
cle is representative of the initial design of a MDDT
along its system. As represented in blue in Figure 3,
the two first steps are purely models (see Section 3)
then the systems and the MDDT are co-existing and
co-evolving in the green part.
We can extend the initial hydrogen producer-
consumer, in a second cycle (middle of Figure 3),
with the indirect beneficiary of the Hydrogen produc-
tion (public heating network and recoverable O
2
pro-
duction); and similarly evaluate the global value and
return on investment. This cycle is completely green,
meaning that from that point on, MDDT and the sys-
tem are co-existing. Nevertheless, during the Model
and Execute phases, the MDDT works in a hybrid
mode: one part is aligned with the current system and
the other part is under study (similar to design phase).
We then have to rely on the models to assess if the
system is sustainable (i.e., from business to technical
perspectives) and for its implementation.
Finally, as illustrated in Figure 3, the final visible
cycle involves integrating green energy production to
evaluate whether the demand can be met with the
How to Leverage Digital Twin for System Design?
309