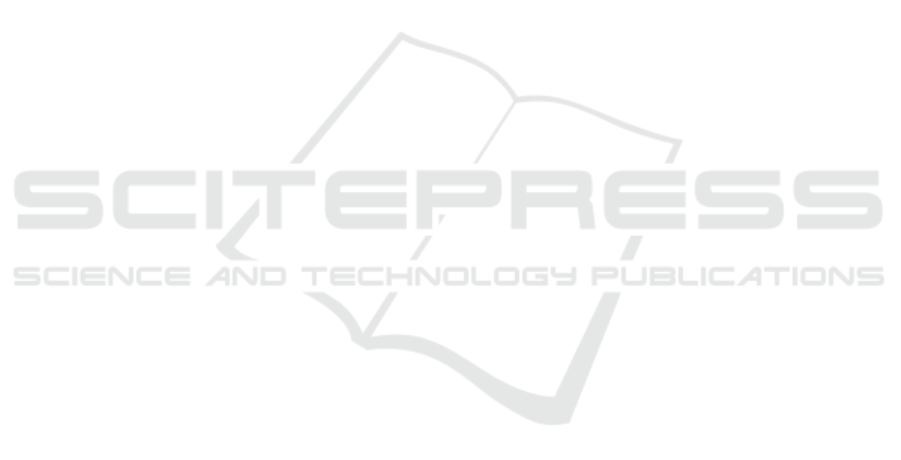
inant type of tremor is the rest tremor. This method
to estimate the rest tremor scores had promising re-
sults and has a low computational cost. After being
further explored and refined, this method has the po-
tential of being implemented for the clinical support
of rest tremor evaluation, helping monitor rest tremor
and the progression of the disease by providing data
for follow-up consultations. This method is limited by
the use of tremor scores given during the clinical eval-
uation in a controlled environment as labels for data
collected in free-living, which can lead to some data
being incorrectly labeled. Thus, the method should be
tested with other datasets to verify its results and with
sensor data collected during the clinical evaluations,
helping validate the method to be applied in a con-
trolled environment. Moreover, the results shown the
capability of this feature in the assessment of tremor
and its use with classical machine learning or deep
learning models for this task should be further ex-
plored.
REFERENCES
Adams, J. L., Dinesh, K., Snyder, C. W., Xiong, M.,
Tarolli, C. G., Sharma, S., Dorsey, E. R., and Sharma,
G. (2021). A real-world study of wearable sen-
sors in parkinson’s disease. npj Parkinson’s Disease,
7(1):106.
Amato, F., Saggio, G., Cesarini, V., Olmo, G., and Costan-
tini, G. (2023). Machine learning-and statistical-based
voice analysis of parkinson’s disease patients: A sur-
vey. Expert Systems with Applications, 219:119651.
Bazgir, O., Habibi, S. A. H., Palma, L., Pierleoni, P., and
Nafees, S. (2018). A classification system for assess-
ment and home monitoring of tremor in patients with
parkinson’s disease. Journal of Medical Signals &
Sensors, 8(2):65–72.
Burq, M., Rainaldi, E., Ho, K. C., Chen, C., Bloem,
B. R., Evers, L. J. W., Helmich, R. C., Myers, L.,
Marks, W. J., and Kapur, R. (2022). Virtual exam
for parkinson’s disease enables frequent and reliable
remote measurements of motor function. npj Digital
Medicine, 5(1). Cited by: 6; All Open Access, Gold
Open Access, Green Open Access.
Channa, A., Ifrim, R.-C., Popescu, D., and Popescu, N.
(2021). A-wear bracelet for detection of hand tremor
and bradykinesia in parkinson’s patients. Sensors,
21(3):981.
de Oliveira Andrade, A., Paixao, A. P. S., Cabral, A. M.,
Rabelo, A. G., Luiz, L. M. D., Dion
´
ısio, V. C., Vieira,
M. F., Pereira, J. M., Rueda, A., Krishnan, S., et al.
(2020). Task-specific tremor quantification in a clini-
cal setting for parkinson’s disease. Journal of Medical
and Biological Engineering, 40(6):821–850.
Goetz, C. G., Tilley, B. C., Shaftman, S. R., Stebbins, G. T.,
Fahn, S., Martinez-Martin, P., Poewe, W., Sampaio,
C., Stern, M. B., Dodel, R., et al. (2008). Movement
disorder society-sponsored revision of the unified
parkinson’s disease rating scale (mds-updrs): scale
presentation and clinimetric testing results. Movement
disorders: official journal of the Movement Disorder
Society, 23(15):2129–2170.
Huo, W., Angeles, P., Tai, Y. F., Pavese, N., Wilson, S.,
Hu, M. T., and Vaidyanathan, R. (2020). A heteroge-
neous sensing suite for multisymptom quantification
of parkinson’s disease. IEEE Transactions on Neural
Systems and Rehabilitation Engineering, 28(6):1397–
1406.
Kuosmanen, E., Wolling, F., Vega, J., Kan, V., Nishiyama,
Y., Harper, S., Van Laerhoven, K., Hosio, S., Fer-
reira, D., et al. (2020). Smartphone-based monitor-
ing of parkinson disease: quasi-experimental study to
quantify hand tremor severity and medication effec-
tiveness. JMIR mHealth and uHealth, 8(11):e21543.
Pierleoni, P., Belli, A., Bazgir, O., Maurizi, L., Paniccia, M.,
and Palma, L. (2019). A smart inertial system for 24h
monitoring and classification of tremor and freezing
of gait in parkinson’s disease. IEEE Sensors Journal,
19(23):11612–11623.
San-Segundo, R., Zhang, A., Cebulla, A., Panev, S., Tabor,
G., Stebbins, K., Massa, R. E., Whitford, A., De la
Torre, F., and Hodgins, J. (2020). Parkinson’s dis-
ease tremor detection in the wild using wearable ac-
celerometers. Sensors, 20(20):5817.
Shawen, N., O’Brien, M. K., Venkatesan, S., Lonini, L.,
Simuni, T., Hamilton, J. L., Ghaffari, R., Rogers, J. A.,
and Jayaraman, A. (2020). Role of data measurement
characteristics in the accurate detection of parkinson’s
disease symptoms using wearable sensors. Journal of
neuroengineering and rehabilitation, 17(1):1–14.
Shcherbak, A., Kovalenko, E., and Somov, A. (2023). De-
tection and classification of early stages of parkinson’s
disease through wearable sensors and machine learn-
ing. IEEE Transactions on Instrumentation and Mea-
surement, 72:1–9.
Sigcha, L., Pav
´
on, I., Costa, N., Costa, S., Gago, M.,
Arezes, P., L
´
opez, J. M., and De Arcas, G. (2021). Au-
tomatic resting tremor assessment in parkinson’s dis-
ease using smartwatches and multitask convolutional
neural networks. Sensors, 21(1):291.
Smid, A., Elting, J. W. J., van Dijk, J. M. C., Otten, B.,
Oterdoom, D. M., Tamasi, K., Heida, T., van Laar, T.,
and Drost, G. (2022). Intraoperative quantification of
mds-updrs tremor measurements using 3d accelerom-
etry: A pilot study. Journal of Clinical Medicine,
11(9):2275.
Welch, P. (1967). The use of fast fourier transform for
the estimation of power spectra: a method based on
time averaging over short, modified periodograms.
IEEE Transactions on audio and electroacoustics,
15(2):70–73.
Zajki-Zechmeister, T., K
¨
ogl, M., Kalsberger, K., Fran-
thal, S., Homayoon, N., Katschnig-Winter, P., Wen-
zel, K., Zajki-Zechmeister, L., and Schwingenschuh,
P. (2020). Quantification of tremor severity with a mo-
bile tremor pen. Heliyon, 6(8):e04702.
Unified Parkinson’s Disease Rating Scale Rest Tremor Score Estimation Using the Fundamental Frequency
319