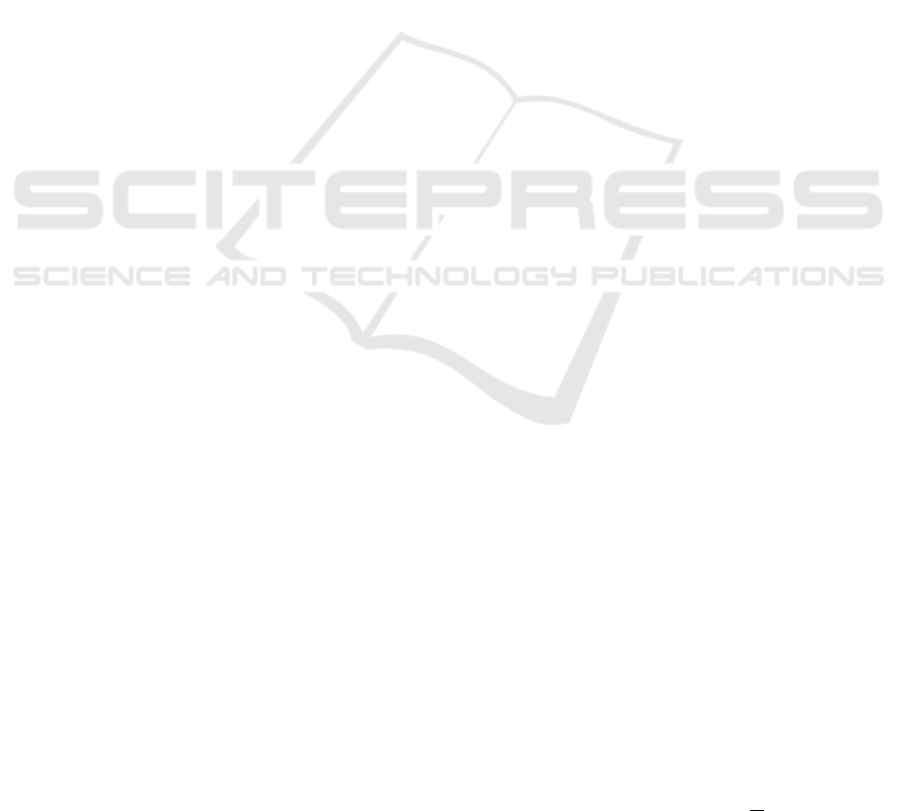
fully generated and refined Depth images from single
RGB inputs, achieving a notable accuracy improve-
ment from 82.78% to 90.19% over five iterations. The
iterative refinement process not only enhanced the ac-
curacy but also significantly reduced the percentage
of missing pixels in Depth images.
Additionally, leveraging the U-Net algorithm for
image segmentation allowed us to automate and ac-
celerate the correction process, further improving the
prediction accuracy to 96.44%. These advancements
were validated using the Cityscapes dataset, which
served as an effective benchmark for urban scene
understanding in autonomous driving applications.
Our methodology demonstrated robust performance
in filling missing information, as evidenced by sub-
stantial improvements in corrected pixel percentages
and accuracy metrics.
This work lays a strong foundation for future re-
search aimed at enhancing Depth image generation
and refinement techniques. The iterative training ap-
proach and segmentation-based corrections can be
extended to other datasets and use cases, such as
3D reconstruction, robotics, and other computer vi-
sion applications, where accurate depth information is
paramount. Future directions may include optimizing
the computational efficiency of the model and explor-
ing multi-modal input strategies to further improve
depth prediction performance in real-time scenarios.
REFERENCES
Agarwal, A. and Arora, C. (2023). Attention attention ev-
erywhere: Monocular depth prediction with skip at-
tention. In Proceedings of the IEEE/CVF Winter Con-
ference on Applications of Computer Vision, pages
5861–5870.
Chaar, M., Weidl, G., and Raiyn, J. (2023). Analyse the
effect of fog on the perception. EU Science Hub, page
329.
Chaar, M. M., Raiyn, J., and Weidl, G. (2024). Improving
the perception of objects under foggy conditions in the
surrounding environment. Research square.
Cordts, M., Omran, M., Ramos, S., Rehfeld, T., Enzweiler,
M., Benenson, R., Franke, U., Roth, S., and Schiele,
B. (2016). The cityscapes dataset for semantic urban
scene understanding. In Proceedings of the IEEE con-
ference on computer vision and pattern recognition,
pages 3213–3223.
Cordts, M., Omran, M., Ramos, S., Scharw
¨
achter, T., En-
zweiler, M., Benenson, R., Franke, U., Roth, S., and
Schiele, B. (2015). The cityscapes dataset. In CVPR
Workshop on The Future of Datasets in Vision.
Downloads, C. D. (2016). Cityscapes dataset downloads.
https://www.cityscapes-dataset.com/downloads/. Ac-
cessed: February 5, 2025.
Eigen, D., Puhrsch, C., and Fergus, R. (2014). Depth map
prediction from a single image using a multi-scale
deep network. Advances in neural information pro-
cessing systems, 27.
Kaehler, A. and Bradski, G. (2016). Learning OpenCV 3:
computer vision in C++ with the OpenCV library. ”
O’Reilly Media, Inc.”.
Ma, F., Cavalheiro, G. V., and Karaman, S. (2019). Self-
supervised sparse-to-dense: Self-supervised depth
completion from lidar and monocular camera. In 2019
International Conference on Robotics and Automation
(ICRA), pages 3288–3295. IEEE.
Minaee, S., Boykov, Y., Porikli, F., Plaza, A., Kehtarnavaz,
N., and Terzopoulos, D. (2021). Image segmenta-
tion using deep learning: A survey. IEEE transac-
tions on pattern analysis and machine intelligence,
44(7):3523–3542.
OpenCv (2024). Depth map from stereo images. Last ac-
cessed 18 November 2024.
Ranftl, R., Lasinger, K., Hafner, D., Schindler, K., and
Koltun, V. (2020). Towards robust monocular depth
estimation: Mixing datasets for zero-shot cross-
dataset transfer. IEEE transactions on pattern anal-
ysis and machine intelligence, 44(3):1623–1637.
Ronneberger, O., Fischer, P., and Brox, T. (2015). U-
net: Convolutional networks for biomedical image
segmentation. In Medical image computing and
computer-assisted intervention–MICCAI 2015: 18th
international conference, Munich, Germany, October
5-9, 2015, proceedings, part III 18, pages 234–241.
Springer.
Team, C. (2021). cityscapesscripts. Last accessed 17
November 2024.
Xian, K., Shen, C., Cao, Z., Lu, H., Xiao, Y., Li, R., and
Luo, Z. (2018). Monocular relative depth perception
with web stereo data supervision. In Proceedings of
the IEEE Conference on Computer Vision and Pattern
Recognition, pages 311–320.
Yu, Y., Wang, C., Fu, Q., Kou, R., Huang, F., Yang, B.,
Yang, T., and Gao, M. (2023). Techniques and chal-
lenges of image segmentation: A review. Electronics,
12(5):1199.
Zhang, A., Ma, Y., Liu, J., and Sun, J. (2023). Promot-
ing monocular depth estimation by multi-scale resid-
ual laplacian pyramid fusion. IEEE Signal Processing
Letters, 30:205–209.
Zhou, R. (2024). Scalable multi-view stereo camera array
for real-time image capture and 3d display in real-
world applications. Mathematical Modeling and Al-
gorithm Application, 2(2):43–48.
APPENDIX A
We calculate the average number of black pixels
across all Depth images in the Cityscapes dataset us-
ing the following formula:
Average black pixels =
1
N
N
∑
P∈P
Π
Predicting Depth Maps from Single RGB Images and Addressing Missing Information in Depth Estimation
555