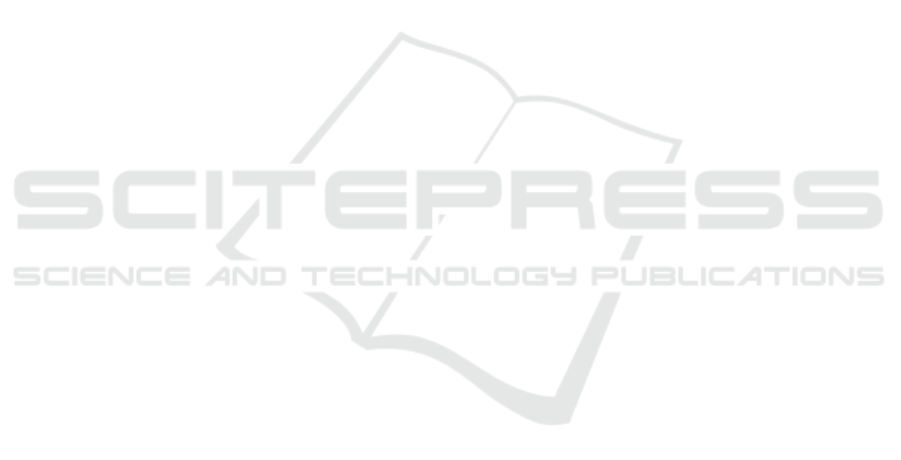
6 FUTURE WORK
In this paper, we proposed a predictive-based resource
allocation algorithm that combines machine learning
predictions with preference aggregation techniques to
optimize resource allocation in multi-agent systems.
• Experimental validation is necessary to assess the
framework performance in diverse practical set-
tings (cloud resource allocation, logistics, pub-
lic goods distribution etc.). This includes a for-
mal computational complexity analysis evaluating
scalability of the proposed algorithm as well as
execution time, memory usage, and performance
to assess the scalability of your approach while
varying distributions of preferences and goods.
• Apart from the theoretical study of the properties
such as envy-freeness or equitability another no-
tion that should be investigated is that of “stable”
allocation (the agents do not have any incentive to
further swap).
• When several agents are eligible for a swap based
on their profiles and predicted preferences, crite-
ria for choosing the most appropriate participants
need to be defined. This decision introduces po-
tential concerns regarding ethics and bias, particu-
larly when prioritizing agents could inadvertently
favor certain groups over others (Hurwicz, 1973).
• Furthermore, the notion of overall satisfaction
could be refined to incorporate subpopulation-
specific goals. For instance, rather than optimiz-
ing global satisfaction, the algorithm could priori-
tize improving the satisfaction of specific subpop-
ulations (e.g., AI-agents vs humans in hybrid soci-
eties) based on implicit or explicit norms (Aldew-
ereld et al., 2016). Similarly to as above, this issue
is directly related to the fairness and ethical con-
cerns of our approach.
REFERENCES
Aldewereld, H., Dignum, V., and Vasconcelos, W. W.
(2016). Group norms for multi-agent organisations.
ACM Transactions on Autonomous and Adaptive Sys-
tems (TAAS), 11(2):1–31.
Arrow, K. J. (2012). Social choice and individual values,
volume 12. Yale university press.
Brandt, F., Conitzer, V., Endriss, U., Lang, J., and Procac-
cia, A. D. (2016). Handbook of computational social
choice. Cambridge University Press.
Chevaleyre, Y., Dunne, P. E., Endriss, U., Lang, J.,
Lemaitre, M., Maudet, N., Padget, J., Phelps, S.,
Rodr
´
ıgues-Aguilar, J. A., and Sousa, P. (2005). Issues
in multiagent resource allocation.
Croitoru, M. and Croitoru, C. (2011). Generalised net-
work flows for combinatorial auctions. In 2011
IEEE/WIC/ACM International Conferences on Web
Intelligence and Intelligent Agent Technology, vol-
ume 2, pages 313–316. IEEE.
Dafoe, A., Hughes, E., Bachrach, Y., Collins, T., Mc-
Kee, K. R., Leibo, J. Z., Larson, K., and Graepel,
T. (2020). Open problems in cooperative ai. arXiv
preprint arXiv:2012.08630.
Hurwicz, L. (1973). The design of mechanisms for resource
allocation. The American Economic Review, 63(2):1–
30.
Ibaraki, T. and Katoh, N. (1988). Resource allocation prob-
lems: algorithmic approaches. MIT press.
Jordan, M. I. and Mitchell, T. M. (2015). Machine learn-
ing: Trends, perspectives, and prospects. Science,
349(6245):255–260.
Katoh, N. and Ibaraki, T. (1998). Resource allocation
problems. Handbook of Combinatorial Optimization:
Volume1–3, pages 905–1006.
Li, J., Ni, X., Yuan, Y., and Wang, F.-Y. (2018). A hier-
archical framework for ad inventory allocation in pro-
grammatic advertising markets. Electronic Commerce
Research and Applications, 31:40–51.
Vinothina, V. V., Sridaran, R., and Ganapathi, P. (2012). A
survey on resource allocation strategies in cloud com-
puting. International Journal of Advanced Computer
Science and Applications, 3(6).
Zhang, J., Zhang, M., Ren, F., and Liu, J. (2016). An in-
novation approach for optimal resource allocation in
emergency management. IEEE Transactions on Com-
puters.
ICAART 2025 - 17th International Conference on Agents and Artificial Intelligence
662