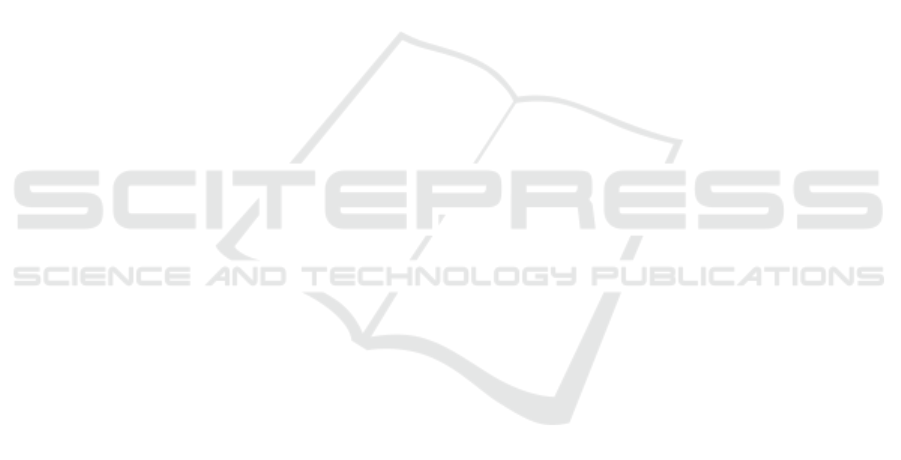
isting system family.
This work can be extended in several directions.
First, TCA provides additional kinds of implications,
not considered in this study, from which other types
of logical dependencies (e.g. mutual exclusions) can
be inferred. They can be used to fine-tune the soft-
ware’s final configuration. To address higher dimen-
sions, like the purpose or the version, Polyadic Con-
cept Analysis (Voutsadakis, 2002) can be used. Sec-
ond, the process can be refined to better match design-
ers’ needs. For instance, LLM can propose various
abstraction level options, or the implications provided
by the rule engine can be used without requiring LLM
to apply them. This may reduce the sensitivity of the
configuration to the randomness of LLM.
ACKNOWLEDGEMENTS
This work was supported by the ANR SmartFCA
project, Grant ANR-21-CE23-0023 of the French Na-
tional Research Agency.
REFERENCES
Acher, M. and Martinez, J. (2023). Generative AI for
reengineering variants into software product lines: An
experience report. In Proc. of the 27th ACM Int. Sys-
tems and Software Product Line Conf. - Volume B,
SPLC 2023, pages 57–66. ACM.
Arulmohan, S., Meurs, M., and Mosser, S. (2023). Extract-
ing domain models from textual requirements in the
era of large language models. In 5th Ws. on Artificial
Intelligence and Model-driven Eng. @ ACM/IEEE
MODELS 2023, pages 580–587. IEEE.
Bazin, A., Georges, T., Huchard, M., Martin, P., and Tiber-
macine, C. (2024). Exploring the 3-dimensional vari-
ability of websites’ user-stories using triadic concept
analysis. Int. J. Approx. Reason., 173:109248.
Bragilovski, M., van Can, A. T., Dalpiaz, F., and Sturm,
A. (2024). Deriving domain models from user stories:
Human vs. machines. In 32nd IEEE Int. Requirements
Engineering Conf., RE 2024, pages 31–42. IEEE.
Dalpiaz, F. (2018). Requirements data sets (user stories).
Mendeley Data, V1, doi: 10.17632/7zbk8zsd8y.1.
Dalpiaz, F., Schalk, I. V. D., Brinkkemper, S., Aydemir,
F. B., and Lucassen, G. (2019). Detecting terminolog-
ical ambiguity in user stories: Tool and experimenta-
tion. Inf. Softw. Technol., 110:3–16.
Ganter, B. and Obiedkov, S. A. (2004). Implications in
triadic formal contexts. In Conceptual Structures at
Work: 12th ICCS 2004, volume 3127 of LNCS, pages
186–195. Springer.
Ganter, B. and Wille, R. (1999). Formal Concept Analysis -
Mathematical Foundations. Springer.
Gomez-Vazquez, M. and Cabot, J. (2024). Exploring the
use of software product lines for the combination of
machine learning models. In Proc. of the 28th ACM
Int. Systems and Software Product Line Conference,
SPLC ’24, page 26–29. ACM.
Hou, X., Zhao, Y., Liu, Y., Yang, Z., Wang, K., Li, L.,
Luo, X., Lo, D., Grundy, J., and Wang, H. (2024).
Large language models for software engineering: A
systematic literature review. ACM Trans. Softw. Eng.
Methodol., 33(8).
Jahan, M., Hassan, M. M., Golpayegani, R., Ranjbaran,
G., Roy, C., Roy, B., and Schneider, K. (2024).
Automated Derivation of UML Sequence Diagrams
from User Stories: Unleashing the Power of Genera-
tive AI vs. a Rule-Based Approach. In Proc. of the
ACM/IEEE 27th Int. Conf. on Model Driven Engi-
neering Languages and Systems, MODELS ’24, page
138–148. ACM.
Lehmann, F. and Wille, R. (1995). A triadic approach to
formal concept analysis. In 3rd Int. Conf. on Concep-
tual Structures, ICCS ’95, volume 954 of LNCS, pages
32–43. Springer.
Lucassen, G., Dalpiaz, F., Van der Werf, J. M., and
Brinkkemper, S. (2016). Improving agile require-
ments: the quality user story framework and tool. Re-
quirements Engineering, 21.
Mandal, S., Chethan, A., Janfaza, V., Mahmud, S. M. F.,
Anderson, T. A., Turek, J., Tithi, J. J., and Muza-
hid, A. (2023). Large language models based au-
tomatic synthesis of software specifications. CoRR,
abs/2304.09181.
Mondal, S., Bappon, S. D., and Roy, C. K. (2024). En-
hancing user interaction in chatgpt: Characterizing
and consolidating multiple prompts for issue resolu-
tion. In 21st IEEE/ACM Int. Conf. on Mining Software
Repositories, pages 222–226. ACM.
Pohl, K., B
¨
ockle, G., and van der Linden, F. (2005). Soft-
ware Product Line Engineering - Foundations, Prin-
ciples, and Techniques. Springer.
Ronanki, K., Cabrero-Daniel, B., and Berger, C. (2024).
Chatgpt as a tool for user story quality evaluation:
Trustworthy out of the box? In Agile Processes in
Software Engineering and Extreme Programming –
Workshops, pages 173–181, Cham. Springer Nature
Switzerland.
Schulhoff, S., Ilie, M., Balepur, N., Kahadze, K., Liu,
A., Si, C., Li, Y., Gupta, A., Han, H., Schul-
hoff, S., Dulepet, P. S., Vidyadhara, S., Ki, D.,
Agrawal, S., Pham, C., Kroiz, G., Li, F., Tao, H.,
Srivastava, A., Costa, H. D., Gupta, S., Rogers,
M. L., Goncearenco, I., Sarli, G., Galynker, I.,
Peskoff, D., Carpuat, M., White, J., Anadkat, S.,
Hoyle, A., and Resnik, P. (2024). The prompt re-
port: A systematic survey of prompting techniques.
https://arxiv.org/abs/2406.06608.
Voutsadakis, G. (2002). Polyadic concept analysis. Order,
19(3):295–304.
White, J., Fu, Q., Hays, S., Sandborn, M., Olea, C., Gilbert,
H., Elnashar, A., Spencer-Smith, J., and Schmidt,
D. C. (2023). A Prompt Pattern Catalog to En-
hance Prompt Engineering with ChatGPT. CoRR,
abs/2302.11382.
Variability-Driven User-Story Generation Using LLM and Triadic Concept Analysis
625