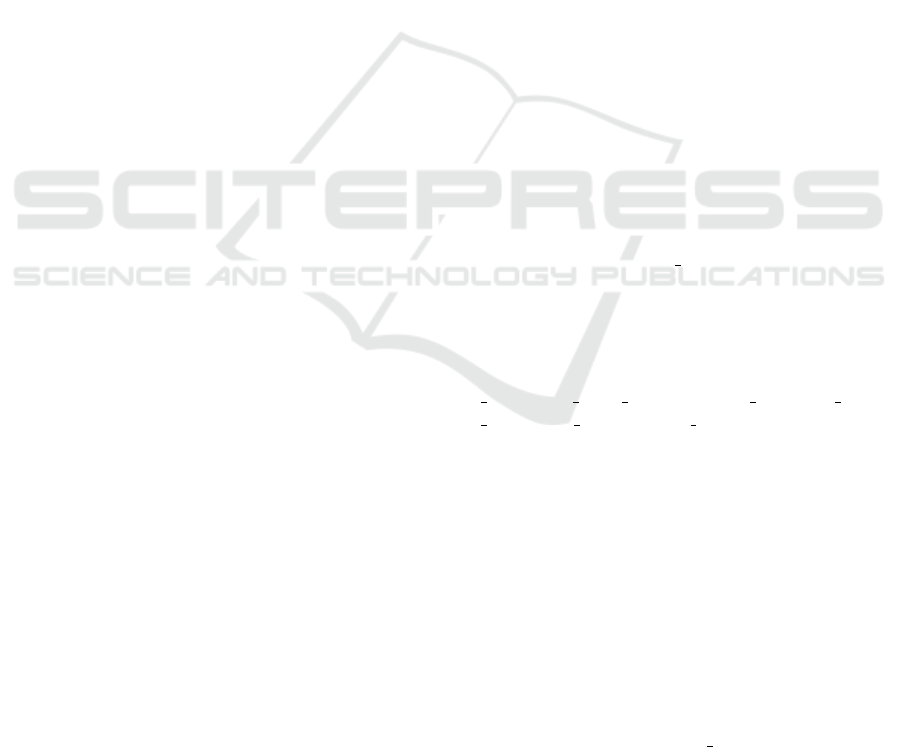
2 STATE OF THE ART
2.1 FHIR
®
Standard
Fast Healthcare Interoperability Resources (FHIR
®
)
(Benson and Grieve, 2016) is a new generation stan-
dard developed by HL7
®
(Health Level Seven) orga-
nization, to respond to the technology evolution of the
last years. The first version of the standard was pro-
posed in 2011. Since then, multiple versions were re-
leased. The latest version available during this work
is the version R5 (5.0.0). FHIR
®
defines two principal
components: resources and APIs. Resource types de-
fine the data elements, constraints, and relationships
with other resource types. They describe a modelling
of real-world clinical information. There are nearly
150 resource types defined by FHIR
®
covering most
of the known entities used in healthcare. FHIR
®
stan-
dard keeps a large flexibility to cover healthcare use
cases. It follows the Reuse and Composability princi-
ple, following the rule 80/20: FHIR
®
standard makes
the modelling of the most known elements, and keeps
the possibility for specific use cases to extend the core
resource types. FHIR
®
defines APIs to access the
resources, and to manipulate these resources. The
FHIR
®
API is following Level 2 of REST Maturity
Model (Ozdemir, 2020). The APIs defined by FHIR
®
have three levels of interactions: APIs at the resource
instance level, APIs at the resource type level, and
APIs at the system interaction level. Instance level in-
teractions allow mainly CRUD operations, and com-
partment access for defined HL7
®
compartments. Re-
source type level allows mainly search operations,
and the system level APIs allow batch and transaction
queries, as well as few other capabilities. The most
complicated API capability is the search at resource
type level, as every resource has sometimes dozens
of search parameters, and every search parameter can
have many searching variabilities.
FHIR
®
REST API is described under the page
RESTful API from the FHIR
®
standard, and the
search variabilities are described in the “Search” page
from the FHIR
®
standard (HL7, 2023). The FHIR
®
API variabilities are usually described under a Capa-
bilityStatement resource, exposed using the capabili-
ties interaction. A CapabilityStatement can describe
a FHIR
®
client or a server of resources. In our study,
we focus on server capabilities. The CapabilityState-
ment resource has three flavours: instance, capability,
and requirements. CapabilityStatement can describe
different behaviours of a FHIR
®
server, like the se-
curity of implementation, the implemented resources,
the system level interaction, the supported resources,
the operations for every resource, and the supported
search parameters for every resource.
2.2 Search Parameters Variabilities
Search parameters variabilities are described in the
“Search” page in the FHIR
®
standard. A search pa-
rameter can be described in a minimal manner in the
CapabilityStatement, with just exposing the search
parameter name, or it can be a complete descrip-
tion of the search parameter variations with a link
to a SearchParameter resource, describing the sup-
ported search variabilities. Every search parame-
ter can have multiple variabilities, many of them
can be documented in the SearchParameter resource.
For instance, modifiers can be described as part of
the SearchParameter resource, comparators as well.
Comparators are also called prefixes. All the vari-
abilities related to modifiers and comparators are de-
scribed in the documentation “RESTful API Search”
under FHIR
®
standard, and most of them depend on
the type of the search parameter. FHIR
®
defines nine
different search parameter types: date, number, quan-
tity, reference, string, token, uri, composite, and spe-
cial. Every search parameter type has many varia-
tions, like comparators, modifiers, the structure of the
searched value, and its precision.
Every resource has a specific number of search pa-
rameters, defined in the FHIR
®
standard (HL7, 2023).
Every resource of type DomainResource, can have an
extra search parameter: ‘ text’. In FHIR
®
R4, all the
resources extend DomainResource, except Bundle,
Parameters, and Binary. However, all the resources
extend Resource type, directly or through inheritance.
Thus, all the FHIR
®
R4 resources can implement any
search parameters from the Base Resource definition:
‘ content’, ‘ id’, ‘ lastUpdated’, ‘ profile’, ‘ query’,
‘ security’, ‘ source’, and ‘ tag’.
Figure 1 describes the number of search param-
eters for FHIR
®
R2, R3, R4 and R5. We collected
the number of the search parameters that FHIR
®
re-
sources can support, but we excluded the common
search parameters described above, which are inher-
ited from DomainResource and Resource. The num-
ber of resources did rise between R2 and R5 release
and was slightly stable after the release R4. However,
the number of search parameters continues to rise ev-
ery release. For R4, there are more than 1600 search
parameters.
Some common search parameters are quite com-
plicated and can have dozens of variations. For ex-
ample, the search parameter ‘ has’ is described by the
notion of reverse chaining. Every other resource that
can reference the current resource through a search
parameter can be used to search on the current in-
HEALTHINF 2025 - 18th International Conference on Health Informatics
298