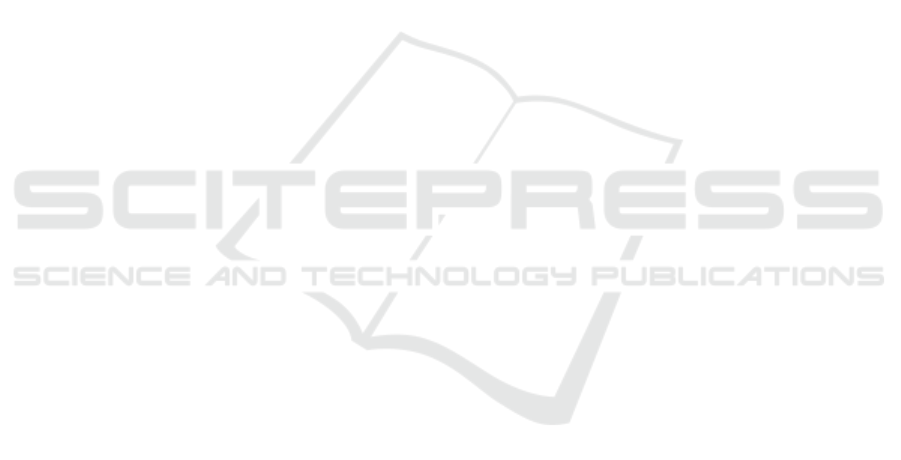
fective Camera-to-Lidar Spatiotemporal Calibration
Based on a Simple Calibration Target.
Gunatilake, A., Piyathilaka, L., Tran, A., Vishwanathan,
V. K., Thiyagarajan, K., and Kodagoda, S. (2021).
Stereo Vision Combined With Laser Profiling for
Mapping of Pipeline Internal Defects. IEEE Sensors
Journal, 21(10):11926–11934.
Hansen, P., Alismail, H., Rander, P., and Browning, B.
(2011). Monocular visual odometry for robot local-
ization in LNG pipes. In 2011 IEEE International
Conference on Robotics and Automation, pages 3111–
3116. IEEE.
Heng, L., Li, B., and Pollefeys, M. (2013). CamOd-
oCal: Automatic intrinsic and extrinsic calibration of
a rig with multiple generic cameras and odometry. In
2013 IEEE/RSJ International Conference on Intelli-
gent Robots and Systems, pages 1793–1800. IEEE.
Kakogawa, A., Komurasaki, Y., and Ma, S. (2019).
Shadow-based operation assistant for a pipeline-
inspection robot using a variance value of the image
histogram. Journal of Robotics and Mechatronics,
31(6):772–780.
Kannala, J. and Brandt, S. S. (2006). A generic camera
model and calibration method for conventional, wide-
angle, and fish-eye lenses. IEEE Transactions on Pat-
tern Analysis and Machine Intelligence, 28(8):1335–
1340.
Kannala, J., Brandt, S. S., and Heikkil
¨
a, J. (2008). Measur-
ing and modelling sewer pipes from video. Machine
Vision and Applications, 19(2):73–83.
Karkoub, M., Bouhali, O., and Sheharyar, A. (2021). Gas
pipeline inspection using autonomous robots with
omni-directional cameras. IEEE Sensors Journal,
21(14):15544–15553.
Kazeminasab, S., Aghashahi, M., and Banks, M. K. (2020).
Development of an inline robot for water quality
monitoring. In 2020 5th International Conference
on Robotics and Automation Engineering (ICRAE),
pages 106–113.
Matsui, K., Yamashita, A., and Kaneko, T. (2010).
3-D shape measurement of pipe by range finder
constructed with omni-directional laser and omni-
directional camera. In 2010 IEEE International Con-
ference on Robotics and Automation, pages 2537–
2542. IEEE.
Moein, E. and Himan, H. J. (2022). Automated Condition
Assessment of Sanitary Sewer Pipes Using LiDAR In-
spection Data, pages 136–144. Proceedings. Ameri-
can Society of Civil Engineers (ASCE).
Mor
´
e, J. J. (1978). The levenberg-marquardt algorithm: Im-
plementation and theory. In Watson, G. A., editor, Nu-
merical Analysis, pages 105–116, Berlin, Heidelberg.
Springer Berlin Heidelberg.
Rusinkiewicz, S. and Levoy, M. (2001). Efficient variants
of the ICP algorithm. In Proceedings Third Interna-
tional Conference on 3-D Digital Imaging and Mod-
eling, pages 145–152. IEEE.
Scaramuzza, D., Martinelli, A., and Siegwart, R. (2006). A
Toolbox for Easily Calibrating Omnidirectional Cam-
eras. In 2006 IEEE/RSJ International Conference
on Intelligent Robots and Systems, pages 5695–5701.
IEEE.
Sepulveda-Valdez, C., Sergiyenko, O., Alaniz-Plata, R.,
N
´
u
˜
nez-L
´
opez, J. A., Tyrsa, V., Flores-Fuentes, W.,
Rodriguez-Qui
˜
nonez, J. C., Mercorelli, P., Kolen-
dovska, M., Kartashov, V., Miranda-Vega, J. E., and
Murrieta-Rico, F. N. (2023). Laser Scanning Point
Cloud Improvement by Implementation of RANSAC
for Pipeline Inspection Application. In IECON 2023-
49th Annual Conference of the IEEE Industrial Elec-
tronics Society, pages 1–6. IEEE.
Sepulveda-Valdez, C., Sergiyenko, O., Tyrsa, V., Mer-
corelli, P., Rodr
´
ıguez-Qui
˜
nonez, J. C., Flores-Fuentes,
W., Zhirabok, A., Alaniz-Plata, R., N
´
u
˜
nez-L
´
opez,
J. A., Andrade-Collazo, H., Miranda-Vega, J. E., and
Murrieta-Rico, F. N. (2024). Mathematical Modeling
for Robot 3D Laser Scanning in Complete Darkness
Environments to Advance Pipeline Inspection.
Summan, R., Jackson, W., Dobie, G., MacLeod, C., Mi-
neo, C., West, G., Offin, D., Bolton, G., Marshall,
S., and Lille, A. (2018). A novel visual pipework
inspection system. AIP Conference Proceedings,
1949(1):220001.
Tian, T., Wang, L., Yan, X., Ruan, F., Aadityaa, G. J.,
Choset, H., and Li, L. (2023). Visual-Inertial-Laser-
Lidar (VILL) SLAM: Real-Time Dense RGB-D Map-
ping for Pipe Environments. In 2023 IEEE/RSJ In-
ternational Conference on Intelligent Robots and Sys-
tems (IROS), pages 1525–1531. IEEE.
Zhao, M., Fang, Z., Ding, N., Li, N., Su, T., and Qian, H.
(2023). Quantitative Detection Technology for Geo-
metric Deformation of Pipelines Based on LiDAR.
Zhao, W., Zhang, L., and Kim, J. (2020). Design and analy-
sis of independently adjustable large in-pipe robot for
long-distance pipeline. Applied Sciences, 10(10).
GISTAM 2025 - 11th International Conference on Geographical Information Systems Theory, Applications and Management
202