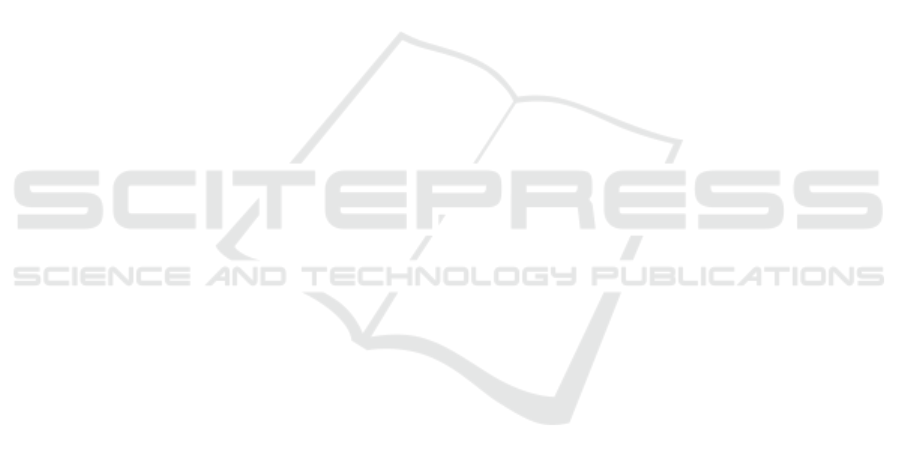
(neighborhood), the network topology and a set
of features that describe them. The method
outperformed AC.Rank
A
and CBA in terms of
interpretability while maintaining the performance of
the models. The best results were achieved with
[G2;G2+BL] configuration.
We believe that this study presents promising
results, which can be better explored in future works
regarding (i) the impact of hyperparameters on
the process (HyP-1, HyP-2, HyP-3, HyP-GCN),
(ii) the possibility of using GCNs in other steps
of the induction process, as well as in other
flows (as explored by (Dall’Agnol and Carvalho,
2024)), (iii) the construction of solutions that
incorporate black-box approaches to improve
inherently interpretable (white-box) solutions.
ACKNOWLEDGEMENTS
This study was financed in part by the Coordenac¸
˜
ao
de Aperfeic¸oamento de Pessoal de N
´
ıvel Superior
- Brasil (CAPES). The authors are also grateful to
the S
˜
ao Paulo Research Foundation - FAPESP (grant
#2024/04890-5) and the Brazilian National Council
for Scientific and Technological Development - CNPq
(grants #313193/2023-1 and #422667/2021-8) for
their financial support.
REFERENCES
Agrawal, R. and Srikant, R. (1994). Fast algorithms
for mining association rules in large databases. In
Proceedings of the 20th International Conference on
Very Large Data Bases (VLDB), pages 487––499.
Morgan Kaufmann Publishers Inc.
Bui-Thi, D., Meysman, P., and Laukens, K. (2022).
MoMAC: Multi-objective optimization to
combine multiple association rules into an
interpretable classification. Applied Intelligence,
52(3):3090––3102.
Dall’Agnol, M. and Carvalho, V. O. (2024). AC.RankA:
Rule ranking method via aggregation of objective
measures for associative classifiers. IEEE Access,
12:88862–88882.
Dall’Agnol, M. and De Carvalho, V. O. (2023a). Clustering
the behavior of objective measures in associative
classifiers. In 18th Iberian Conference on Information
Systems and Technologies (CISTI), pages 1–6.
Dall’Agnol, M. and De Carvalho, V. O. (2023b). Ranking
rules in associative classifiers via borda’s methods. In
18th Iberian Conference on Information Systems and
Technologies (CISTI), pages 1–6.
Demsar, J. (2006). Statistical comparisons of classifiers
over multiple data sets. J. Mach. Learn. Res., 7:1––30.
Ju, W., Fang, Z., Gu, Y., Liu, Z., Long, Q., Qiao, Z., Qin,
Y., Shen, J., Sun, F., Xiao, Z., Yang, J., Yuan, J.,
Zhao, Y., Wang, Y., Luo, X., and Zhang, M. (2024).
A comprehensive survey on deep graph representation
learning. Neural Networks, 173:106207.
Khemani, B., Patil, S., Kotecha, K., and Tanwar, S.
(2024). A review of graph neural networks: concepts,
architectures, techniques, challenges, datasets,
applications, and future directions. J Big Data,
11(18).
Kipf, T. N. and Welling, M. (2017). Semi-supervised
classification with graph convolutional networks.
In International Conference on Learning
Representations (ICLR).
Liu, B., Hsu, W., and Ma, Y. (1998). Integrating
classification and association rule mining. In In
Proceedings of the 4th International Conference on
Knowledge Discovery and Data Mining (KDD),,
pages 27–31.
Margot, V. and Luta, G. (2021). A new method to compare
the interpretability of rule-based algorithms. Ai,
2(4):621–635.
Rudin, C. (2019). Stop explaining black box machine
learning models for high stakes decisions and use
interpretable models instead.
Sharma, R., Kaushik, M., Peious, S. A., Yahia, S. B.,
and Draheim, D. (2020). Expected vs. Unexpected:
Selecting right measures of interestingness. In Big
Data Analytics and Knowledge Discovery - 22nd
International Conference (DaWaK), volume 12393,
pages 38–47.
Somyanonthanakul, R. and Theeramunkong, T. (2022).
Scenario-based analysis for discovering relations
among interestingness measures. Information
Sciences, 590:346–385.
Tan, P.-N., Steinbach, M., Karpatne, A., and Kumar, V.
(2019). Introduction to Data Mining. 2 edition.
Tew, C., Giraud-Carrier, C., Tanner, K., and Burton,
S. (2014). Behavior-based clustering and analysis
of interestingness measures for association rule
mining. Data Mining and Knowledge Discovery,
28(4):1004–1045.
Zhou, J., Cui, G., Hu, S., Zhang, Z., Yang, C., Liu, Z.,
Wang, L., Li, C., and Sun, M. (2020). Graph neural
networks: A review of methods and applications. AI
Open, 1:57–81.
Using Graph Convolutional Networks to Rank Rules in Associative Classifiers
325