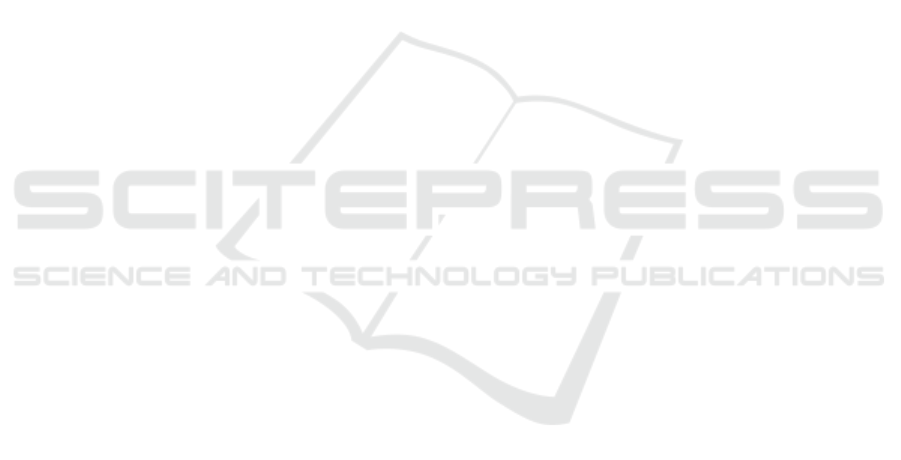
ternational Conference on Software Maintenance and
Evolution (ICSME), pages 481–490.
Huang, D., Bu, Q., Zhang, J. M., Luck, M., and Cui, H.
(2023). Agentcoder: Multi-agent-based code gener-
ation with iterative testing and optimisation. arXiv
preprint arXiv:2312.13010.
Kaplan, J., McCandlish, S., Henighan, T., Brown, T. B.,
Chess, B., Child, R., Gray, S., Radford, A., Wu, J., and
Amodei, D. (2020). Scaling laws for neural language
models. arXiv preprint arXiv:2001.08361.
Koschuetzki, T. (2008). Extra hot: CakePHP 1.2 stable
is finally released! http://debuggable.com/posts/
extra-hot-cakephp-1.2-stable-is-finally-released!:
4954151c-f87c-434b-abbd-4e404834cda3. Ac-
cessed: Oct.30, 2024.
Liu, N. F., Lin, K., Hewitt, J., Paranjape, A., Bevilacqua,
M., Petroni, F., and Liang, P. (2024a). Lost in the mid-
dle: How language models use long contexts. Trans-
actions of the Association for Computational Linguis-
tics, 12:157–173.
Liu, Y., Le-Cong, T., Widyasari, R., Tantithamthavorn, C.,
Li, L., Le, X.-B. D., and Lo, D. (2024b). Refining
chatgpt-generated code: Characterizing and mitigat-
ing code quality issues. ACM Transactions on Soft-
ware Engineering and Methodology, 33(5):1–26.
Madaan, A., Tandon, N., Gupta, P., Hallinan, S., Gao, L.,
Wiegreffe, S., Alon, U., Dziri, N., Prabhumoye, S.,
Yang, Y., et al. (2024). Self-refine: Iterative refine-
ment with self-feedback. Advances in Neural Infor-
mation Processing Systems, 36.
Norri, J., Junkkari, M., and Poranen, T. (2020). Digitization
of data for a historical medical dictionary. Language
Resources and Evaluation, 54(3):615–643.
OpenAI (2024a). GPT-4o mini: advancing cost-
efficient intelligence. https://openai.com/index/
gpt-4o-mini-advancing-cost-efficient-intelligence/.
Accessed: Sep.3, 2024.
OpenAI (2024b). Introducing OpenAI o1-preview. https:
//openai.com/index/introducing-openai-o1-preview/.
Accessed: Sep.19, 2024.
Ou
´
edraogo, W. C., Kabor
´
e, K., Tian, H., Song, Y.,
Koyuncu, A., Klein, J., Lo, D., and Bissyand
´
e, T. F.
(2024). Large-scale, independent and comprehensive
study of the power of llms for test case generation.
arXiv preprint arXiv:2407.00225.
Pan, R., Ibrahimzada, A. R., Krishna, R., Sankar, D., Wassi,
L. P., Merler, M., Sobolev, B., Pavuluri, R., Sinha,
S., and Jabbarvand, R. (2024). Lost in translation:
A study of bugs introduced by large language mod-
els while translating code. In Proceedings of the
IEEE/ACM 46th International Conference on Soft-
ware Engineering, pages 1–13.
Radford, A. and Narasimhan, K. (2018). Improving lan-
guage understanding by generative pre-training.
Rasheed, Z., Sami, M. A., Kemell, K.-K., Waseem,
M., Saari, M., Syst
¨
a, K., and Abrahamsson, P.
(2024a). Codepori: Large-scale system for au-
tonomous software development using multi-agent
technology. arXiv preprint arXiv:2402.01411.
Rasheed, Z., Sami, M. A., Rasku, J., Kemell, K.-K., Zhang,
Z., Harjamaki, J., Siddeeq, S., Lahti, S., Herda, T.,
Nurminen, M., et al. (2024b). Timeless: A vision for
the next generation of software development. arXiv
preprint arXiv:2411.08507.
Rasheed, Z., Sami, M. A., Waseem, M., Kemell, K.-K.,
Wang, X., Nguyen, A., Syst
¨
a, K., and Abrahamsson,
P. (2024c). Ai-powered code review with llms: Early
results. arXiv preprint arXiv:2404.18496.
Rasheed, Z., Waseem, M., Kemell, K.-K., Xiaofeng, W.,
Duc, A. N., Syst
¨
a, K., and Abrahamsson, P. (2023).
Autonomous agents in software development: A vi-
sion paper. arXiv preprint arXiv:2311.18440.
Rasheed, Z., Waseem, M., Syst
¨
a, K., and Abrahamsson, P.
(2024d). Large language model evaluation via multi
AI agents: Preliminary results. In ICLR 2024 Work-
shop on Large Language Model (LLM) Agents.
Sami, M. A., Waseem, M., Zhang, Z., Rasheed, Z., Syst
¨
a,
K., and Abrahamsson, P. (2024). Early results of an
ai multiagent system for requirements elicitation and
analysis. In International Conference on Product-
Focused Software Process Improvement, pages 307–
316. Springer.
Sami, M. A., Waseem, M., Zhang, Z., Rasheed, Z.,
Syst
¨
a, K., and Abrahamsson, P. (2025). Early re-
sults of an ai multiagent system for requirements elic-
itation and analysis. In Pfahl, D., Gonzalez Huerta,
J., Kl
¨
under, J., and Anwar, H., editors, Product-
Focused Software Process Improvement, pages 307–
316, Cham. Springer Nature Switzerland.
Shinn, N., Cassano, F., Gopinath, A., Narasimhan, K., and
Yao, S. (2024). Reflexion: Language agents with ver-
bal reinforcement learning. Advances in Neural Infor-
mation Processing Systems, 36.
Smyth, S. (2023). Penetration testing and legacy systems.
arXiv preprint arXiv:2402.10217.
Sommerville, I. (2016). Software engineering. Always
learning. Pearson, tenth edition edition.
Tampere University and Rasheed, Z. (2025). Autonomous
legacy web application upgrades using a multi-agent
system. https://doi.org/10.5281/zenodo.14858713.
Vaswani, A. (2017). Attention is all you need. Advances in
Neural Information Processing Systems.
Vesi
´
c, S. and Lakovi
´
c, D. (2023). A framework for evalu-
ating legacy systems – a case study. Kultura polisa,
20(1):32–50.
Zamfirescu-Pereira, J., Wong, R. Y., Hartmann, B., and
Yang, Q. (2023). Why johnny can’t prompt: How non-
AI experts try (and fail) to design LLM prompts. In
Proceedings of the 2023 CHI Conference on Human
Factors in Computing Systems, pages 1–21. ACM.
Zhong, L., Wang, Z., and Shang, J. (2024). Ldb: A large
language model debugger via verifying runtime exe-
cution step-by-step. arXiv preprint arXiv:2402.16906.
ENASE 2025 - 20th International Conference on Evaluation of Novel Approaches to Software Engineering
196