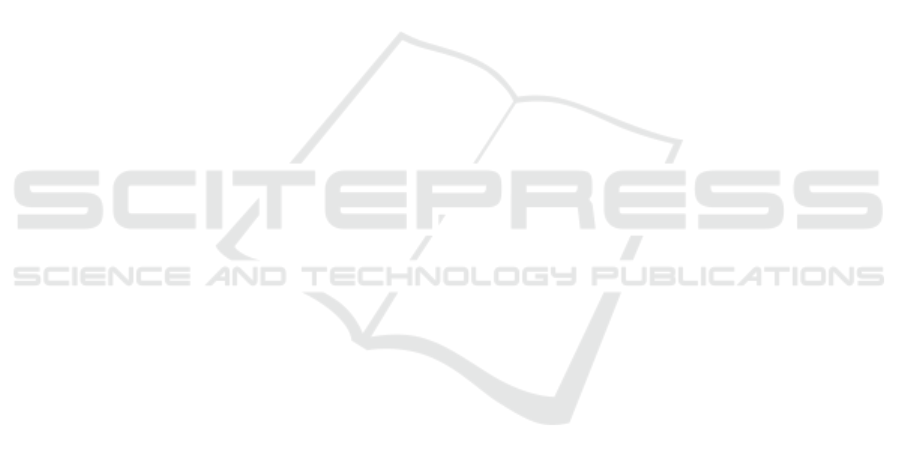
A DQN is trained using data generated through re-
peated interactions with the environment in which the
decision making problem is being solved. Each in-
teraction warrants a feedback which then improves its
performance. As the DQN weights are randomly ini-
tialized the interaction is suboptimal or even danger-
ous at the beginning. In order to overcome this issue,
a DQN is pre-trained using data associated with ex-
pert interactions with the environment. As this stage
is before the DQN gets to interact with the environ-
ment, the previously mentioned dangers are averted.
The pre-training phase is also called the offline learn-
ing phase. The training phase where the DQN in-
teracts with the environment (and continues to train)
is called the online phase. Training DQNs in two
phases, offline followed by an online phase, is a pop-
ular and practical training paradigm. This will the fo-
cus of this paper.
1.1 Problem Description and Our
Contribution
While a target network has been demonstrated to be
useful in stabilizing learning for DQL, its contribution
to finding better policies remains under-explored. In
(Mnih et al., 2015), the significance of a target net-
work is highlighted in mitigating the moving target
problem and in enhancing stability. However, there is
a lack of empirical evidence for its necessity through-
out the entire training process (Mnih et al., 2015). Re-
cent mathematical proofs, e.g., in (Ramaswamy and
H
¨
ullermeier, 2022) suggest that the target network
could be removed at certain stages of training. These
proofs consider both the online and offline phases
of learning. Recall that online learning refers to the
learning paradigm wherein the learning agent influ-
ences the data used for training in a direct “ongoing”
manner. In offline learning, the agent is trained us-
ing data that was collected in the past - pre-training
- and often the agent has no influence on this train-
ing data (Hester et al., 2017). As mentioned before
an offline learning phase (pre-training) typically pre-
cedes an online learning phase. This kind of training
is called pre-trained online learning.
1. When an agent is trained in two phases - the of-
fline followed by an online phase, a target network
can be completely omitted.
2. In order to find the best policy, we observed that
there is an optimal amount of pre-training. Too
little affects stability during the online phase, too
much affects optimality.
3. As compared to training with a target network, the
training without a target network has lower vari-
ance. Hence, learning is faster!
1.2 Related Work
There have been several other works that have ques-
tioned the superfluity of a target network. For ex-
ample, in (Ramaswamy et al., 2023), it is suggested
that the target network could be removed in online
learning by replacing the activation function used in
the DQN with their newly developed Truncated Gaus-
sian Error Linear Unit (TGeLU) activation function
(Ramaswamy et al., 2023). This paper seeks to ad-
dress the practical implications of these theoretical
insights. The central question guiding this research is:
“How does the removal of the target network at vari-
ous scenarios and stages of training impact the stabil-
ity and effectiveness of DQL?”
In DQL, a target network is employed alongside
the main network during the training process to calcu-
late the Mean Square Error (MSE) loss. The primary
purpose of integrating a target network in DQL train-
ing is to address the moving target problem, which
arises from high variance in Q-value estimates, lead-
ing to unstable learning. In addition to the moving tar-
get problem affecting stable learning, NNs using non-
linear activation functions also suffer from a problem
referred to as numerical instability or exploding gra-
dient, which is caused by high variance. During the
backpropagation phase, the gradient will grow expo-
nentially, and if the loss value gets too high, this will
result in the weights becoming too large to handle
(Philipp et al., 2018).
By using a target network with less frequent up-
dates. This means that the target Q-values change
more slowly and are suggested to contribute to a
smoother training process, allowing for more reliable
convergence to an optimal policy, but at the same
time may slow down the learning process (Mnih et al.,
2015). Using a target network will also contribute to
a higher memory-consuming due to the need to keep
a copy of the network during training, which makes
the memory demand twice as much compared to only
using the main network. While it may be useful at
the beginning of the training to use a target network
to obtain stable learning, there is no proof that using
one will result in better policies.
Removing the target network to reduce the overes-
timation of Q-values and decrease memory demand is
not a novel idea per se, but the approach to removing
the target network and some of the findings we have
discovered are new. In this paper, we have been able
to show that it is possible for an agent to achieve just
as good or better policy than an agent trained with a
target network for the entire process without any ma-
ICPRAM 2025 - 14th International Conference on Pattern Recognition Applications and Methods
438