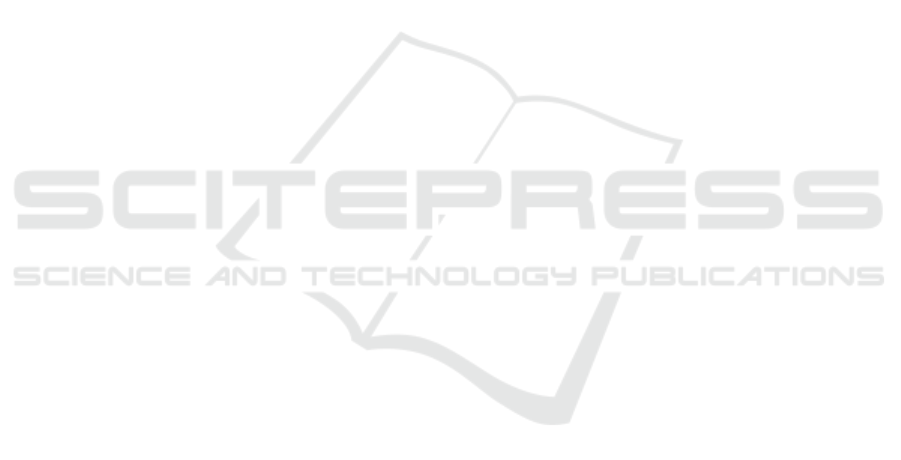
2018 26th ACM joint meeting on European software
engineering conference and symposium on the foun-
dations of software engineering, pages 60–70.
He, S., Zhu, J., He, P., and Lyu, M. R. (2016). Experi-
ence report: System log analysis for anomaly detec-
tion. In 2016 IEEE 27th international symposium on
software reliability engineering (ISSRE), pages 207–
218. IEEE.
Joulin, A. (2016). Fasttext. zip: Compressing text classifi-
cation models. arXiv preprint arXiv:1612.03651.
Le, V.-H. and Zhang, H. (2022). Log-based anomaly detec-
tion with deep learning: How far are we? In Proceed-
ings of the 44th international conference on software
engineering, pages 1356–1367.
Liang, Y., Zhang, Y., Xiong, H., and Sahoo, R. (2007). Fail-
ure prediction in ibm bluegene/l event logs. In Sev-
enth IEEE International Conference on Data Mining
(ICDM 2007), pages 583–588. IEEE.
Lin, Q., Zhang, H., Lou, J.-G., Zhang, Y., and Chen, X.
(2016). Log clustering based problem identification
for online service systems. In Proceedings of the
38th International Conference on Software Engineer-
ing Companion, pages 102–111.
Liu, F. T., Ting, K. M., and Zhou, Z.-H. (2008). Isolation
forest. In 2008 eighth ieee international conference
on data mining, pages 413–422. IEEE.
Lou, J.-G., Fu, Q., Yang, S., Xu, Y., and Li, J. (2010). Min-
ing invariants from console logs for system problem
detection. In 2010 USENIX Annual Technical Confer-
ence (USENIX ATC 10).
Lu, S., Wei, X., Li, Y., and Wang, L. (2018). Detect-
ing anomaly in big data system logs using convolu-
tional neural network. In 2018 IEEE 16th Intl Conf
on Dependable, Autonomic and Secure Computing,
16th Intl Conf on Pervasive Intelligence and Comput-
ing, 4th Intl Conf on Big Data Intelligence and Com-
puting and Cyber Science and Technology Congress
(DASC/PiCom/DataCom/CyberSciTech), pages 151–
158. IEEE.
Makanju, A. A., Zincir-Heywood, A. N., and Milios, E. E.
(2009). Clustering event logs using iterative partition-
ing. In Proceedings of the 15th ACM SIGKDD inter-
national conference on Knowledge discovery and data
mining, pages 1255–1264.
Meng, W., Liu, Y., Zhu, Y., Zhang, S., Pei, D., Liu, Y.,
Chen, Y., Zhang, R., Tao, S., Sun, P., et al. (2019).
Loganomaly: Unsupervised detection of sequential
and quantitative anomalies in unstructured logs. In
IJCAI, volume 19, pages 4739–4745.
Nandi, A., Mandal, A., Atreja, S., Dasgupta, G. B., and
Bhattacharya, S. (2016). Anomaly detection using
program control flow graph mining from execution
logs. In Proceedings of the 22nd ACM SIGKDD inter-
national conference on knowledge discovery and data
mining, pages 215–224.
Nedelkoski, S., Bogatinovski, J., Acker, A., Cardoso, J.,
and Kao, O. (2020). Self-attentive classification-based
anomaly detection in unstructured logs. In 2020 IEEE
International Conference on Data Mining (ICDM),
pages 1196–1201. IEEE.
Nedelkoski, S., Bogatinovski, J., Acker, A., Cardoso, J.,
and Kao, O. (2021). Self-supervised log parsing.
In Machine Learning and Knowledge Discovery in
Databases: Applied Data Science Track: European
Conference, ECML PKDD 2020, Ghent, Belgium,
September 14–18, 2020, Proceedings, Part IV, pages
122–138. Springer.
Shima, K. (2016). Length matters: Clustering system
log messages using length of words. arXiv preprint
arXiv:1611.03213.
Wang, J., Tang, Y., He, S., Zhao, C., Sharma, P. K., Alfarraj,
O., and Tolba, A. (2020). Logevent2vec: Logevent-to-
vector based anomaly detection for large-scale logs in
internet of things. Sensors, 20(9):2451.
Xia, B., Bai, Y., Yin, J., Li, Y., and Xu, J. (2021). Loggan: a
log-level generative adversarial network for anomaly
detection using permutation event modeling. Informa-
tion Systems Frontiers, 23:285–298.
Xu, W., Huang, L., Fox, A., Patterson, D., and Jordan,
M. I. (2009). Detecting large-scale system problems
by mining console logs. In Proceedings of the ACM
SIGOPS 22nd symposium on Operating systems prin-
ciples, pages 117–132.
Yang, L., Chen, J., Wang, Z., Wang, W., Jiang, J., Dong,
X., and Zhang, W. (2021). Semi-supervised log-based
anomaly detection via probabilistic label estimation.
In 2021 IEEE/ACM 43rd International Conference
on Software Engineering (ICSE), pages 1448–1460.
IEEE.
Zhang, X., Xu, Y., Lin, Q., Qiao, B., Zhang, H., Dang, Y.,
Xie, C., Yang, X., Cheng, Q., Li, Z., et al. (2019).
Robust log-based anomaly detection on unstable log
data. In Proceedings of the 2019 27th ACM joint meet-
ing on European software engineering conference and
symposium on the foundations of software engineer-
ing, pages 807–817.
Zhu, J., He, S., Liu, J., He, P., Xie, Q., Zheng, Z., and Lyu,
M. R. (2019). Tools and benchmarks for automated
log parsing. In 2019 IEEE/ACM 41st International
Conference on Software Engineering: Software En-
gineering in Practice (ICSE-SEIP), pages 121–130.
IEEE.
IoTBDS 2025 - 10th International Conference on Internet of Things, Big Data and Security
152