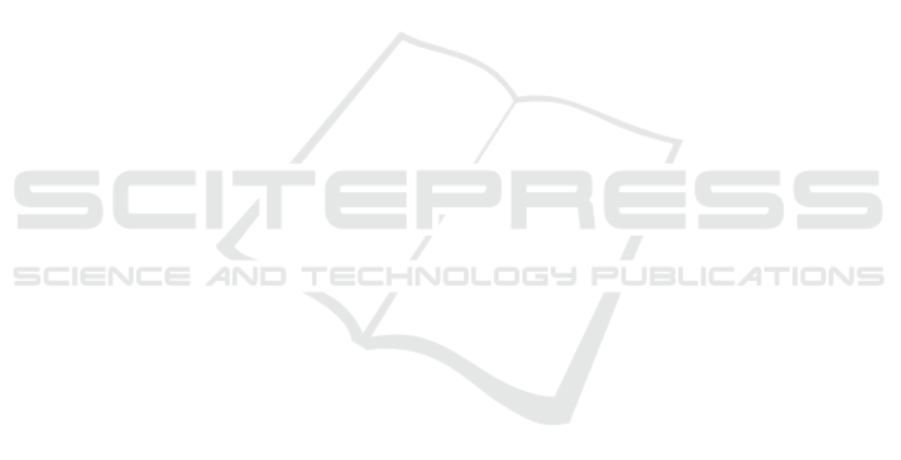
early screening of mental health conditions, advancing
eHealth solutions for mental health assessments.
ACKNOWLEDGMENTS
Work on this study was partially funded by VwV Invest
BW – Innovation II funding program, with project
number BW1 4056/03.
REFERENCES
Ak
c¸
ay, M. B. and O
˘
guz, K. (2020). Speech emotion recog-
nition: Emotional models, databases, features, prepro-
cessing methods, supporting modalities, and classifiers.
Speech Communication, 116:56–76.
Aloshban, N., Esposito, A., and Vinciarelli, A. (2022). What
you say or how you say it? depression detection
through joint modeling of linguistic and acoustic as-
pects of speech. Cognitive Computation, 14(5):1585–
1598.
Anusha, R., Subhashini, P., Jyothi, D., Harshitha, P., Sushma,
J., and Mukesh, N. (2021). Speech emotion recognition
using machine learning. In 2021 5th international
conference on trends in electronics and informatics
(ICOEI), pages 1608–1612. IEEE.
APA (2022). Diagnostic and Statistical Manual of Mental
Disorders (DSMV). American Psychiatric Association,
5th edition.
Atmaja, B. T. and Sasou, A. (2022). Evaluating self-
supervised speech representations for speech emotion
recognition. IEEE Access, 10:124396–124407.
Baevski, A., Zhou, Y., Mohamed, A., and Auli, M. (2020).
wav2vec 2.0: A framework for self-supervised learning
of speech representations. Advances in neural informa-
tion processing systems, 33:12449–12460.
Boehnlein, J., Altegoer, L., Muck, N. K., Roesmann, K.,
Redlich, R., Dannlowski, U., and Leehr, E. J. (2020).
Factors influencing the success of exposure therapy for
specific phobia: A systematic review. Neuroscience &
Biobehavioral Reviews, 108:796–820.
Bredin, H. (2023). pyannote. audio 2.1 speaker diarization
pipeline: principle, benchmark, and recipe. In 24th
INTERSPEECH Conference (INTERSPEECH 2023),
pages 1983–1987. ISCA.
Bundespsychotherapeutenkammer (BPtK) (2023). Keine
vorschnelle einf
¨
uhrung von ki-anwendungen! Resolu-
tion verabschiedet vom 42. Deutscher Psychotherapeu-
tentag, 5./6. Mai 2023, Frankfurt.
Cummins, N., Matcham, F., Klapper, J., and Schuller, B.
(2020). Artificial intelligence to aid the detection of
mood disorders. In Artificial Intelligence in Precision
Health, pages 231–255. Elsevier.
Danner, M., Had
ˇ
zi
´
c, B., Gerhardt, S., Ludwig, S., Uslu,
I., Shao, P., Weber, T., Shiban, Y., and Ratsch, M.
(2023). Advancing mental health diagnostics: Gpt-
based method for depression detection. In 2023 62nd
Annual Conference of the Society of Instrument and
Control Engineers (SICE), pages 1290–1296. IEEE.
European Parliament and Council of the European Union
(2024). Laying down harmonised rules on artificial
intelligence (artificial intelligence act) and amending
certain union legislative acts.
Fehr, J., Citro, B., Malpani, R., Lippert, C., and Madai,
V. I. (2024). A trustworthy ai reality-check: the lack
of transparency of artificial intelligence products in
healthcare. Frontiers in Digital Health, 6:1267290.
Hadzic, B., Mohammed, P., Danner, M., Ohse, J., Zhang, Y.,
Shiban, Y., and R
¨
atsch, M. (2024a). Enhancing early
depression detection with ai: a comparative use of nlp
models. SICE journal of control, measurement, and
system integration, 17(1):135–143.
Hadzic, B., Ohse, J., Danner, M., Peperkorn, N. L., Mo-
hammed, P., Shiban, Y., and R
¨
atsch, M. (2024b). Ai-
supported diagnostic of depression using clinical in-
terviews: A pilot study. In VISIGRAPP (1): GRAPP,
HUCAPP, IVAPP, pages 500–507.
Hyde, J., Ryan, K. M., and Waters, A. M. (2019). Psy-
chophysiological markers of fear and anxiety. Current
Psychiatry Reports, 21:1–10.
Islam, M. S., Kabir, M. N., Ghani, N. A., Zamli, K. Z.,
Zulkifli, N. S. A., Rahman, M. M., and Moni, M. A.
(2024). Challenges and future in deep learning for
sentiment analysis: a comprehensive review and a pro-
posed novel hybrid approach. Artificial Intelligence
Review, 57(3):62.
Joormann, J. and Stanton, C. H. (2016). Examining emotion
regulation in depression: A review and future direc-
tions. Behaviour research and therapy, 86:35–49.
Kirschbaum, C., Pirke, K.-M., and Hellhammer, D. H.
(1993). The ‘trier social stress test’–a tool for investi-
gating psychobiological stress responses in a laboratory
setting. Neuropsychobiology, 28(1-2):76–81.
Kroenke, K., Strine, T. W., Spitzer, R. L., Williams, J. B.,
Berry, J. T., and Mokdad, A. H. (2009). The phq-8 as a
measure of current depression in the general population.
Journal of affective disorders, 114(1-3):163–173.
Ma, Z., Chen, M., Zhang, H., Zheng, Z., Chen, W., Li, X., Ye,
J., Chen, X., and Hain, T. (2024). Emobox: Multilin-
gual multi-corpus speech emotion recognition toolkit
and benchmark. arXiv preprint arXiv:2406.07162.
Ma, Z., Zheng, Z., Ye, J., Li, J., Gao, Z., Zhang, S., and Chen,
X. (2023). emotion2vec: Self-supervised pre-training
for speech emotion representation. arXiv preprint
arXiv:2312.15185.
Mohammed, P., Had
ˇ
zi
´
c, B., Alkostantini, M. E., Kubota, N.,
Shiban, Y., and R
¨
atsch, M. (2024). Hearing emotions:
Fine-tuning speech emotion recognition models. In
Proceedings of the 5th Symposium on Pattern Recogni-
tion and Applications (SPRA 2024).
Mustafa, M. B., Yusoof, M. A., Don, Z. M., and Malekzadeh,
M. (2018). Speech emotion recognition research: an
analysis of research focus. International Journal of
Speech Technology, 21:137–156.
Ohse, J., Had
ˇ
zi
´
c, B., Mohammed, P., Peperkorn, N., Danner,
M., Yorita, A., Kubota, N., R
¨
atsch, M., and Shiban, Y.
ICT4AWE 2025 - 11th International Conference on Information and Communication Technologies for Ageing Well and e-Health
112