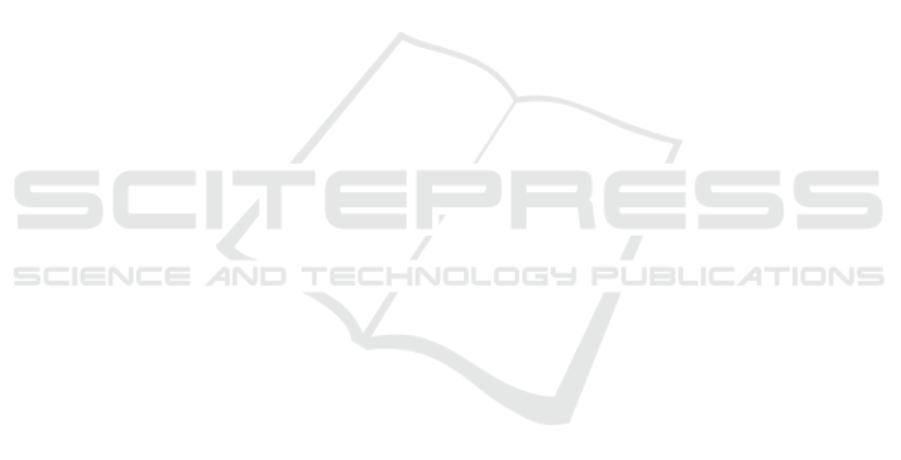
Several important takeaways from the field test are
noted. These include the importance of presenting the
results generated by the automation solution, which
should be done without overwhelming the stakehold-
ers. We also note the complexity of the initial formal-
ization of use-case-specific requirements, such that
these requirements could be supplied to the automa-
tion system. Additionally we note the particular com-
plexity of estimating and accounting for the costs that
are specific to the specific companies (e.g., cloud dis-
count contracts, running costs of the private data cen-
ter placements). This complexity can be detrimental
to the validity of the final results. Finally, we note
the importance of the appropriate visualization used
in the final results presentations.
REFERENCES
Aloysius, G. C., Bhatia, A., and Tiwari, K. (2023). Stabil-
ity and Availability Optimization of Distributed ERP
Systems During Cloud Migration. Lecture Notes in
Networks and Systems, 654 LNNS:343–354.
Altmann, J. and Kashef, M. M. (2014). Cost model based
service placement in federated hybrid clouds. Future
Generation Computer Systems, 41:79–90.
Badger, M., Grance, T., Patt-Corner, R., and Voas, J. (2012).
Cloud Computing Synopsis and Recommendations.
Technical Report NIST Special Publication (SP) 800-
146, National Institute of Standards and Technology.
Berhorst, R., D
¨
urk, M.-O., Hauzeneder, C., Sperzel, S.,
Wagner, P., and Zimmermann, R. (2021). DSAG-
Leitfaden: Hybrid Operations. Technical report,
Deutschsprachige SAP Anwendergruppe e.V.
B
¨
ogelsack, A., Chakraborty, U., Kumar, D., Rank, J., Tis-
chbierek, J., and Wolz, E. (2022). SAP S/4HANA
on Microsoft Azure – Concepts and Architecture. In
B
¨
ogelsack, A., Chakraborty, U., Kumar, D., Rank, J.,
Tischbierek, J., and Wolz, E., editors, SAP S/4HANA
Systems in Hyperscaler Clouds: Deploying SAP
S/4HANA in AWS, Google Cloud, and Azure, pages
267–323. Apress, Berkeley, CA.
Brogi, A., Corradini, A., and Soldani, J. (2019). Estimat-
ing costs of multi-component enterprise applications.
Formal Aspects of Computing, 31(4):421–451.
Deb, M. and Choudhury, A. (2021). Hybrid Cloud: A New
Paradigm in Cloud Computing. In Machine Learning
Techniques and Analytics for Cloud Security, chap-
ter 1, pages 1–23. John Wiley & Sons, Ltd.
Dimara, E., Zhang, H., Tory, M., and Franconeri, S. (2022).
The Unmet Data Visualization Needs of Decision
Makers Within Organizations. IEEE Transactions on
Visualization and Computer Graphics, 28(12):4101–
4112.
Greenberg, A., Hamilton, J., Maltz, D. A., and Patel, P.
(2009). The cost of a cloud: Research problems in
data center networks. SIGCOMM Comput. Commun.
Rev., 39(1):68–73.
Jung, G., Mukherjee, T., Kunde, S., Kim, H., Sharma,
N., and Goetz, F. (2013). CloudAdvisor: A
recommendation-as-a-service platform for cloud con-
figuration and pricing. Proceedings - 2013 IEEE 9th
World Congress on Services, SERVICES 2013, pages
456–463.
Kashef, M. M. and Altmann, J. (2012). A Cost Model for
Hybrid Clouds. In Vanmechelen, K., Altmann, J., and
Rana, O. F., editors, Economics of Grids, Clouds, Sys-
tems, and Services, pages 46–60, Berlin, Heidelberg.
Springer.
Kharitonov, A., Nahhas, A., M
¨
uller, H., and Turowski, K.
(2023). Data driven meta-heuristic-assisted approach
for placement of standard IT enterprise systems in
hybrid-cloud. In Proceedings of the 13th International
Conference on Cloud Computing and Services Science
- CLOSER, pages 139–146. SciTePress / INSTICC.
Marquard, U. and G
¨
otz, C. (2008). SAP Standard Appli-
cation Benchmarks - IT Benchmarks with a Business
Focus. In Kounev, S., Gorton, I., and Sachs, K., ed-
itors, Performance Evaluation: Metrics, Models and
Benchmarks, pages 4–8, Berlin, Heidelberg. Springer.
Menzel, M., Sch
¨
onherr, M., and Tai, S. (2013). (MC2)2:
Criteria, requirements and a software prototype for
Cloud infrastructure decisions. Software - Practice
and Experience, 43(11):1283–1297.
Mettouris, C., Vanezi, E., Zampas, G., Argyrou, S.,
Achilleos, A., Constantinides, A., and Papadopoulos,
G. A. (2022). CloudRecoMan: Cloud adoption made
easy. ACM International Conference Proceeding Se-
ries, pages 110–117.
OMG (2010). Business process model and notation
(BPMN) version 2.0. Standard, Object Management
Group (OMG).
Sahu, P., Roy, S., and Gharote, M. (2024). CloudAdvisor
for Sustainable and Data Residency Compliant Data
Placement in Multi-Cloud. In 2024 16th International
Conference on COMmunication Systems & NETworkS
(COMSNETS), pages 285–287.
Salapura, V. and Mahindru, R. (2016). Enabling enterprise-
level workloads in the enterprise-class cloud. IBM
Journal of Research and Development, 60(2-3):3:1–
3:8.
Subrahmanyam, R. S. (2022). An Overview of SAP
S/4HANA, pages 1–23. Apress, Berkeley, CA.
Venkatraman, A. and Arend, C. (2022). A Resilient, Ef-
ficient, and Adaptive Hybrid Cloud Fit for a Dy-
namic Digital Business. International Data Corpora-
tion (IDC). Report No.: IDC #EUR149741222.
Wu, C., Buyya, R., and Ramamohanarao, K. (2019).
Cloud Pricing Models: Taxonomy, Survey, and In-
terdisciplinary Challenges. ACM Comput. Surv.,
52(6):108:1–108:36.
Wu, J. Y. and Chen, L. T. (2020). Odoo erp with busi-
ness intelligence tool for a small-medium enterprise:
A scenario case study. In Proceedings of the 2020
11th International Conference on E-Education, E-
Business, E-Management, and E-Learning, IC4E ’20,
page 323–327, New York, NY, USA. Association for
Computing Machinery.
Towards IT Workload Hybrid-Cloud Placement Advisory in Enterprise
839