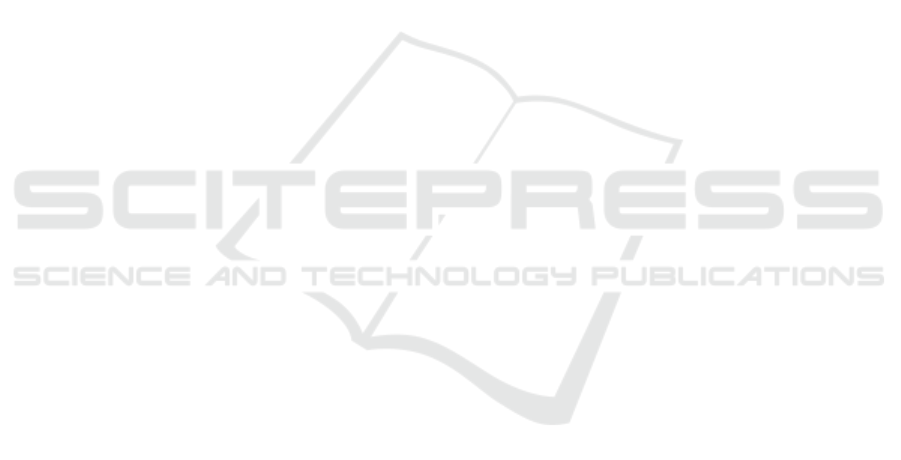
smile analysis and action unit information will be in-
vestigated to address the limitations of the current
approach. Furthermore, fusion with complementary
data modalities, such as audio and text transcriptions,
will be investigated to enhance the screening perfor-
mance.
ACKNOWLEDGEMENTS
We acknowledge the support of FAPES and CAPES
(Process 2021-2S6CD, FAPES No. 132/2021)
through the PDPG (Programa de Desenvolvimento da
P
´
os-Graduac¸
˜
ao – Parcerias Estrat
´
egicas nos Estados).
We sincerely thank Vera Lucia Carvalho Tess, Kelly
dos Santos Prado and the team at the Clinical Outpa-
tient Department for Pregnancy and Postpartum Care
at the Institute of Psychiatry at HCFMUSP for their
invaluable assistance in the recruitment and evalua-
tion of participants. Our gratitude also extends to Ana
Maria S. S. Oliveira and Luciana Cristina Ranhel for
their support in participant recruitment, and to Lucas
Batini Araujo and Herbert Dias de Souza for their
help in facilitating communication with participants
and acquiring the necessary data.
REFERENCES
Belharbi, S., Pedersoli, M., Koerich, A. L., Bacon, S., and
Granger, E. (2024). Guided interpretable facial ex-
pression recognition via spatial action unit cues. In
2024 IEEE 18th International Conference on Auto-
matic Face and Gesture Recognition (FG), pages 1–
10.
Biaggi, A., Conroy, S., Pawlby, S., and Pariante, C. M.
(2016). Identifying the women at risk of antenatal
anxiety and depression: A systematic review. Jour-
nal of affective disorders, 191:62–77.
Bian, Y., K
¨
uster, D., Liu, H., and Krumhuber, E. G.
(2024). Understanding naturalistic facial expressions
with deep learning and multimodal large language
models. Sensors, 24(1):126.
Bordes, F., Pang, R. Y., Ajay, A., Li, A. C., Bardes, A.,
Petryk, S., Ma
˜
nas, O., Lin, Z., Mahmoud, A., Jayara-
man, B., et al. (2024). An introduction to vision-
language modeling. arXiv preprint arXiv:2405.17247.
Caro-Fuentes, S. and Sanabria-Mazo, J. P. (2024). A
systematic review of the psychometric properties of
the patient health questionnaire-4 (phq-4) in clinical
and non-clinical populations. J. of the Academy of
Consultation-Liaison Psychiatry.
Darvariu, V.-A., Convertino, L., Mehrotra, A., and Mu-
solesi, M. (2020). Quantifying the relationships be-
tween everyday objects and emotional states through
deep learning based image analysis using smart-
phones. Proc. of the ACM on Interactive, Mobile,
Wearable and Ubiquitous Tech., 4(1):1–21.
Dowse, E., Chan, S., Ebert, L., Wynne, O., Thomas, S.,
Jones, D., Fealy, S., Evans, T.-J., and Oldmeadow, C.
(2020). Impact of perinatal depression and anxiety on
birth outcomes: a retrospective data analysis. Mater-
nal and child health journal, 24:718–726.
Goodfellow, I. J., Erhan, D., Carrier, P. L., Courville, A.,
Mirza, M., Hamner, B., Cukierski, W., Tang, Y.,
Thaler, D., Lee, D.-H., et al. (2013). Challenges in
representation learning: A report on three machine
learning contests. In Neural Inf. Process.: 20th Int.
Conf., Daegu, Korea, November 3-7, 2013. Proc., Part
III 20, pages 117–124. Springer.
Gupta, S., Kumar, P., and Tekchandani, R. K. (2023). Fa-
cial emotion recognition based real-time learner en-
gagement detection system in online learning context
using deep learning models. Multimedia Tools and
Applicat., 82(8):11365–11394.
Harris, P. A., Taylor, R., Thielke, R., Payne, J., Gonza-
lez, N., and Conde, J. G. (2009). Research electronic
data capture (redcap)—a metadata-driven methodol-
ogy and workflow process for providing translational
research informatics support. J. of Biomed. Informat.,
42(2):377–381.
He, K., Zhang, X., Ren, S., and Sun, J. (2016). Deep resid-
ual learning for image recognition. In Proc. of the
IEEE Conf. on Comput. Cision and Pattern Recogni-
tion, pages 770–778.
Javadekar, A., Karmarkar, A., Chaudhury, S., Saldanha,
D., and Patil, J. (2023). Biopsychosocial correlates
of emotional problems in women during pregnancy
and postpartum period. Industrial Psychiatry Journal,
32(Suppl 1):S141–S146.
Kong, X., Yao, Y., Wang, C., Wang, Y., Teng, J., and Qi, X.
(2022). Automatic identification of depression using
facial images with deep convolutional neural network.
Medical Science Monitor: Int. Med. J. of Experimen-
tal and Clinical Research, 28:e936409–1.
Kumar, P., Vedernikov, A., and Li, X. (2024). Measur-
ing non-typical emotions for mental health: A sur-
vey of computational approaches. arXiv preprint
arXiv:2403.08824.
Kurki, T., Hiilesmaa, V., Raitasalo, R., Mattila, H., and
Ylikorkala, O. (2000). Depression and anxiety in early
pregnancy and risk for preeclampsia. Obstetrics &
Gynecology, 95(4):487–490.
Li, H., Bowen, A., Bowen, R., Muhajarine, N., and Bal-
buena, L. (2021). Mood instability, depression, and
anxiety in pregnancy and adverse neonatal outcomes.
BMC Pregnancy and Childbirth, 21:1–9.
Li, S., Deng, W., and Du, J. (2017). Reliable crowdsourcing
and deep locality-preserving learning for expression
recognition in the wild. In Proc. of the IEEE Conf. on
Comput. Vision and Pattern Recognition, pages 2852–
2861.
Liu, D., Liu, B., Lin, T., Liu, G., Yang, G., Qi, D., Qiu,
Y., Lu, Y., Yuan, Q., Shuai, S. C., et al. (2022). Mea-
suring depression severity based on facial expression
and body movement using deep convolutional neural
network. Frontiers in psychiatry, 13:1017064.
AI-Driven Early Mental Health Screening: Analyzing Selfies of Pregnant Women
317