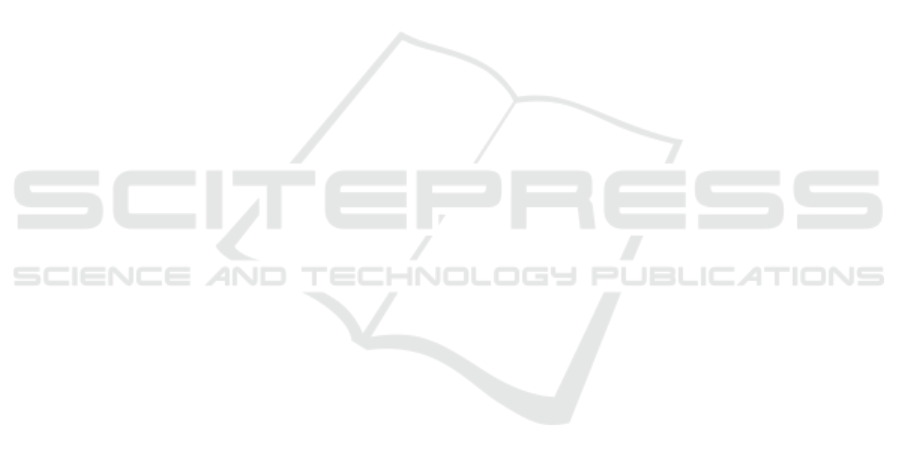
the literature findings to achieve a high degree of gen-
eralization of the proposed model. By this approach
we try to ensure its compatibility for easier adoption
in the business contexts.
4.1 Limitations
Although the proposed artifact is based on an exten-
sive and consistent literature analysis and designed
using a standard modeling language, we acknowledge
that we can not yet claim an overall standardization
and generalization. To reach this point, a number of
real-world use cases must be applied and tested to
prove the proposed model’s practical validity. We rec-
ognize this limitation and aim to address it in our fu-
ture work.
5 CONCLUSION AND OUTLOOK
Successful integration of LLMs into an enterprise
presents numerous challenges and necessitates the
adoption of LLMOps. Standardizing LLMOps prac-
tices may provide significant advantages to businesses
seeking to effectively manage the LLM life cycle
while ensuring workflow consistency across various
teams and projects. In this paper, we conducted an ex-
tensive SLR on the existing standard LLMOps mod-
els in both academic and industrial literature. Fur-
thermore, we consolidated an overview of identified
LLMOps processes and designed a cyclic LLMOps
representation in Figure 2 and Figure 3 as a visual
summary of the analyzed literature. Both figures are
utilized as a trustworthy research foundation for our
artifact.
Based on the SLR findings, we concluded that
none of the discovered paper proposed a formal-
ization or standardization model for LLMOps adop-
tion. Moreover, none of the obtained findings relied
on some standard modeling languages (e.g., BPMN,
Unified Modeling Language). To address the iden-
tified research gap, we proposed an use-case and
tool-agnostic LLMOps business model designed us-
ing BPMN. The model is designed to formalize and
standardize the main steps required to effectively in-
tegrate LLMOps into various business scenarios. The
conducted SLR and obtained findings answer RQ 1..
The proposed BPMN model in Figure 4 addresses and
answers RQ 2..
As discussed previously, in the subsection 4.1, the
presented work is subject to certain limitations that we
intend to tackle in our future work. Firstly, we aim to
test the proposed artifact on the real-world use cases
from various domains to prove its generalization. The
discovered findings might lead to further adjustments
and refinements of the presented model. Secondly, we
intend to elaborate more on specific type of activities
and the related tasks and roles. Therefore, we believe
that the presented results and artifact should not be re-
garded as final but rather considered as starting point.
REFERENCES
Arawjo, I., Swoopes, C., Vaithilingam, P., Wattenberg, M.,
and Glassman, E. L. (2024). ChainForge: A Visual
Toolkit for Prompt Engineering and LLM Hypothesis
Testing. In Mueller, F. F., Kyburz, P., Williamson,
J. R., Sas, C., Wilson, M. L., Dugas, P. T., and
Shklovski, I., editors, Proceedings of the CHI Confer-
ence on Human Factors in Computing Systems, ACM
Digital Library, pages 1–18. Association for Comput-
ing Machinery.
Basak, P. (2024). Unlocking fmops/llmops with airflow:
A guide to operationalizing and managing large lan-
guage models. Technical report, Airflow Summit
2024.
Chen, T. (2024). Challenges and opportunities in integrat-
ing llms into continuous integration/continuous de-
ployment (ci/cd) pipelines. In 2024 5th International
Seminar on Artificial Intelligence, Networking and In-
formation Technology (AINIT), pages 364–367.
Cruzes, D. S. and Dyba, T. (2011). Recommended steps for
thematic synthesis in software engineering. In 2011
international symposium on empirical software engi-
neering and measurement, pages 275–284. IEEE.
Datta, A., Sen, S., and Bandyopadhyay, A. (2024). Llmops
explained. Technical report, TruEra and Intel Corpo-
ration.
Diaz-De-Arcaya, J., L
´
opez-De-Armentia, J., Mi
˜
n
´
on, R.,
Ojanguren, I. L., and Torre-Bastida, A. I. (2024).
Large language model operations (llmops): Defini-
tion, challenges, and lifecycle management. In 2024
9th International Conference on Smart and Sustain-
able Technologies (SpliTech), pages 1–4. IEEE.
Fujitsu (2024). Fujitsu composite ai. Technical report, Fu-
jitsu Limited.
He, Z., Liang, T., Jiao, W., Zhang, Z., Yang, Y., Wang,
R., Tu, Z., Shi, S., and Wang, X. (2023). Exploring
human-like translation strategy with large language
models. arXiv preprint arXiv:2305.04118.
Hevner, A. R., March, S. T., Park, J., and Ram, S. (2004).
Design science in information systems research. MIS
quarterly, pages 75–105.
Huang, K., Wang, Y., Goertzel, B., Li, Y., Wright, S., and
Ponnapalli, J. (2024). Generative AI Security: Theo-
ries and Practices. Springer.
Kamath, U., Keenan, K., Somers, G., and Sorenson, S.
(2024). Large Language Models: A Deep Dive.
Springer.
Kartakis, S. and Hotz, H. (2023). Fmops/llmops: Opera-
tionalise generative ai using mlops principles. Techni-
cal report, Amazon Web Services Inc.
Towards a Standardized Business Process Model for LLMOps
865