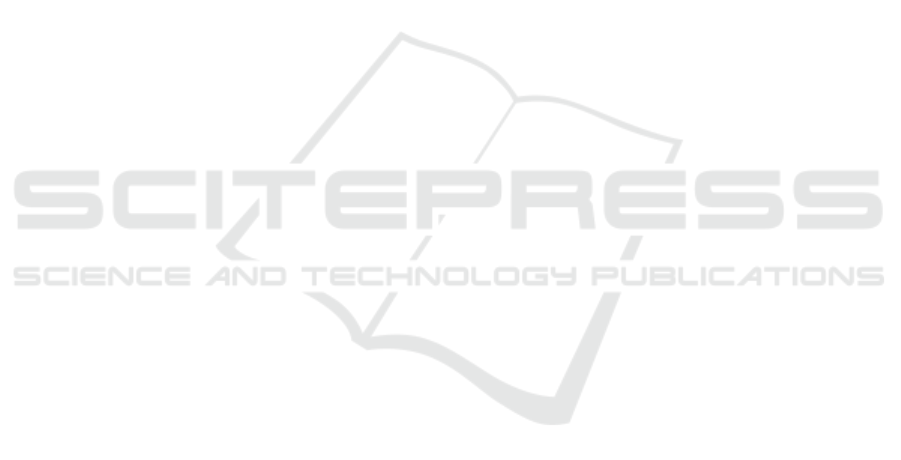
ACKNOWLEDGEMENTS
This work was supported in part by the Coordenac¸
˜
ao
de Aperfeic¸oamento de Pessoal de N
´
ıvel Superior -
Brasil (CAPES) - Finance Code 001, Conselho Na-
cional de Desenvolvimento e Pesquisa (CNPq) un-
der Grant 140254/2021-8, and Fundac¸
˜
ao de Amparo
`
a Pesquisa do Rio de Janeiro (FAPERJ)
REFERENCES
Ahmada, F., Ansaria, M. Z., Anwara, R., Shahzada, B., and
Ikrama, A. (2023). Deep learning based classification
of underwater acoustic signals. In International Con-
ference on Machine Learning and Data Engineering
(ICMLDE 2023), pages 1115–1124. Elsevier.
Ahmada, F., Ansaria, M. Z., Anwara, R., Shahzada, B., and
Ikrama, A. (2024). Spectral analysis of the underwa-
ter acoustic noise radiated by ships with controllable
pitch propellers. Ocean Engineering, 115112(299):1–
14.
Belghith, E. H., Rioult, F., and Bouzidi, M. (2018). Acous-
tic diversity classifier for automated marine big data
analysis. In IEEE 30th International Conference
on Tools with Artificial Intelligence, pages 130–136.
IEEE.
Chalmers, C., Fergus, P., Wich, S., and Longmore, S. N.
(2021). Modelling animal biodiversity using acous-
tic monitoring and deep learning. Proceedings of the
IEEE International Workshop.
Dong, Y., Shen, X., Yan, Y., and Wang, H. (2022). Small-
scale data underwater acoustic target recognition with
deep forest model. In IEEE International Conference
on Signal Processing, Communications and Comput-
ing (ICSPCC). IEEE.
F. Traverso, T. G., Rizzuto, E., and A.Trucco (2024). Under-
water noise characterization of a typical fishing vessel
from atlantic canada. Ocean Engineering, pages 1–6.
Gencoglu, O., Virtanen, T., and Huttunen, H. Recognition
of acoustic events using deep neural networks. pages
1–5.
Hamard, Q., Pham, M.-T., Cazau, D., and Heerah, K.
(2024). A deep learning model for detecting and clas-
sifying multiple marine mammal species from passive
acoustic data. Ecological Informatics, 115112(84):1–
19.
He, J., Zhang, B., Liu, P., Li, X., Wang, L., and Tang, R.
(2024). Effective underwater acoustic target passive
localization of using a multi-task learning model with
attention mechanism: Analysis and comparison un-
der real sea trial datasets. Applied Ocean Research,
115112(84):1–19.
Kuang, Y., Wub, Q., Wangc, Y., Dey, N., Shi, F., Crespo,
R. G., and Sherratt, R. S. (2020). Simplified inverse
filter tracked affective acoustic signals classification
incorporating deep convolutional neural networks.
Applied Soft Computing Journal, 115112(97):1–16.
Kuang, Y., Wub, Q., Wangc, Y., Dey, N., Shi, F., Cre-
spo, R. G., and Sherratt, R. S. (2024). Simplified
inverse filter tracked affective acoustic signals classi-
fication incorporating deep convolutional neural net-
works. Ecological Informatics, 115112(84):1–16.
Liang, L.-P., Zhang, J., Xu, K.-J., Ye, G.-Y., Yang, S.-L.,
and Yu, X.-L. (2024). Classification modeling of valve
internal leakage acoustic emission signals based on
optimal wavelet scattering coefficients. Measurement,
115112(236):1–15.
Marinati, R., Coelho, R., and Z
˜
ao, L. (2024). Frs: Adap-
tive score for improving acoustic source classifica-
tion from noisy signals. IEEE SIGNAL PROCESSING
LETTERS, 115112(31):1–5.
Marquesy, T. P., Rezvanifary, A., Cotey, M., Albuy, A. B.,
Ersahinz, K., Mudgez, T., and Gauthierx, S. (2019).
Segmentation, classification, and visualization of orca
calls using deep learning. ICASSP, 115112(84):8231–
8235.
Mathiasa, S. G., Akmala, M. U., and Saara Asifa,
Leonid Kovala, S. K. D. G. (2024). Pattern identifi-
cations in transformed acoustic signals using classifi-
cation models. In Anais da Confer
ˆ
encia, pages 93–99.
Elsevier.
Pala, A., Oleynik, A., Malde, K., and Handegard, N. O.
Recognition of acoustic events using deep neural net-
works. pages 1–14.
Safaei, M., Soleymani, S. A., Safaei, M., Chizari, H., and
Nilashi, M. (2024). Deep learning algorithm for su-
pervision process in production using acoustic signal.
Ecological Informatics, 115112(84):1–16.
Wei, Z., Ju, Y., and Song, M. (2023). A method of under-
water acoustic signal classification based on deep neu-
ral network. In International Conference on Machine
Learning and Data Engineering (ICMLDE 2023),
pages 1115–1124. Elsevier.
Improving Underwater Ship Sound Classification with CNNs and Advanced Signal Processing
561