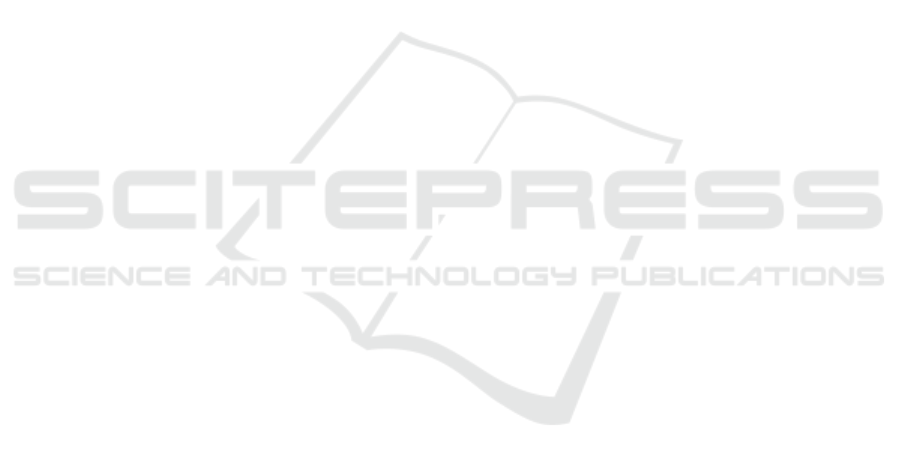
Long-term studies are required to assess the plat-
form’s impact on feedback management and product
development through qualitative user feedback analy-
sis and usability testing.
In conclusion, this work addresses the need for au-
tomated, scalable tools to interpret social media feed-
back, enhancing the RE process and helping stake-
holders meet evolving user expectations. Its contri-
butions to integrating feedback workflows into issue
management tools and expanding feedback sources
highlight its relevance in dynamic software develop-
ment environments.
REFERENCES
Ali, N. and Hong, J. E. (2019). A bird’s eye view on
social network sites and requirements engineering.
In ICSOFT 2019 - Proceedings of the 14th Interna-
tional Conference on Software Technologies, pages
347–354.
Bangor, A., Kortum, P. T., and Miller, J. T. (2009). De-
termining what individual sus scores mean: adding
an adjective rating scale. Journal of Usability Stud-
ies archive, 4:114–123.
Brooke, J. B. (1996). Sus: A ’quick and dirty’ usability
scale.
Di Sorbo, A., Panichella, S., Alexandru, C. V., Visaggio,
C. A., and Canfora, G. (2017). Surf: Summarizer
of user reviews feedback. In Proceedings - 2017
IEEE/ACM 39th International Conference on Soft-
ware Engineering Companion, ICSE-C 2017, pages
55–58.
Ebrahimi, A. M. and Barforoush, A. A. (2019). Prepro-
cessing role in analyzing tweets towards requirement
engineering. In ICEE 2019 - 27th Iranian Conference
on Electrical Engineering, pages 1905–1911.
Groen, E. C., Seyff, N., Ali, R., Dalpiaz, F., Doerr, J., Guz-
man, E., Hosseini, M., Marco, J., Oriol, M., Perini, A.,
and Stade, M. (2017). The crowd in requirements en-
gineering: The landscape and challenges. IEEE Soft-
ware, 34(2):44–52.
Guzman, E., Alkadhi, R., and Seyff, N. (2017). An ex-
ploratory study of twitter messages about software
applications. Requirements Engineering, 22(3):387–
412.
Iqbal, T., Khan, M., Taveter, K., and Seyff, N. (2021). Min-
ing reddit as a new source for software requirements.
In Proceedings of the IEEE International Conference
on Requirements Engineering, pages 128–138.
Kanchev, G. M. and Chopra, A. K. (2015). Social media
through the requirements lens: A case study of google
maps. In 1st International Workshop on Crowd-Based
Requirements Engineering, CrowdRE 2015 - Proceed-
ings, pages 7–12.
Li, C., Huang, L., Ge, J., Luo, B., and Ng, V. (2018). Au-
tomatically classifying user requests in crowdsourc-
ing requirements engineering. Journal of Systems and
Software, 138:108–123.
Maalej, W., Kurtanovi
´
c, Z., Nabil, H., and Stanik, C.
(2016a). On the automatic classification of app re-
views. Requirements Engineering, 21(3):311–331.
Maalej, W., Nayebi, M., Johann, T., and Ruhe, G. (2016b).
Toward data-driven requirements engineering. IEEE
Software, 33(1):48–54.
McIlroy, S., Ali, N., Khalid, H., and E. Hassan, A. (2016).
Analyzing and automatically labelling the types of
user issues that are raised in mobile app reviews. Em-
pirical Software Engineering, 21(3):1067–1106.
Nayebi, M., Cho, H., and Ruhe, G. (2018). App store min-
ing is not enough for app improvement. Empirical
Software Engineering.
Oehri, E. and Guzman, E. (2020). Same same but dif-
ferent: Finding similar user feedback across multiple
platforms and languages. In Proceedings of the IEEE
International Conference on Requirements Engineer-
ing, volume 2020-August, pages 44–54.
Panichella, S., Di Sorbo, A., Guzman, E., Visaggio, C. A.,
Canfora, G., and Gall, H. (2016). Ardoc: App re-
views development oriented classifier. In Proceedings
of the ACM SIGSOFT Symposium on the Foundations
of Software Engineering, volume 13-18-November-
2016, pages 1023–1027.
Scanlan, J., de Salas, K., Lim, D., and Roehrer, E. (2022).
Using social media to support requirements gathering
when users are not available. In Proceedings of the
Annual Hawaii International Conference on System
Sciences, pages 4227–4236.
Silva, M. (2024). Automated user feedback mining for
software requirements elicitation - a case study in the
streaming industry. Master’s thesis, Faculty of Engi-
neering of University of Porto. Available at: https:
//repositorio-aberto.up.pt/handle/10216/161054.
Stanik, C. and Maalej, W. (2019). Requirements intelli-
gence with openreq analytics. In Proceedings of the
IEEE International Conference on Requirements En-
gineering, volume 2019-September, pages 482–483.
Tizard, J., Wang, H., Yohannes, L., and Blincoe, K. (2019).
Can a conversation paint a picture? mining require-
ments in software forums.
Villarroel, L., Bavota, G., Russo, B., Oliveto, R., and
Di Penta, M. (2016). Release planning of mobile apps
based on user reviews. In Proceedings - International
Conference on Software Engineering, volume 14-22-
May-2016, pages 14–24.
Williams, G. and Mahmoud, A. (2017). Mining twitter
feeds for software user requirements. In Proceedings
- 2017 IEEE 25th International Requirements Engi-
neering Conference, RE 2017, pages 1–10.
ENASE 2025 - 20th International Conference on Evaluation of Novel Approaches to Software Engineering
160