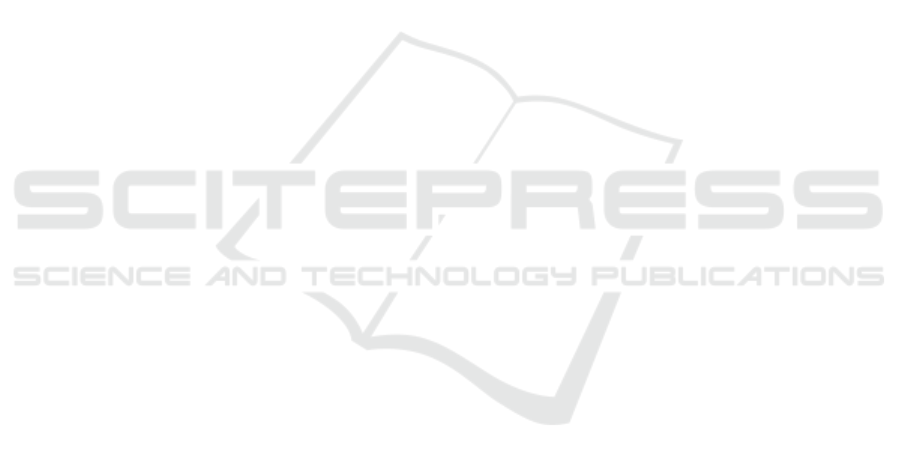
ACKNOWLEDGEMENTS
This work is supported by CESI EAST Region and
Ektidar, a Lebanese project aimed at empowering
youth.
REFERENCES
Agrawal, S., Sarkar, S., Aouedi, O., Yenduri, G., Piamrat,
K., Alazab, M., Bhattacharya, S., Maddikunta, P.
K. R., and Gadekallu, T. R. (2022). Federated learn-
ing for intrusion detection system: Concepts, chal-
lenges and future directions. Computer Communica-
tions, 195:346–361.
Al-Hawawreh, M., Sitnikova, E., and Aboutorab, N. (2022).
X-iiotid: A connectivity-agnostic and device-agnostic
intrusion data set for industrial internet of things.
IEEE Internet of Things Journal, 9(5):3962–3977.
Arbaoui, M., Brahmia, M.-E.-A., and Rahmoun, A. (2022).
Towards secure and reliable aggregation for federated
learning protocols in healthcare applications. In 2022
Ninth International Conference on Software Defined
Systems (SDS), pages 1–3.
Arbaoui, M., Brahmia, M.-e.-A., Rahmoun, A., and Zghal,
M. (2024). Federated learning survey: A multi-level
taxonomy of aggregation techniques, experimental in-
sights, and future frontiers. ACM Trans. Intell. Syst.
Technol., 15(6).
Bilot, T., Madhoun, N. E., Agha, K. A., and Zouaoui, A.
(2023). Graph neural networks for intrusion detection:
A survey. IEEE Access, 11:49114–49139.
Brahmia, M.-e.-A., Babouche, S., Ouchani, S., and Zghal,
M. (2022). An adaptive attack prediction framework
in cyber-physical systems. In 2022 Ninth Interna-
tional Conference on Software Defined Systems (SDS),
pages 1–7.
Chaudhary, A., Mittal, H., and Arora, A. (2019). Anomaly
detection using graph neural networks. In 2019 Inter-
national Conference on Machine Learning, Big Data,
Cloud and Parallel Computing (COMITCon), pages
346–350.
Chou, D. and Jiang, M. (2021). A survey on data-driven net-
work intrusion detection. ACM Comput. Surv., 54(9).
Ghalmane, Z., Cherifi, C., Cherifi, H., and Hassouni,
M. E. (2019a). Centrality in complex networks with
overlapping community structure. Scientific Reports,
9(1):10133.
Ghalmane, Z., Cherifi, C., Cherifi, H., and Hassouni, M. E.
(2020). Exploring hubs and overlapping nodes inter-
actions in modular complex networks. IEEE Access,
8:79650–79683.
Ghalmane, Z., Cherifi, C., Cherifi, H., and Hassouni,
M. E. (2021). Extracting modular-based backbones in
weighted networks. Information Sciences, 576:454–
474.
Ghalmane, Z., El Hassouni, M., Cherifi, C., and Cherifi, H.
(2018a). k-truss decomposition for modular centrality.
In 2018 9th International Symposium on Signal, Im-
age, Video and Communications (ISIVC), pages 241–
248.
Ghalmane, Z., Hassouni, M. E., and Cherifi, H. (2018b).
Betweenness centrality for networks with non-
overlapping community structure. In 2018 IEEE
Workshop on Complexity in Engineering (COM-
PENG), pages 1–5.
Ghalmane, Z., Hassouni, M. E., and Cherifi, H. (2019b).
Immunization of networks with non-overlapping com-
munity structure. Social Network Analysis and Min-
ing, 9(1):45.
Liu, Z., Thapa, N., Shaver, A., Roy, K., Siddula, M., Yuan,
X., and Yu, A. (2021). Using embedded feature selec-
tion and CNN for classification on CCD-INID-V1-A
new IoT dataset. Sensors (Basel), 21(14).
Lo, W. W., Layeghy, S., Sarhan, M., Gallagher, M., and
Portmann, M. (2022). E-graphsage: A graph neu-
ral network based intrusion detection system for iot.
In NOMS 2022-2022 IEEE/IFIP Network Operations
and Management Symposium, pages 1–9.
Park, S. B., Jo, H. J., and Lee, D. H. (2023). G-idcs: Graph-
based intrusion detection and classification system for
can protocol. IEEE Access, 11:39213–39227.
Protogerou, A., Papadopoulos, S., Drosou, A., Tzovaras,
D., and Refanidis, I. (2021). A graph neural net-
work method for distributed anomaly detection in iot.
Evolving Systems, 12(1):19–36.
Sarhan, M., Layeghy, S., Moustafa, N., and Portmann, M.
(2021). Netflow datasets for machine learning-based
network intrusion detection systems. In Deze, Z.,
Huang, H., Hou, R., Rho, S., and Chilamkurti, N., ed-
itors, Big Data Technologies and Applications, pages
117–135, Cham. Springer International Publishing.
Sowmya, T. and Mary Anita, E. (2023). A comprehensive
review of ai based intrusion detection system. Mea-
surement: Sensors, 28:100827.
Termos, M., Ghalmane, Z., Brahmia, M.-E.-A., Fadlallah,
A., Jaber, A., and Zghal, M. (2023). Intrusion detec-
tion system for iot based on complex networks and
machine learning. In 2023 IEEE Intl Conf on De-
pendable, Autonomic and Secure Computing (DASC),
pages 0471–0477.
Termos, M., Ghalmane, Z., el Amine Brahmia, M., Fad-
lallah, A., Jaber, A., and Zghal, M. (2024). Gdlc: A
new graph deep learning framework based on central-
ity measures for intrusion detection in iot networks.
Internet of Things, 26:101214.
Wu, Z., Pan, S., Chen, F., Long, G., Zhang, C., and Yu,
P. S. (2021). A comprehensive survey on graph neural
networks. IEEE Transactions on Neural Networks and
Learning Systems, 32(1):4–24.
Zhang, B., Li, J., Chen, C., Lee, K., and Lee, I. (2022). A
practical botnet traffic detection system using gnn. In
Meng, W. and Conti, M., editors, Cyberspace Safety
and Security, pages 66–78, Cham. Springer Interna-
tional Publishing.
Zhong, M., Lin, M., Zhang, C., and Xu, Z. (2024). A sur-
vey on graph neural networks for intrusion detection
systems: Methods, trends and challenges. Computers
& Security, 141:103821.
IoTBDS 2025 - 10th International Conference on Internet of Things, Big Data and Security
336