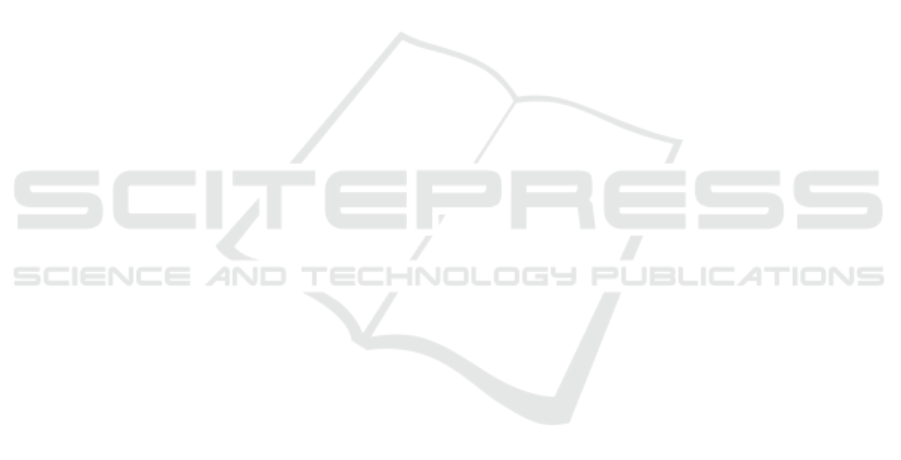
tion recognition capabilities to optimize interactions
and create a more adaptive and enriching educational
experience.
REFERENCES
Abdelghani, R., Wang, Y., Yuan, X., Wang, T., Sauz
´
eon,
H., and Oudeyer, P. (2022). Gpt-3-driven pedagogical
agents for training children’s curious question-asking
skills. arxiv. preprint arXiv, 2211.
Adeshola, I. and Adepoju, A. P. (2024). The opportunities
and challenges of chatgpt in education. Interactive
Learning Environments, 32(10):6159–6172.
Bangor, A., Kortum, P., and Miller, J. (2009). Determining
what individual sus scores mean: Adding an adjective
rating scale. Journal of usability studies, 4(3):114–
123.
Brooke, J. (1996). Sus: A quick and dirty usability scale.
Usability Evaluation in Industry.
Chan, T.-W. and Baskin, A. B. (1988). Studying with the
prince: The computer as a learning companion. In
Proceedings of the International Conference on Intel-
ligent Tutoring Systems, volume 194200.
Chiu, T. K. (2024). Future research recommendations
for transforming higher education with generative
ai. Computers and Education: Artificial Intelligence,
6:100197.
Chiu, T. K. and Hew, T. K. (2018). Factors influencing peer
learning and performance in mooc asynchronous on-
line discussion forum. Australasian Journal of Edu-
cational Technology, 34(4).
Gao, Y., Xiong, Y., Gao, X., Jia, K., Pan, J., Bi, Y., Dai, Y.,
Sun, J., and Wang, H. (2023). Retrieval-augmented
generation for large language models: A survey. arXiv
preprint arXiv:2312.10997.
Hone, K. S. and El Said, G. R. (2016). Exploring the factors
affecting mooc retention: A survey study. Computers
& Education, 98:157–168.
Ji, Z., Lee, N., Frieske, R., Yu, T., Su, D., Xu, Y., Ishii, E.,
Bang, Y. J., Madotto, A., and Fung, P. (2023). Survey
of hallucination in natural language generation. ACM
Computing Surveys, 55(12):1–38.
Ko, H.-T., Liu, Y.-K., Tsai, Y.-C., and Suen, S. (2024). En-
hancing python learning through retrieval-augmented
generation: A theoretical and applied innovation in
generative ai education. In International Conference
on Innovative Technologies and Learning, pages 164–
173. Springer.
Lewis, P., Perez, E., Piktus, A., Petroni, F., Karpukhin,
V., Goyal, N., K
¨
uttler, H., Lewis, M., Yih, W.-t.,
Rockt
¨
aschel, T., et al. (2020). Retrieval-augmented
generation for knowledge-intensive nlp tasks. Ad-
vances in Neural Information Processing Systems,
33:9459–9474.
Liu, R., Zenke, C., Liu, C., Holmes, A., Thornton, P., and
Malan, D. J. (2024). Teaching cs50 with ai: leveraging
generative artificial intelligence in computer science
education. In Proceedings of the 55th ACM Techni-
cal Symposium on Computer Science Education V. 1,
pages 750–756.
Mariani, M. M., Hashemi, N., and Wirtz, J. (2023). Arti-
ficial intelligence empowered conversational agents:
A systematic literature review and research agenda.
Journal of Business Research, 161:113838.
Miladi, F., Psych
´
e, V., and Lemire, D. (2024). Leverag-
ing gpt-4 for accuracy in education: A comparative
study on retrieval-augmented generation in moocs. In
International Conference on Artificial Intelligence in
Education, pages 427–434. Springer.
Neelakantan, A., Xu, T., Puri, R., Radford, A., Han,
J. M., Tworek, J., Yuan, Q., Tezak, N., Kim, J. W.,
Hallacy, C., et al. (2022). Text and code embed-
dings by contrastive pre-training. arXiv preprint
arXiv:2201.10005.
Psych
´
e, V. (2020). Clom-Motsia: MOOC sur l’intelligence
artificielle. https://clom-motsia.teluq.ca/, last ac-
cessed Jan 17 2024.
Shuster, K., Poff, S., Chen, M., Kiela, D., and Weston, J.
(2021). Retrieval augmentation reduces hallucination
in conversation. arXiv preprint arXiv:2104.07567.
Slade, J. J., Hyk, A., and Gurung, R. A. (2024). Trans-
forming learning: Assessing the efficacy of a retrieval-
augmented generation system as a tutor for introduc-
tory psychology. In Proceedings of the Human Fac-
tors and Ergonomics Society Annual Meeting, vol-
ume 68, pages 1827–1830. SAGE Publications Sage
CA: Los Angeles, CA.
Taneja, K., Maiti, P., Kakar, S., Guruprasad, P., Rao, S., and
Goel, A. K. (2024). Jill watson: A virtual teaching
assistant powered by chatgpt. In International Con-
ference on Artificial Intelligence in Education, pages
324–337. Springer.
Tullis, T. S. and Stetson, J. N. (2004). A comparison of
questionnaires for assessing website usability. In Us-
ability professional association conference, volume 1,
pages 1–12. Minneapolis, USA.
Vijaymeena, M. and Kavitha, K. (2016). A survey on simi-
larity measures in text mining. Machine Learning and
Applications: An International Journal, 3(2):19–28.
Wang, K., Ramos, J., and Lawrence, R. (2023). Chated:
a chatbot leveraging chatgpt for an enhanced learn-
ing experience in higher education. arXiv preprint
arXiv:2401.00052.
Xie, Z., Wu, X., and Xie, Y. (2024). Can interaction with
generative artificial intelligence enhance learning au-
tonomy? a longitudinal study from comparative per-
spectives of virtual companionship and knowledge ac-
quisition preferences. Journal of Computer Assisted
Learning.
Yan, L., Zhao, L., Echeverria, V., Jin, Y., Alfredo, R.,
Li, X., Ga
ˇ
sevi’c, D., and Martinez-Maldonado, R.
(2024). Vizchat: enhancing learning analytics dash-
boards with contextualised explanations using multi-
modal generative ai chatbots. In International Con-
ference on Artificial Intelligence in Education, pages
180–193. Springer.
Yin, S., Shang, Q., Wang, H., and Che, B. (2019). The
analysis and early warning of student loss in mooc
course. In Proceedings of the ACM Turing Celebra-
tion Conference-China, pages 1–6.
CSEDU 2025 - 17th International Conference on Computer Supported Education
354