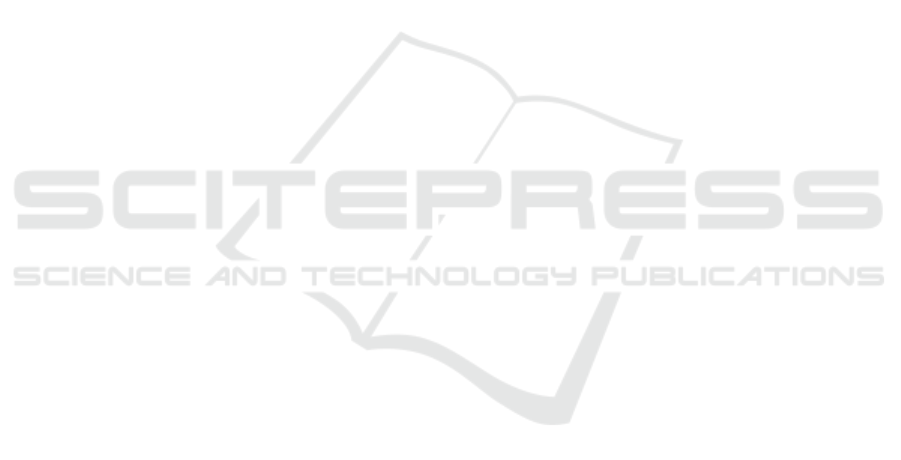
Fontana, F., Ferme, V., Zanoni, M., and Yamashita, A.
(2015b). Automatic metric thresholds derivation for
code smell detection. In 6th Int. Workshop on Emerg-
ing Trends in Software Metrics, pages 44–53. IEEE.
Fowler, M. (2018). Refactoring: improving the design of
existing code. Addison-Wesley Professional.
Gligoric, M., Groce, A., Zhang, C., Sharma, R., Alipour,
M., and Marinov, D. (2013). Comparing non-adequate
test suites using coverage criteria. In Int. Symposium
on Software Testing and Analysis, pages 302–313.
Gondra, I. (2008). Applying machine learning to software
fault-proneness prediction. Journal of Systems and
Software, 81(2):186–195.
Hemmati, H. (2015). How effective are code coverage crite-
ria? In International Conference on Software Quality,
Reliability and Security, pages 151–156. IEEE.
Hossain, M. (2018). Challenges of software quality assur-
ance and testing. International Journal of Software
Engineering and Computer Systems, 4(1):133–144.
Inozemtseva, L. and Holmes, R. (2014). Coverage is not
strongly correlated with test suite effectiveness. In
36th ICSE, pages 435–445. ACM.
Jansen, T., Pastor Ric
´
os, F., Luo, Y., van der Vlist, K., van
Dalen, R., Aho, P., and Vos, T. (2022). Scriptless gui
testing on mobile applications. In IEEE QRS.
Jerzyk, M. and Madeyski, L. (2023). Code smells: A com-
prehensive online catalog and taxonomy. In Devel-
opments in Information and Knowledge Management
Systems for Business Applications. Springer.
Kochhar, P., Thung, F., and Lo, D. (2015). Code coverage
and test suite effectiveness: Empirical study with real
bugs in large systems. In 22nd SANER. IEEE.
Madeyski, L. (2010). The impact of test-first programming
on branch coverage and mutation score indicator of
unit tests: An experiment. Information and Software
Technology, 52(2):169–184.
Martino, S., Fasolino, A., Starace, L., and Tramontana, P.
(2024). GUI testing of android applications: Investi-
gating the impact of the number of testers on different
exploratory testing strategies. J. Softw. Evol. Process.
Memon, A. (2002). Gui testing: Pitfalls and process. Com-
puter, 35(08):87–88.
Memon, A. and Xie, Q. (2005). Studying the fault-detection
effectiveness of gui test cases for rapidly evolving
software. IEEE transactions on software engineering.
Olbrich, S., Cruzes, D., and Sjøberg, D. (2010). Are all
code smells harmful? a study of god classes and brain
classes in the evolution of three open source systems.
In Int. conf. on software maintenance. IEEE.
Pastor Ric
´
os, F., Aho, P., Vos, T., Torres, I., Cal
´
as, E., and
Mart
´
ınez, H. (2020). Deploying testar to enable re-
mote testing in an industrial ci pipeline: a case-based
evaluation. In 9th ISoLA, pages 543–557. Springer.
Pastor Ric
´
os, F., Mar
´
ın, B., Prasetya, I., Vos, T., Davidson,
J., and Hovorka, K. (2024). An industrial experience
leveraging the iv4xr framework for bdd testing of a 3d
sandbox game. In 18th RCIS. Springer.
Pereira dos Reis, J., Brito e Abreu, F.,
de Figueiredo Carneiro, G., and Anslow, C. (2022).
Code smells detection and visualization: a systematic
literature review. Archives of Computational Methods
in Engineering, 29(1):47–94.
Pradhan, S., Ray, M., and Patnaik, S. (2019). Coverage cri-
teria for state-based testing: A systematic review. In-
ternational Journal of Information Technology Project
Management (IJITPM), 10(1):1–20.
Rahman, M., Ahammed, T., Joarder, M., and Sakib, K.
(2023). Does code smell frequency have a relationship
with fault-proneness? In 27th EASE, pages 261–262.
Rodr
´
ıguez-Valdes, O., Vos, T., Aho, P., and Mar
´
ın, B.
(2021). 30 years of automated gui testing: a biblio-
metric analysis. In QUATIC, pages 473–488. Springer.
Sae-Lim, N., Hayashi, S., and Saeki, M. (2018). Context-
based approach to prioritize code smells for prefactor-
ing. Journal of Software: Evolution and Process.
Spadini, D., Palomba, F., Zaidman, A., Bruntink, M., and
Bacchelli, A. (2018). On the relation of test smells to
software code quality. In 34th ICSME. IEEE.
Staats, M., Gay, G., Whalen, M., and Heimdahl, M. (2012).
On the danger of coverage directed test case genera-
tion. In 15th FASE, pages 409–424. Springer.
Tengeri, D., Besz
´
edes,
´
A., Gergely, T., Vid
´
acs, L., Havas,
D., and Gyim
´
othy, T. (2015). Beyond code cover-
age—an approach for test suite assessment and im-
provement. In 8th ICST Workshops, pages 1–7. IEEE.
Tramontana, P., Amalfitano, D., Amatucci, N., Memon, A.,
and Fasolino, A. (2019). Developing and evaluating
objective termination criteria for random testing. ACM
Trans. Softw. Eng. Methodol., 28(3).
van der Brugge, A., Pastor-Ric
´
os, F., Aho, P., Mar
´
ın, B.,
and Vos, T. (2021). Evaluating testar’s effectiveness
through code coverage. XXV Jornadas de Ingenier
´
ıa
del Software y Bases de Datos (JISBD), pages 1–14.
Vargas, N., Mar
´
ın, B., and Giachetti, G. (2021). A list of
risks and mitigation strategies in agile projects. In
40th Int. Conf. SCCC, pages 1–8. IEEE.
Vos, T., Aho, P., Pastor Ricos, F., Rodriguez-Valdes, O.,
and Mulders, A. (2021). TESTAR – scriptless test-
ing through graphical user interface. Software Testing,
Verification and Reliability, 31(3).
Vos, T., Mar
´
ın, B., Escalona, M., and Marchetto, A. (2012).
A methodological framework for evaluating software
testing techniques and tools. In 12th int. conference
on quality software, pages 230–239. IEEE.
Wang, W., Li, D., Yang, W., Cao, Y., Zhang, Z., Deng, Y.,
and Xie, T. (2018). An empirical study of android test
generation tools in industrial cases. In 33rd ASE.
Wieringa, R. and Daneva, M. (2015). Six strategies for
generalizing software engineering theories. Science
of computer programming, 101:136–152.
Wohlin, C., Runeson, P., H
¨
ost, M., Ohlsson, M., Regnell,
B., and Wessl
´
en, A. (2012). Experimentation in soft-
ware engineering. Springer.
Yu, S., Fang, C., Li, X., Ling, Y., Chen, Z., and Su,
Z. (2024). Effective, platform-independent gui test-
ing via image embedding and reinforcement learn-
ing. ACM Transactions on Software Engineering and
Methodology, 33(7).
ENASE 2025 - 20th International Conference on Evaluation of Novel Approaches to Software Engineering
148