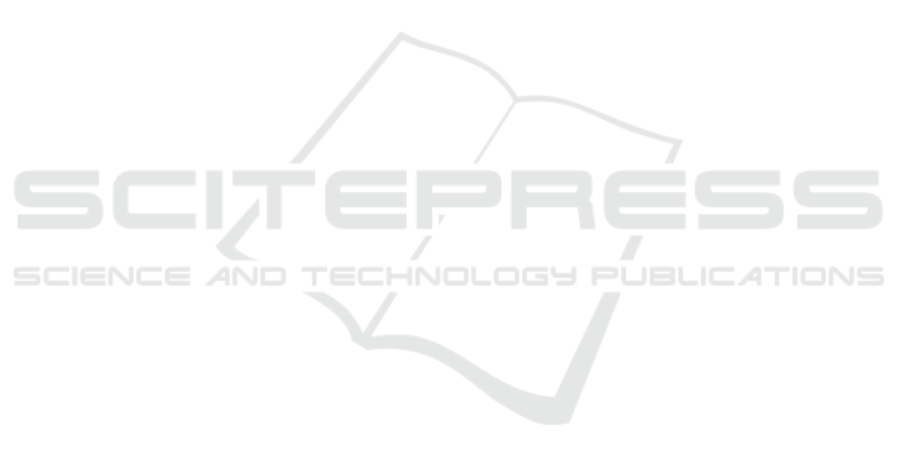
future objective is to represent the point cloud using a
single projection plane and use our model to densify
it. This approach would enable us to generate paral-
lax mapping for a coarse 3D mesh, maintaining visual
detail while optimizing computational efficiency.
REFERENCES
Alhaija, H. A., Mustikovela, S. K., Mescheder, L., Geiger,
A., and Rother, C. (2017). Augmented reality meets
deep learning for car instance segmentation in urban
scenes. In British machine vision conference, vol-
ume 1.
Chabra, R., Lenssen, J. E., Ilg, E., Schmidt, T., Straub,
J., Lovegrove, S., and Newcombe, R. (2020). Deep
local shapes: Learning local sdf priors for detailed
3d reconstruction. In Computer Vision–ECCV 2020:
16th European Conference, Glasgow, UK, August 23–
28, 2020, Proceedings, Part XXIX 16, pages 608–625.
Springer.
Chang, A. X., Funkhouser, T., Guibas, L., Hanrahan,
P., Huang, Q., Li, Z., Savarese, S., Savva, M.,
Song, S., Su, H., et al. (2015). Shapenet: An
information-rich 3d model repository. arXiv preprint
arXiv:1512.03012.
Chibane, J., Alldieck, T., and Pons-Moll, G. (2020). Im-
plicit functions in feature space for 3d shape recon-
struction and completion. In Proceedings of the
IEEE/CVF conference on computer vision and pattern
recognition, pages 6970–6981.
Choy, C. B., Xu, D., Gwak, J., Chen, K., and Savarese,
S. (2016). 3d-r2n2: A unified approach for single
and multi-view 3d object reconstruction. In Computer
Vision–ECCV 2016: 14th European Conference, Am-
sterdam, The Netherlands, October 11-14, 2016, Pro-
ceedings, Part VIII 14, pages 628–644. Springer.
Curless, B. and Levoy, M. (1996). A volumetric method for
building complex models from range images. In Pro-
ceedings of the 23rd annual conference on Computer
graphics and interactive techniques, pages 303–312.
Genova, K., Cole, F., Sud, A., Sarna, A., and Funkhouser,
T. (2020). Local deep implicit functions for 3d shape.
In Proceedings of the IEEE/CVF Conference on Com-
puter Vision and Pattern Recognition, pages 4857–
4866.
Lionar, S., Emtsev, D., Svilarkovic, D., and Peng, S.
(2021a). Dynamic plane convolutional occupancy net-
works. In Proceedings of the IEEE/CVF Winter Con-
ference on Applications of Computer Vision, pages
1829–1838.
Lionar, S., Schmid, L., Cadena, C., Siegwart, R., and Cra-
mariuc, A. (2021b). Neuralblox: Real-time neural
representation fusion for robust volumetric mapping.
In 2021 International Conference on 3D Vision (3DV),
pages 1279–1289. IEEE.
Mescheder, L., Oechsle, M., Niemeyer, M., Nowozin, S.,
and Geiger, A. (2019). Occupancy networks: Learn-
ing 3d reconstruction in function space. In Proceed-
ings of the IEEE/CVF conference on computer vision
and pattern recognition, pages 4460–4470.
Papa, L., Russo, P., and Amerini, I. (2023). Meter: a mobile
vision transformer architecture for monocular depth
estimation. IEEE Transactions on Circuits and Sys-
tems for Video Technology, pages 1–1.
Park, J. J., Florence, P., Straub, J., Newcombe, R., and
Lovegrove, S. (2019). Deepsdf: Learning continu-
ous signed distance functions for shape representa-
tion. In Proceedings of the IEEE/CVF conference on
computer vision and pattern recognition, pages 165–
174.
Patil, A. G., Qian, Y., Yang, S., Jackson, B., Bennett, E.,
and Zhang, H. (2023). Rosi: Recovering 3d shape
interiors from few articulation images. arXiv preprint
arXiv:2304.06342.
Peng, S., Niemeyer, M., Mescheder, L., Pollefeys, M.,
and Geiger, A. (2020). Convolutional occupancy net-
works. In Computer Vision–ECCV 2020: 16th Euro-
pean Conference, Glasgow, UK, August 23–28, 2020,
Proceedings, Part III 16, pages 523–540. Springer.
Qi, C. R., Su, H., Mo, K., and Guibas, L. J. (2017). Point-
net: Deep learning on point sets for 3d classification
and segmentation. In Proceedings of the IEEE con-
ference on computer vision and pattern recognition,
pages 652–660.
Rao, Y., Liu, Z., Zhao, W., Zhou, J., and Lu, J. (2023). Dy-
namic spatial sparsification for efficient vision trans-
formers and convolutional neural networks. IEEE
Transactions on Pattern Analysis and Machine Intel-
ligence, 45(9):10883–10897.
Sitzmann, V., Martel, J. N. P., Bergman, A. W., Lindell,
D. B., and Wetzstein, G. (2020). Implicit neural rep-
resentations with periodic activation functions.
Tang, J., Lei, J., Xu, D., Ma, F., Jia, K., and Zhang, L.
(2021). Sa-convonet: Sign-agnostic optimization of
convolutional occupancy networks. In Proceedings of
the IEEE/CVF International Conference on Computer
Vision, pages 6504–6513.
Tonti, C. M., Papa, L., and Amerini, I. (2024). Lightweight
3-D Convolutional Occupancy Networks for Virtual
Object Reconstruction . IEEE Computer Graphics
and Applications, 44(02):23–36.
Touvron, H., Cord, M., El-Nouby, A., Verbeek, J., and
J
´
egou, H. (2022). Three things everyone should know
about vision transformers. In European Conference
on Computer Vision, pages 497–515. Springer.
Urmson, C., Anhalt, J., Bagnell, D., Baker, C., Bittner, R.,
Clark, M., Dolan, J., Duggins, D., Galatali, T., Geyer,
C., et al. (2008). Autonomous driving in urban envi-
ronments: Boss and the urban challenge. Journal of
field Robotics, 25(8):425–466.
Virtanen, J.-P., Daniel, S., Turppa, T., Zhu, L., Julin, A.,
Hyypp
¨
a, H., and Hyypp
¨
a, J. (2020). Interactive dense
point clouds in a game engine. ISPRS Journal of Pho-
togrammetry and Remote Sensing, 163:375–389.
Zhu, Y., Mottaghi, R., Kolve, E., Lim, J. J., Gupta, A., Fei-
Fei, L., and Farhadi, A. (2017). Target-driven visual
navigation in indoor scenes using deep reinforcement
learning. In 2017 IEEE international conference on
robotics and automation (ICRA), pages 3357–3364.
IEEE.
GRAPP 2025 - 20th International Conference on Computer Graphics Theory and Applications
414