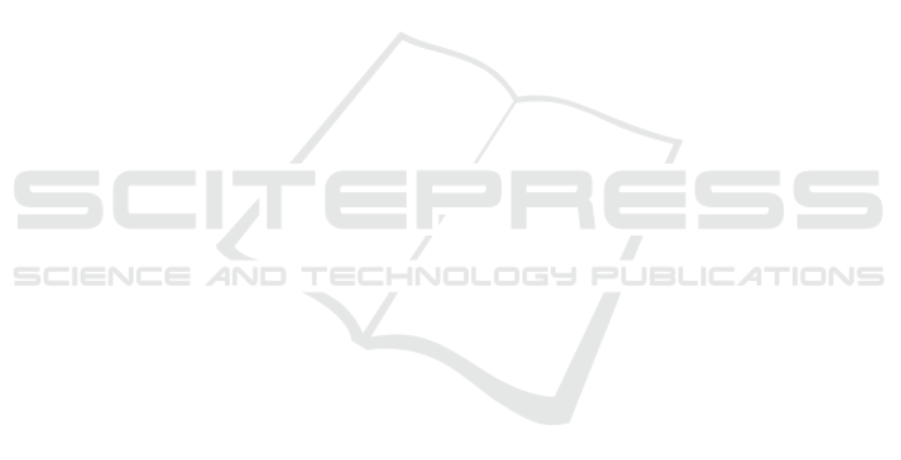
Potanin, M., Holtkemper, M., and Beecks, C. (2024). Ex-
ploring the integration of data science competencies
in modern automation frameworks: Insights for work-
force empowerment. In Intelligent Systems Confer-
ence, pages 232–240. Springer.
Ramamurthy, B. (2016). A practical and sustainable model
for learning and teaching data science. In Alphonce,
C., Tims, J., Caspersen, M., and Edwards, S., ed-
itors, Proceedings of the 47th ACM Technical Sym-
posium on Computing Science Education, pages 169–
174, New York, NY, USA. ACM.
Rosenthal, S. and Chung, T. (2020). A data science ma-
jor. In Zhang, J., Sherriff, M., Heckman, S., Cutter,
P., and Monge, A., editors, Proceedings of the 51st
ACM Technical Symposium on Computer Science Ed-
ucation, pages 178–184, New York, NY, USA. ACM.
Rowe, C. J. (1995). Clarifying the use of competence and
competency models in recruitment, assessment and
staff development. Industrial and Commercial Train-
ing, 27:12–17.
Russ-Eft, D. (1995). Defining competencies: a critique. Hu-
man Resource Development Quarterly, 6(4):329–335.
Salloum, M., Jeske, D., Ma, W., Papalexakis, V., Shelton,
C., Tsotras, V., and Zhou, S. (2021). Developing an in-
terdisciplinary data science program. In Sherriff, M.,
Merkle, L. D., Cutter, P., Monge, A., and Sheard, J.,
editors, Proceedings of the 52nd ACM Technical Sym-
posium on Computer Science Education, pages 509–
515, New York, NY, USA. ACM.
Schanzer, E., Pfenning, N., Denny, F., Dooman, S., Politz,
J. G., Lerner, B. S., Fisler, K., and Krishnamurthi, S.
(2022). Integrated data science for secondary schools.
In Merkle, L., Doyle, M., Sheard, J., Soh, L.-K.,
and Dorn, B., editors, Proceedings of the 53rd ACM
Technical Symposium on Computer Science Educa-
tion, pages 22–28, New York, NY, USA. ACM.
Schmitt, K. R. B., Clark, L., Kinnaird, K. M., Wertz, R.
E. H., and Sandstede, B. (2023). Evaluation of edi-
son’s data science competency framework through a
comparative literature analysis. Foundations of Data
Science, 5(2):177–198.
Shao, G., Quintana, J. P., Zakharov, W., Purzer, S., and Kim,
E. (2021). Exploring potential roles of academic li-
braries in undergraduate data science education cur-
riculum development. The Journal of Academic Li-
brarianship, 47(2):102320.
Shippmann, J., Ash, R., Batitsta, M., L., C., L.D, E., J., K.,
E.P., P., and J.I., S. (2000). The practice of compe-
tency modeling. Personnel Psychology, 53:703–740.
Stuart, R., Thompson, J. E., and Harrison, J. (1995). Trans-
lation: From generalizable to organization-specific
competence frameworks. Journal of Management De-
velopment, 14(1):67–80.
Suryan, K. and Gupta, R. (2021). Investigating academia-
industry gap for data science jobs and curriculum.
In 2021 International Conference on Data Analytics
for Business and Industry (ICDABI), pages 453–458.
IEEE.
The Joint Task Force on Computing Curricula (2013). Com-
puter science curricula 2013 - curriculum guidelines
for undergraduate degree programs in computer sci-
ence.
Thistlethwaite, J. E., Forman, D., Matthews, L. R., Rogers,
G. D., Steketee, C., and Yassine, T. (2014). Com-
petencies and frameworks in interprofessional educa-
tion: a comparative analysis. Academic Medicine,
89(6):869–875.
UN Global Working Group. Competency framework for big
data acquisition and processing.
Urs, S. R. and Minhaj, M. (2023). Evolution of data science
and its education in ischools: An impressionistic study
using curriculum analysis. Journal of the Association
for Information Science and Technology.
Vaast, E. and Pinsonneault, A. (2021). When digital tech-
nologies enable and threaten occupational identity:
The delicate balancing act of data scientists. MIS
Quarterly, 45(3):1087–1112.
Veaux, R. D., Agarwal, M., Averett, M., Baumer, B.,
Bray, A., Bressoud, T., Bryant, L., Cheng, L., Fran-
cis, A., Gould, R., Kim, A. Y., Kretchmar, M., Lu,
Q., Moskol, A., Nolan, D., Pelayo, R., Raleigh, S.,
Sethi, R. J., Sondjaja, M., Tiruviluamala, N., Uhlig,
P., Washington, T., Wesley, C., White, D., and Ye, P.
(2017). Curriculum guidelines for undergraduate pro-
grams in data science. Annual Review of Statistics and
Its Application, 4(1):15–30.
vom Brocke, J., Simons, A., Niehaves, B., Reimer, K., Plat-
tfaut, R., and Cleven, A., editors (2009). Reconstruct-
ing the giant: On the importance of rigour in docu-
menting the literature, volume 161.
Webster, J. and Watson, R. (2002). Analyzing the past to
prepare for the future: Writing a literature review. MIS
Quarterly, 26(2).
Wiktorski, T., Demchenko, Y., and Belloum, A. (2017).
Model curricula for data science edison data science
framework. In 2017 IEEE International Conference
on Cloud Computing Technology and Science (Cloud-
Com), pages 369–374. IEEE.
Wiktorski, T., Demchenko, Y., and Chertov, O. (2019).
Data science model curriculum implementation for
various types of big data infrastructure courses. In
2019 15th International Conference on eScience
(eScience), pages 541–547. IEEE.
Wu, H. (2017). Systematic study of big data science and
analytics programs. In 2017 ASEE Annual Conference
& Exposition.
Zhou, T., Jiang, D., Wang, F., Li, X., and Zheng, L. (2020).
A cdio oriented curriculum for division of data sci-
ence and big data technologies: The content, process
of derivation, and levels of proficiency. In 2020 8th
International Conference on Digital Home (ICDH),
pages 172–177. IEEE.
Zschech, P., Horn, R., Hoeschele, D., C., J., and K., H.
(2020). Intelligent user assistance for automated data
mining method selection. Business & Information
Systems Engineering, 62:227–247.
Towards a Standardized Data Science Competence Framework: A Literature Review Approach
581