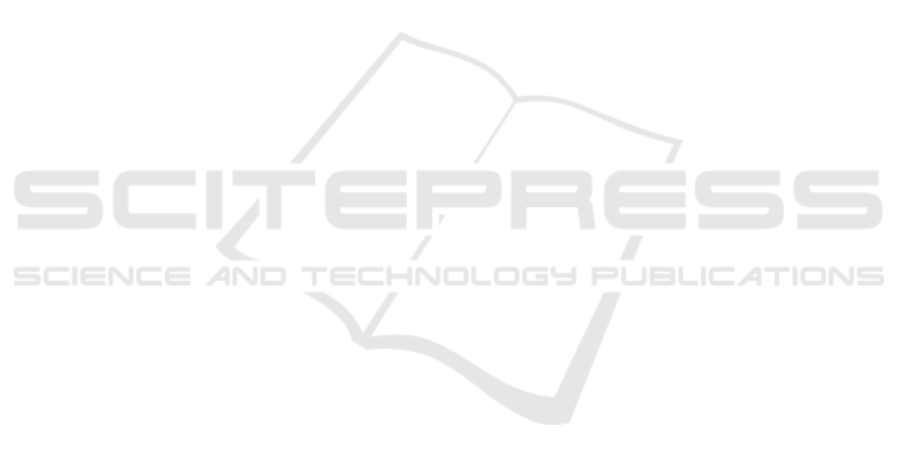
(FAPESP); Silvia Regina Vergilio is supported by
grant #310034/2022-1, CNPq. This work was also
supported by Coordination for the Improvement of
Higher Education Personnel (CAPES) - Program of
Academic Excellence (PROEX).
REFERENCES
Bernhard, L., Scharnowski, T., Schl
¨
ogel, M., Blazytko, T.,
and Holz, T. (2022). JIT-Picking: Differential fuzzing
of JavaScript engines. In ACM Conference on Com-
puter and Communications Security CCS, pages 351–
364. ACM.
Eom, J., Jeong, S., and Kwon, T. (2024). Fuzzing
JavaScript interpreters with coverage-guided rein-
forcement learning for LLM-based mutation. In Pro-
ceedings of the 33rd ACM SIGSOFT International
Symposium on Software Testing and Analysis (ISSTA
’24), pages 1–13, New York, NY, USA. ACM.
Fagin, R. and Wimmers, E. L. (2000). A formula for incor-
porating weights into scoring rules. Theoretical Com-
puter Science, 239(2):309–338.
Groß, S., Koch, S., Bernhard, L., Holz, T., and Johns, M.
(2023). Fuzilli: Fuzzing for JavaScript JIT compiler
vulnerabilities. In Network and Distributed Systems
Security (NDSS) Symposium 2023, pages 10–25, San
Diego, CA, USA.
Han, H., Oh, D., and Cha, S. (2019). CodeAlchemist:
Semantics-aware code generation to find vulnerabili-
ties in JavaScript engines. In Network and Distributed
System Security Symposium.
He, X., Xie, X., Li, Y., Sun, J., Li, F., Zou, W., Liu,
Y., Yu, L., Zhou, J., Shi, W., et al. (2021). Sofi:
Reflection-augmented fuzzing for JavaScript engines.
In Proceedings of the 2021 ACM SIGSAC Conference
on Computer and Communications Security, pages
2229–2242.
Holler, C., Herzig, K., and Zeller, A. (2012). Fuzzing with
code fragments. In 21st USENIX Security Symposium
(USENIX Security 12), pages 445–458, Bellevue, WA.
USENIX Association.
Kang, Z. (2021). A review on JavaScript engine vulnerabil-
ity mining. In Journal of Physics: Conference Series,
volume 1744, page 042197. IOP Publishing.
Kienle, H. M. (2010). It’s about time to take JavaScript
(more) seriously. IEEE Software, 27(3):60–62.
Lee, S., Han, H., Cha, S. K., and Son, S. (2020). Montage:
A neural network language Model-Guided JavaScript
engine fuzzer. In 29th USENIX Security Symposium
(USENIX Security 20), pages 2613–2630. USENIX
Association.
Li, F. and Paxson, V. (2017). A large-scale empirical study
of security patches. In Proceedings of the 2017 ACM
SIGSAC Conference on Computer and Communica-
tions Security, CCS ’17, page 2201–2215, New York,
NY, USA. Association for Computing Machinery.
Mozilla (2022). jsfunfuzz. https://github.com/
MozillaSecurity/funfuzz. Accessed in 08/24/2022.
Oliveira, B. G., Endo, A. T., and Vergilio, S. (2023).
Characterizing security-related commits of JavaScript
engines. In In Proceedings of the 25th Interna-
tional Conference on Enterprise Information Systems
(ICEIS), volume 2, pages 86–97.
Park, S., Xu, W., Yun, I., Jang, D., and Kim, T. (2020).
Fuzzing JavaScript engines with aspect-preserving
mutation. In 2020 IEEE Symposium on Security and
Privacy (SP), pages 1629–1642.
Shin, Y. and Williams, L. (2008). Is complexity really the
enemy of software security? In Proceedings of the
4th ACM Workshop on Quality of Protection, QoP
’08, page 47–50, New York, NY, USA. Association
for Computing Machinery.
Sun, L., Wu, C., Wang, Z., Kang, Y., and Tang, B. (2022).
KOP-Fuzzer: A key-operation-based fuzzer for type
confusion bugs in JavaScript engines. In 2022 IEEE
46th Annual Computers, Software, and Applications
Conference (COMPSAC), pages 757–766.
Tian, Y., Qin, X., and Gan, S. (2021). Research on fuzzing
technology for JavaScript Engines. In Proceedings
of the 5th International Conference on Computer Sci-
ence and Application Engineering, pages 1–7.
Wang, J., Chen, B., Wei, L., and Liu, Y. (2019). Superion:
Grammar-aware greybox fuzzing. In 2019 IEEE/ACM
41st International Conference on Software Engineer-
ing (ICSE), pages 724–735.
Wang, J., Zhang, Z., Xin, Q. A., Liu, S., Du, X., and
Chen, J. (2023). FuzzJIT: Oracle-Enhanced fuzzing
for JavaScript engine JIT compiler. In 32nd USENIX
Security Symposium (USENIX Security 23), Anaheim,
CA. USENIX Association.
Xiang, Y., Zhang, X., Liu, P., Ji, S., Liang, H., Xu, J., and
Wang, W. (2024). Critical code guided directed grey-
box fuzzing for commits. In 33rd USENIX Security
Symposium (USENIX Security 24), pages 2459–2474,
Philadelphia, PA. USENIX Association.
Zhu, X. and B
¨
ohme, M. (2021). Regression greybox
fuzzing. In Proceedings of the 2021 ACM SIGSAC
Conference on Computer and Communications Secu-
rity, CCS ’21, page 2169–2182, New York, NY, USA.
Association for Computing Machinery.
ICEIS 2025 - 27th International Conference on Enterprise Information Systems
70