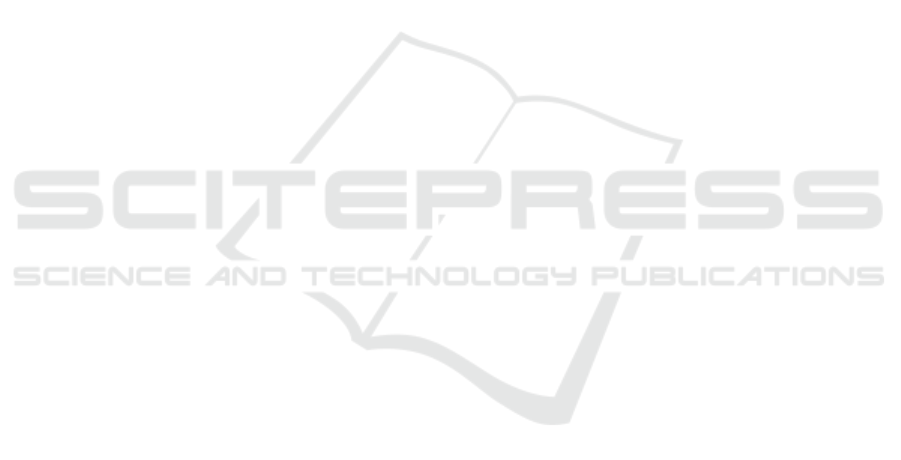
tailed views of data series provided users with a sim-
plified navigation experience. An empirical evalu-
ation was conducted with 14 healthcare profession-
als affiliated with the Montpellier University Hospi-
tal. Results indicated an accuracy rate of 89% in
task resolution, while usability assessments using the
SUS scale placed the interface in the top quartile
for usability. Additionally, qualitative feedback ob-
tained from open-ended questions identified potential
areas for improvement. To the best of our knowl-
edge, GLIMPSE-Med is the first interface across do-
mains to visually integrate data quality and prediction
outputs with multivariate time series in a single on-
screen view. In the medical field, it is also the first
to compress both the temporal axis and the modality
axis. Although tested exclusively in a medical con-
text, GLIMPSE-Med is not limited to this use case.
The evaluation demonstrated that GLIMPSE-Med ef-
fectively addressed the problem at hand; however,
qualitative feedback and discussions with users high-
lighted opportunities for further interface improve-
ments.
REFERENCES
Aigner, W. and Miksch, S. (2006). CareVis: integrated
visualization of computerized protocols and tempo-
ral patient data. Artificial Intelligence in Medicine,
37(3):203–218.
Aigner, W., Miksch, S., Schumann, H., and Tominski, C.
(2011). Visualization of Time-Oriented Data. Human-
Computer Interaction Series. Springer, London.
Alonso, D. L., Rose, A., Plaisant, C., and Norman, K. L.
(1998). Viewing personal history records: A compar-
ison of tabular format and graphical presentation us-
ing LifeLines. Behaviour & Information Technology,
17(5):249–262.
Bade, R., Schlechtweg, S., and Miksch, S. (2004). Con-
necting time-oriented data and information to a co-
herent interactive visualization. In Proceedings of the
SIGCHI Conference on Human Factors in Computing
Systems, (CHI ’04), pages 105–112, New York, NY,
USA. Association for Computing Machinery.
Bangor, A., Kortum, P. T., and Miller, J. T. (2008). An
empirical evaluation of the system usability scale. In-
ternational Journal of Human–Computer Interaction,
24(6):574–594.
Brooke, J. (1996). SUS - A quick and dirty usability scale.
CRC Press.
Combi, C., Keravnou-Papailiou, E., and Shahar, Y. (2010).
Temporal Information Systems in Medicine. Springer
US, Boston, MA.
Devlin, J., Chang, M.-W., Lee, K., and Toutanova, K.
(2019). BERT: Pre-training of deep bidirectional
transformers for language understanding. In Proceed-
ings of the 2019 Conference of the North American
Chapter of the Association for Computational Lin-
guistics: Human Language Technologies, Volume 1
(Long and Short Papers), pages 4171–4186. Associ-
ation for Computational Linguistics.
Faiola, A. and Newlon, C. (2011). Advancing Critical Care
in the ICU: A Human-Centered Biomedical Data Vi-
sualization Systems. In Robertson, M. M., editor, Er-
gonomics and Health Aspects of Work with Comput-
ers, Lecture Notes in Computer Science, pages 119–
128, Berlin, Heidelberg. Springer.
Goldberger, A. L., Amaral, L. A. N., Glass, L., Hausdorff,
J. M., Ivanov, P. C., Mark, R. G., Mietus, J. E., Moody,
G. B., Peng, C.-K., and Stanley, E. (2000). Phys-
ioBank, PhysioToolkit, and PhysioNet: Components
of a new research resource for complex physiologic
signals. Circulation, 101(23):E215–220.
Gronier, G. and Baudet, A. (2021). Psychometric eval-
uation of the F-SUS: Creation and validation of the
french version of the system usability scale. In-
ternational Journal of Human–Computer Interaction,
37(16):1571–1582.
Jalali, V. and Kaur, D. (2020). A study of classification
and feature extraction techniques for brain tumor de-
tection. International Journal of Multimedia Informa-
tion Retrieval, 9(4):271–290.
Johnson, A. E. W., Pollard, T. J., and Mark, R. G. (2015).
MIMIC-III Clinical Database.
Johnson, A. E. W., Pollard, T. J., Shen, L., Lehman, L.-
W. H., Feng, M., Ghassemi, M., Moody, B., Szolovits,
P., Celi, L. A., and Mark, R. G. (2016). MIMIC-III,
a freely accessible critical care database. Scientific
Data, 3:160035.
Linhares, C. D. G., Lima, D. M., Ponciano, J. R., Oli-
vatto, M. M., Gutierrez, M. A., Poco, J., Traina, C.,
and Traina, A. J. M. (2023). ClinicalPath: A visu-
alization tool to improve the evaluation of electronic
health records in clinical decision-making. IEEE
Transactions on Visualization and Computer Graph-
ics, 29(10):4031–4046.
Munzner, T. (2009). A nested model for visualization de-
sign and validation. IEEE Transactions on Visualiza-
tion and Computer Graphics, 15(6):921–928.
Qureshi, I., Yan, J., Abbas, Q., Shaheed, K., Riaz, A. B.,
Wahid, A., Khan, M. W. J., and Szczuko, P. (2023).
Medical image segmentation using deep semantic-
based methods: A review of techniques, applications
and emerging trends. Information Fusion, 90:316–
352.
Rind, A., Wang, T. D., Aigner, W., Miksch, S., Wongsupha-
sawat, K., Plaisant, C., and Shneiderman, B. (2013).
Interactive Information Visualization to Explore and
Query Electronic Health Records. Foundations and
Trends® in Human–Computer Interaction, 5(3):207–
298.
Scheer, J., Volkert, A., Brich, N., Weinert, L., Santhanam,
N., Krone, M., Ganslandt, T., Boeker, M., and Nagel,
T. (2022). Visualization techniques of time-oriented
data for the comparison of single patients with mul-
tiple patients or cohorts: scoping review. Journal of
Medical Internet Research, 24(10):e38041.
IVAPP 2025 - 16th International Conference on Information Visualization Theory and Applications
774