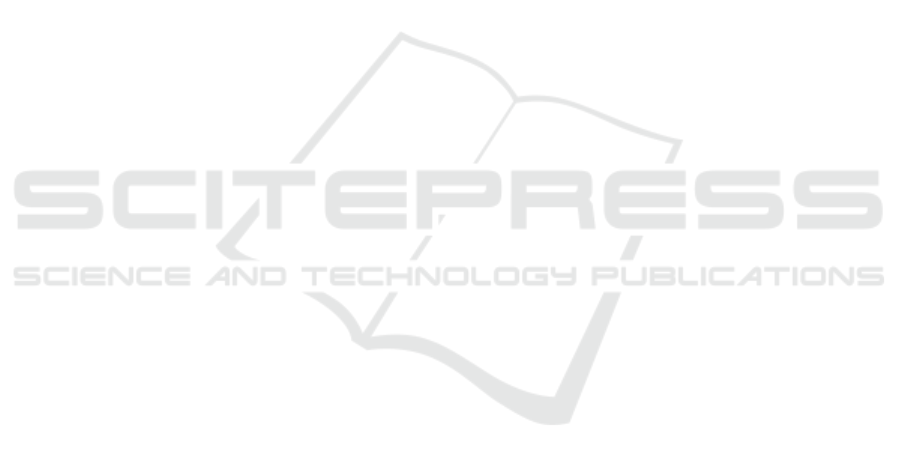
2014). This preference extends to architectural plans
as well. Additionally, tools that enable new ways of
interacting with these plans can be particularly ben-
eficial. The potential of generative AI in architec-
tural education goes far beyond automation. Research
suggests that carefully integrating these tools can en-
hance creativity and improve time management. For
example, studies have shown that architecture stu-
dents often use generative AI during the concept de-
velopment stage to visualize ideas and streamline
workflows, leading to clearer concepts and greater
efficiency (Kee et al., 2024). Moreover, AI-driven
tools like ArchiGuesser have demonstrated the abil-
ity to make learning architectural history more in-
teractive and engaging through gamified experiences
(Ploennigs and Berger, 2023).Generative AI’s capa-
bilities extend further, as shown in its ability to gener-
ate multimodal outputs, such as text-to-image models,
which have been explored for educational and design
ideation purposes (Koh et al., 2023).
Despite these advantages, questions persist about
the effectiveness and ethical implications of using
generative AI in education. Comparisons between
traditional methods and AI-supported workflows re-
veal striking differences in how creativity, human
agency, and educational outcomes are approached.
Traditional methods, rooted in manual drafting and
iterative instructor feedback, often emphasize foun-
dational skills and critical thinking. On the other
hand, generative AI offers faster ideation and en-
hanced visualization, raising concerns about potential
over-reliance on automation and the erosion of essen-
tial skills. Additionally, within the realm of generative
AI, there is a great contrast between general-purpose
tools like ChatGPT and specialized AI systems built
for architectural applications. While the former prior-
itizes accessibility and ease of use, the latter delivers
targeted solutions, supporting tasks such as sketch-
to-model transformation, generative floor plans, and
context-aware feedback tailored to architectural edu-
cation (Li et al., 2024b).
This paper focuses on the contrasts between tradi-
tional methods, general-purpose generative AI tools,
and custom AI solutions. By examining the impact of
these approaches on architectural education, it aims
to highlight the most effective ways generative AI can
enhance learning outcomes.
2 METHODOLOGY
This study employs a qualitative framework to eval-
uate the role of generative artificial intelligence
(GenAI) in architectural education. The methodology
involves a comparative analysis of traditional non-AI
approaches, generative AI tools, and the custom-built
Architecture AI Tool (ArchAI), emphasizing their
respective contributions to creativity, learning effi-
ciency, and design workflows. Additionally, the study
contrasts stock generative AI solutions, such as Chat-
GPT and Claude AI, with the custom tool to identify
domain-specific advantages. Images from the Archi-
tecture AI Tool (ArchAI) and its outputs will be inte-
grated for demonstrative purposes.
2.1 Comparative Framework:
Generative AI vs. Non-Generative
AI
The study examines the differences between gener-
ative AI-enabled workflows and traditional non-AI-
supported methods. Generative AI offers significant
advantages in automating repetitive tasks, providing
real-time feedback, and enhancing visualization ca-
pabilities. These aspects are compared against non-
generative approaches, which emphasize manual ef-
fort and critical thinking.
Firstly, in terms of Automated Design Assistance,
generative AI tools, such as the Architecture AI Tool
(ArchAI), automate blueprint analysis, layout sugges-
tions, and design visualization, allowing students to
focus on conceptual development. Non-generative
methods rely on manual iterations and instructor-led
feedback, which, while foundational, are less efficient
for rapid prototyping. By manually adjusting layouts,
students spend more time learning technical processes
but less time innovating and exploring creative possi-
bilities.
Secondly, regarding Feedback and Iteration, the
Architecture AI Tool (ArchAI) provides actionable,
data-driven feedback linked to uploaded blueprints,
allowing immediate refinement. This is achieved
by analyzing spatial layouts, identifying inefficien-
cies, and suggesting practical improvements based
on its integrated architectural knowledge base. Tra-
ditional methods depend on instructor evaluations,
which, while valuable for mentorship and guidance,
are constrained by the availability of instructors and
often lack scalability for larger cohorts. The reliance
on periodic feedback slows the iteration process and
reduces the agility of student workflows.
Thirdly, in the aspect of Visual Outputs, gener-
ative AI tools generate updated images and alterna-
tive layouts from user inputs, enhancing creativity
through visual exploration. This visual immediacy
enables students to evaluate multiple design iterations
in real-time. Non-generative methods, though rein-
forcing manual drafting skills, require extensive effort
GRAPP 2025 - 20th International Conference on Computer Graphics Theory and Applications
416