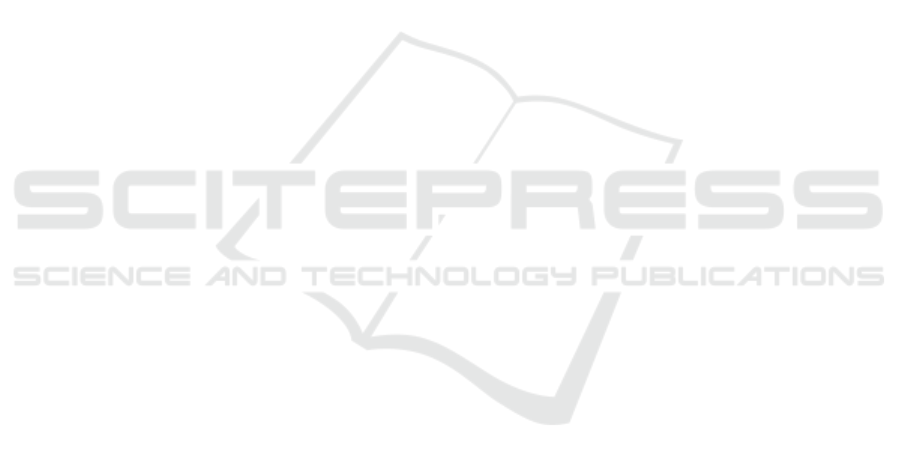
Despite these advancements, several challenges
remain. The implementation of QTNs on near-term
quantum devices requires further optimization to ad-
dress hardware constraints, such as limited qubit co-
herence and noise. Additionally, more systematic
benchmarking against classical and QML approaches
is needed to fully understand the trade-offs and ad-
vantages of QTNs.
In conclusion, the incorporation of QTNs into
QML has the potential to drive significant advance-
ments in computational efficiency, generalization, and
data handling. Continued development and explo-
ration of QTNs will not only advance the field of
quantum machine learning but also contribute to the
broader landscape of computational science, paving
the way for transformative quantum technologies.
ACKNOWLEDGEMENTS
This work has been supported by CESAR - Centro de
Estudos e Sistemas Avançados do Recife - and CE-
SAR School.
REFERENCES
Adhikary, S., Srinivasan, S., Miller, J., Rabusseau, G., and
Boots, B. (2021). Quantum tensor networks, stochas-
tic processes, and weighted automata. Proceedings of
the 24th International Conference on Artificial Intelli-
gence and Statistics (AISTATS), 130:2080–2088.
Ali, M. and Kabel, M. (2024). Piecewise polynomial tensor
network quantum feature encoding. arXiv (Cornell
University).
Araz, J. Y. and Spannowsky, M. (2022). Classical versus
quantum: Comparing tensor-network-based quantum
circuits on large hadron collider data. Physical Review
A, 106(6).
Azad, F. (2024). Tensor networks for classical and quan-
tum simulation of open and closed quantum systems.
PhD thesis, University Coll. London, University Coll.
London.
Bañuls, M. C. (2023). Tensor network algorithms: A route
map. Annual Review of Condensed Matter Physics,
14(1):173–191.
Bernardi, A., Lazzari, C. D., and Gesmundo, F. (2022). Di-
mension of tensor network varieties. Communications
in Contemporary Mathematics, 25(10).
Bhatia, A. S. and Kumar, A. (2018). Quantifying ma-
trix product state. Quantum Information Processing,
17(3).
Biamonte, J. and Bergholm, V. (2017a). Quantum Tensor
Networks in a Nutshell.
Biamonte, J. and Bergholm, V. (2017b). Tensor networks in
a nutshell. arXiv (Cornell University).
Bridgeman, J. C. and Chubb, C. T. (2016). Hand-waving
and interpretive dance: an introductory course on ten-
sor networks. Journal of Physics A: Mathematical and
Theoretical, 50(22):223001–223001.
Cores, C. F., Kristjuhan, K., and Jones, M. N. (2024). A
joint optimization approach of parameterized quan-
tum circuits with a tensor network.
Guala, D., Zhang, S., Cruz, E., Riofrío, C. A., Klepsch,
J., and Arrazola, J. M. (2023). Practical overview of
image classification with tensor-network quantum cir-
cuits. Scientific Reports, 13(1):4427.
Hou, Y.-Y., Li, J., Xu, T., and Liu, X.-Y. (2024). A
hybrid quantum-classical classification modelbased
on branching multi-scale entanglementrenormaliza-
tion ansatz. Research Square (Research Square).
Huang, H. Y., Broughton, M., and Mohseni, M. e. a.
(2021a). Power of data in quantum machine learning.
Nature Communications, 12:2631.
Huang, R., Tan, X., and Xu, Q. (2021b). Variational
quantum tensor networks classifiers. Neurocomput-
ing, 452:89–98.
Huggins, W., Patil, P., Mitchell, B., Whaley, K. B., and
Stoudenmire, E. M. (2019). Towards quantum ma-
chine learning with tensor networks. Quantum Sci-
ence and Technology, 4(2):024001.
Kardashin, A., Uvarov, A., and Biamonte, J. (2021). Quan-
tum machine learning tensor network states. Frontiers
in Physics, 8.
Liu, X.-Y., Zhao, Q., Biamonte, J., and Walid, A. (2021).
Tensor, tensor networks, quantum tensor networks in
machine learning: An hourglass architecture.
Lykov, D. (2024). Large-scale tensor network quantum al-
gorithm simulator. Knowledge UChicago.
Manjunath, T. C., Venkatesan, M., and Prabhavathy, P.
(2023). Research oriented reviewing of quantum ma-
chine learning. International Research Journal on Ad-
vanced Science Hub, 5(Issue 05S):165–177.
Orús, R. (2014). A practical introduction to tensor net-
works: Matrix product states and projected entan-
gled pair states. Annals of Physics, 349(0003-
4916):117–158.
Orús, R. (2019). Tensor networks for complex quantum
systems. Nature Reviews Physics, 1(9):538–550.
Quantinuum (2024).
Rieser, H.-M., Köster, F., and Raulf, A. P. (2023). Tensor
networks for quantum machine learning. Proceedings
of the Royal Society A: Mathematical, Physical and
Engineering Sciences, 479(2275):20230218.
Sengupta, R., Adhikary, S., Oseledets, I., and Biamonte, J.
(2022). Tensor networks in machine learning.
Tychola, K. A., Kalampokas, T., and Papakostas, G. A.
(2023). Quantum machine learning—an overview.
Electronics, 12(11):2379.
Xiao, Y. and Watson, M. (2019). Guidance on conducting
a systematic literature review. Journal of Planning
Education and Research, 39(1):93–112.
QAIO 2025 - Workshop on Quantum Artificial Intelligence and Optimization 2025
854