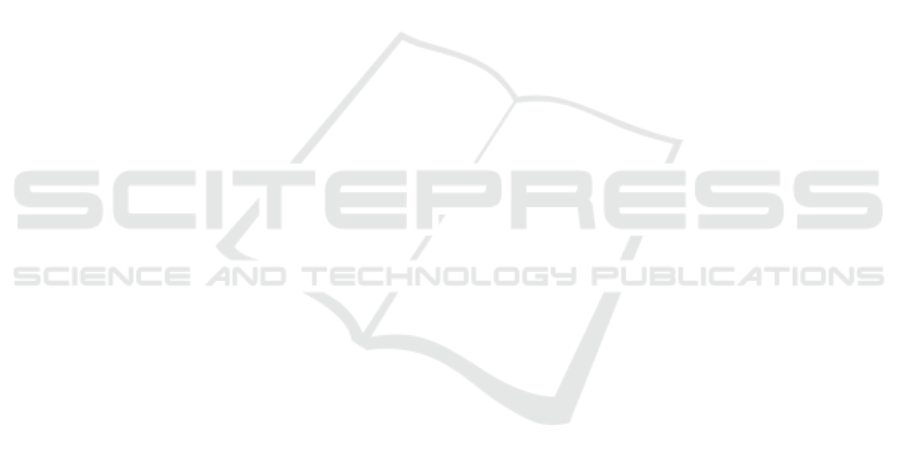
4 CONCLUSIONS
This study analyzed the feasibility of transitioning
Milan’s free-floating car-sharing fleet from ICEVs to
BEVs. Through a scenario-based simulation, it con-
sidered the interplay between vehicle energy auton-
omy, charging infrastructure distribution, and user de-
mand.
The results demonstrate that the integration of
BEVs into car-sharing services is achievable, pro-
vided that charging infrastructure is strategically
planned. Battery swapping and high-power conduc-
tive charging (110 kW) emerged as the most effec-
tive solutions for ensuring high operational perfor-
mance, with feasible rental percentages exceeding
90%. However, these solutions also face significant
implementation challenges, including infrastructure
costs and compatibility issues.
Mid-tier options, such as 22 kW conductive and
11 kW inductive charging, present a balanced com-
promise, offering acceptable performance with lower
costs and scalability. Moreover, the study under-
scores the importance of maintaining short distances
between rental endpoints requiring charging and the
nearest charging stations, ensuring alignment with
user convenience thresholds.
Ultimately, the findings highlight the need for a
tiered and phased deployment strategy for charging
infrastructure to support the successful electrification
of car-sharing fleets. This approach can maximize en-
vironmental benefits while ensuring operational feasi-
bility, positioning Milan as a model city for sustain-
able urban mobility.
ACKNOWLEDGEMENTS
This study was carried out within the MOST –
Sustainable Mobility Center and received funding
from the European Union Next-GenerationEU (PI-
ANO NAZIONALE DI RIPRESA E RESILIENZA
(PNRR) – MISSIONE 4 COMPONENTE 2, IN-
VESTIMENTO 1.4 – D.D. 1033 17/06/2022,
CN00000023). This manuscript reflects only the
authors’ views and opinions, neither the European
Union nor the European Commission can be consid-
ered responsible for them.
REFERENCES
Cederqvist, M. (2023). The carsharing telematics market.
Technical report, Berg Insight AB.
Colombo, C. G., Borghetti, F., Longo, M., and Foiadelli,
F. (2023). Electrification of motorway network: A
methodological approach to define location of charg-
ing infrastructure for ev. Sustainability, 15(23).
Electromaps (2024). https://www.electromaps.com/it.
Genikomsakis, K. N. and Mitrentsis, G. (2017). A computa-
tionally efficient simulation model for estimating en-
ergy consumption of electric vehicles in the context of
route planning applications. Transportation Research
Part D: Transport and Environment, 50:98–118.
Gerossier, A., Girard, R., and Kariniotakis, G. (2019).
Modeling and forecasting electric vehicle consump-
tion profiles. Energies, 12(7).
Hammerschmitt, B. K., Unsihuay-Vila, C., Sausen, J. P.,
Capeletti, M. B., Aoki, A. R., Teixeira, M. D., Bar-
riquello, C. H., and Abaide, A. d. R. (2024). Adaptive
charging simulation model for different electric vehi-
cles and mobility patterns. Energies, 17(16).
Hensher, D. A. (2018). Tackling road congestion – what
might it look like in the future under a collaborative
and connected mobility model? Transport Policy,
66:A1–A8.
Herrmann, S., Schulte, F., and Voß, S. (2014). Increas-
ing acceptance of free-floating car sharing systems us-
ing smart relocation strategies: A survey based study
of car2go hamburg. In Gonz
´
alez-Ram
´
ırez, R. G.,
Schulte, F., Voß, S., and Ceroni D
´
ıaz, J. A., edi-
tors, Computational Logistics, pages 151–162, Cham.
Springer International Publishing.
Liao, F. and Correia, G. (2021). Electric carsharing and
micromobility: A literature review on their usage pat-
tern, demand, and potential impacts. International
Journal of Sustainable Transportation, 16(3):269–
286.
Moss, M. L. (2012). Urban mobility in the 21st century.
Technical report, NYU Rudin Center for Transporta-
tion Policy.
Nicholas, M. and Bernard, M. R. (2021). Success factors
for electric carsharing. Technical report, INTERNA-
TIONAL COUNCIL ON CLEAN TRANSPORTA-
TION.
Perboli, G., Ferrero, F., Musso, S., and Vesco, A. (2018).
Business models and tariff simulation in car-sharing
services. Transportation Research Part A: Policy and
Practice, 115:32–48.
Rauf, H., Zehra Zaidi, S. S., Naveed, H., Mehmood, D.,
Jabeen, F., and Malik, A. W. (2023). Urbanevsim:
Open-source electric vehicle mobility and charging
simulation platform. In 2023 International Confer-
ence on Frontiers of Information Technology (FIT),
pages 79–84.
Weibin, Z., Yuhang, S., Yong, Q., Qianmu, L., and Minglei,
S. (2018). Traveler behavior analysis based on car2go
sharing operation data. In nternational Conference
on Transportation and Development 2018: Planning,
Sustainability, and Infrastructure Systems.
VEHITS 2025 - 11th International Conference on Vehicle Technology and Intelligent Transport Systems
542