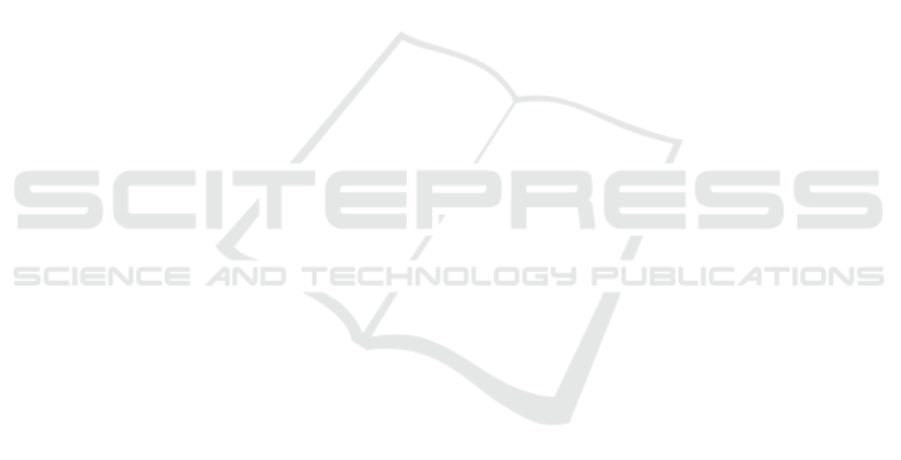
dra, JSON files, or CSV files, new DBMSs and data
formats can be easily added using wrappers.
Finally, Table 2 illustrates the time required for
user interactions across scenarios A, B, and C de-
picted in Figs. 3, 4, and 5 when processing the
Yelp dataset, comparing the conventional manual ap-
proach versus using the proposed framework tool.
The framework streamlines the workflow by automat-
ing every step of the process. In Step 1, it infers the
schema from the data. Following this, the framework
facilitates editing the schema in Step 2 by providing a
user-friendly interface that allows users to make nec-
essary adjustments with minimal effort. In Step 3,
it supports creating custom mappings between differ-
ent data models. It then moves on to generate multi-
model data in Step 4. Finally, it translates queries to
operate across different data models in Step 5. The
results demonstrate a substantial reduction in the time
required for each step when using the framework,
highlighting its efficiency and effectiveness in reduc-
ing user input and eventual errors.
5 CONCLUSION
This paper proposes a solution to the problem of lack
of real-world multi-model data (and the respective
queries). We use a different approach instead of the
common strategy of generating a synthetic dataset de-
spite having numerous realistic features. Using a spe-
cific utilization of our previously created toolset, we
introduce the idea of a transformation framework that
can transform a given, preferably real-world, dataset
into a preferred multi-model dataset. Using a well-
known dataset, Yelp, we demonstrate the advantages
and applicability of the idea.
Our future work will focus primarily on imple-
menting a common interface that will cover the whole
functionality of the proposed framework and simplify
the integration of the tools. In addition, we want to
focus on the simulation of the evolution of the re-
sulting datasets, either through user specification or
through the detection of changes in the input single-
model data or operations. Lastly, we want to create
a repository of the resulting multi-model datasets to
provide a robust source of test cases to be immediately
used. We also want to perform extensive experiments
with the datasets to provide unbiased benchmarking
results for elected multi-model databases.
ACKNOWLEDGMENT
This work was supported by the GA
ˇ
CR grant no. 23-
07781S and GAUK grant no. 292323.
REFERENCES
Belloni, S., Ritter, D., Schr
¨
oder, M., and R
¨
orup, N. (2022).
DeepBench: Benchmarking JSON Document Stores.
In Proceedings of the 2022 Workshop on 9th Interna-
tional Workshop of Testing Database Systems, DBTest
’22, page 1–9, New York, NY, USA. Association for
Computing Machinery.
Bondiombouy, C. and Valduriez, P. (2016). Query process-
ing in multistore systems: an overview. Int. J. Cloud
Comput., 5(4):309–346.
Bonifati, A., Holubov
´
a, I., Prat-P
´
erez, A., and Sakr, S.
(2020). Graph Generators: State of the Art and Open
Challenges. ACM Comput. Surv., 53(2).
Feinberg, D., Adrian, M., Heudecker, N., Ronthal, A. M.,
and Palanca, T. (12 October 2015). Gartner Magic
Quadrant for Operational Database Management Sys-
tems, 12 October 2015.
Ghazal, A., Rabl, T., Hu, M., Raab, F., Poess, M., Crolotte,
A., and Jacobsen, H.-A. (2013). BigBench: towards
an industry standard benchmark for big data analytics.
In Proceedings of the 2013 ACM SIGMOD Interna-
tional Conference on Management of Data, SIGMOD
’13, page 1197–1208, New York, NY, USA. Associa-
tion for Computing Machinery.
International, E. (2013). JavaScript Object Notation
(JSON). http://www.JSON.org/.
Kim, B., Koo, K., Enkhbat, U., Kim, S., Kim, J., and Moon,
B. (2022). M2Bench: A Database Benchmark for
Multi-Model Analytic Workloads. Proc. VLDB En-
dow., 16(4):747–759.
Koupil, P., B
´
art
´
ık, J., and Holubov
´
a, I. (2022a). MM-
evocat: A Tool for Modelling and Evolution Manage-
ment of Multi-Model Data. In Proc. of CIKM ’22,
CIKM ’22, pages 4892–4896, New York, NY, USA.
ACM.
Koupil, P., B
´
art
´
ık, J., and Holubov
´
a, I. (2024). MM-
evoquee: Query Synchronisation in Multi-Model
Databases. In Proc. of EDBT ’24, pages 818–821.
OpenProceedings.org.
Koupil, P., Crha, D., and Holubov
´
a, I. (2023). A Universal
Approach for Simplified Redundancy-Aware Cross-
Model Querying. Available at SSRN 4596127.
Koupil, P. and Holubov
´
a, I. (2022). A unified represen-
tation and transformation of multi-model data using
category theory. J. Big Data, 9(1):61.
Koupil, P., Hricko, S., and Holubov
´
a, I. (2022b). MM-
infer: A Tool for Inference of Multi-Model Schemas.
In Proceedings of the 25th International Conference
on Extending Database Technology, EDBT 2022, Ed-
inburgh, UK, March 29 - April 1, 2022, pages 2:566–
2:569. OpenProceedings.org.
Reshaping Reality: Creating Multi-Model Data and Queries from Real-World Inputs
183