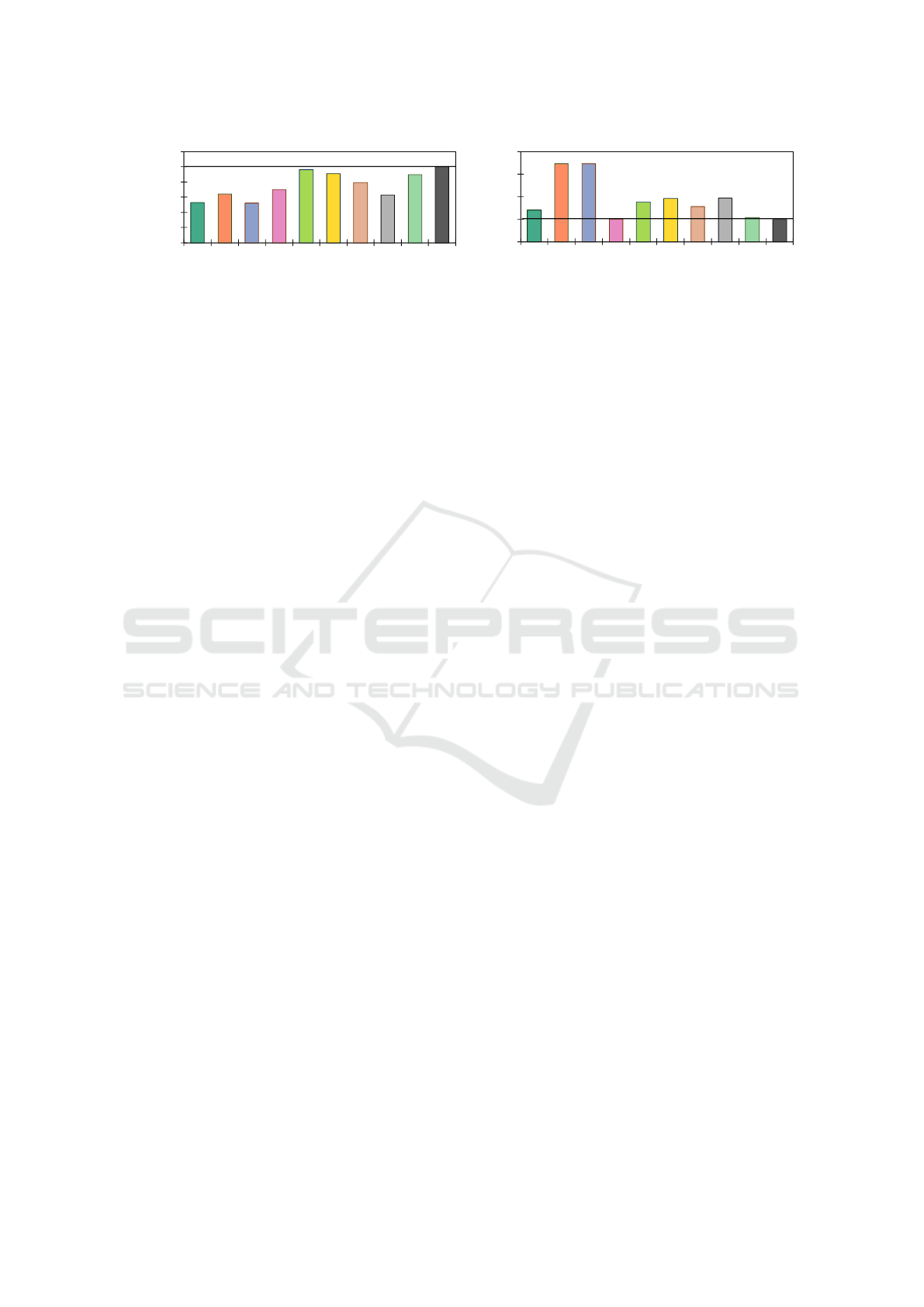
0.0
0.2
0.4
0.6
0.8
1.0
1.2
STD-WN16
WTD-WN24
STD-WN64
Best-WN16
Best-WN24
Best-WN64
Kube-Sched.
Random
SmartNodeTuner
Best-All
Perf. Normalized
(a) Performance
0.0
1.0
2.0
3.0
4.0
STD-WN16
WTD-WN24
STD-WN64
Best-WN16
Best-WN24
Best-WN64
Kube-Sched.
Random
SmartNodeTuner
Best-All
Energy normalized
(b) Energy consumption
Figure 8: Performance and Energy results for each strategy normalized to Best-All.
REFERENCES
Bailey, D. H., Barszcz, E., Barton, J. T., Browning, D. S.,
Carter, R. L., Dagum, L., Fatoohi, R. A., Frederick-
son, P. O., Lasinski, T. A., Schreiber, R. S., Simon,
H. D., Venkatakrishnan, V., and Weeratunga, S. K.
(1991). The nas parallel benchmarks and summary
and preliminary results. In ACM/IEEE SC, pages 158–
165, USA. ACM.
Barham, P., Dragovic, B., Fraser, K., Hand, S., Harris, T.,
Ho, A., Neugebauer, R., Pratt, I., and Warfield, A.
(2003). Xen and the art of virtualization. SIGOPS
Oper. Syst. Rev., 37(5):164–177.
Borovits, N., Kumara, I., Krishnan, P., Palma, S. D.,
Di Nucci, D., Palomba, F., Tamburri, D. A., and
van den Heuvel, W.-J. (2020). Deepiac: deep
learning-based linguistic anti-pattern detection in iac.
In Proceedings of the 4th ACM SIGSOFT Interna-
tional Workshop on Machine-Learning Techniques
for Software-Quality Evaluation, MaLTeSQuE 2020,
page 7–12, New York, NY, USA. Association for
Computing Machinery.
Che, S., Boyer, M., Meng, J., Tarjan, D., Sheaffer, J. W.,
Lee, S.-H., and Skadron, K. (2009). Rodinia: A
benchmark suite for heterogeneous computing. In
IEEE Int. Symp. on Workload Characterization, pages
44–54, DC, USA. IEEE Computer Society.
Ham, T. J., Chelepalli, B. K., Xue, N., and Lee, B. C.
(2013). Disintegrated control for energy-efficient and
heterogeneous memory systems. In IEEE HPCA,
pages 424–435.
Huang, H., Rao, J., Wu, S., Jin, H., Jiang, H., Che, H.,
and Wu, X. (2021). Towards exploiting cpu elas-
ticity via efficient thread oversubscription. In Pro-
ceedings of the 30th International Symposium on
High-Performance Parallel and Distributed Comput-
ing, HPDC ’21, page 215–226, New York, NY, USA.
Association for Computing Machinery.
Kumara, I., Vasileiou, Z., Meditskos, G., Tamburri, D. A.,
Heuvel, W.-J. V. D., Karakostas, A., Vrochidis, S., and
Kompatsiaris, I. (2020). Towards semantic detection
of smells in cloud infrastructure code. ARXIV-CS.SE.
Lepiller, J., Piskac, R., Sch
¨
af, M., and Santolucito, M.
(2021). Analyzing infrastructure as code to prevent
intra-update sniping vulnerabilities. International
Conference on Tools and Algorithms for the Construc-
tion and Analysis of Systems.
Liu, F., Tong, J., Mao, J., Bohn, R., Messina, J., Badger, L.,
and Leaf, D. (2012). NIST Cloud Computing Refer-
ence Architecture: Recommendations of the National
Institute of Standards and Technology. CreateSpace
Independent Publishing Platform, USA.
Lorenzon, A. F. and Beck Filho, A. C. S. (2019). Parallel
computing hits the power wall: principles, challenges,
and a survey of solutions. Springer Nature.
Lorenzon, A. F., De Oliveira, C. C., Souza, J. D., and Beck,
A. C. S. (2018). Aurora: Seamless optimization of
openmp applications. IEEE transactions on parallel
and distributed systems, 30(5):1007–1021.
Maas, W., de Souza, P. S. S., Luizelli, M. C., Rossi,
F. D., Navaux, P. O. A., and Lorenzon, A. F. (2024).
An ann-guided multi-objective framework for power-
performance balancing in hpc systems. In Proceed-
ings of the 21st ACM International Conference on
Computing Frontiers, CF ’24, page 138–146, New
York, NY, USA. Association for Computing Machin-
ery.
M
´
arquez, G., Villegas, M. M., and Astudillo, H. (2018).
A pattern language for scalable microservices-based
systems. In ECSA, NY, USA. ACM.
Masanet, E., Shehabi, A., Lei, N., Smith, S., and Koomey,
J. (2020). Recalibrating global data center energy-use
estimates. Science, 367(6481):984–986.
Navaux, P. O. A., Lorenzon, A. F., and da Silva Serpa, M.
(2023). Challenges in high-performance computing.
Journal of the Brazilian Computer Society, 29(1):51–
62.
Palma, S. D., Nucci, D. D., and Tamburri, D. A. (2020).
Ansiblemetrics: A python library for measuring
infrastructure-as-code blueprints in ansible. SOFT-
WAREX.
Saavedra, N. and Ferreira, J. F. (2022). Glitch: Automated
polyglot security smell detection in infrastructure as
code. ARXIV-CS.CR.
Sandobalin, J., Insfr
´
an, E., and Abrah
˜
ao, S. M. (2017). An
infrastructure modelling tool for cloud provisioning.
IEEE International Conference on Services Comput-
ing (SCC).
Sandobal
´
ın, J., Insfran, E., and Abrah
˜
ao, S. (2020). On the
Energy-Aware Node Selection for Cloud-Based Parallel Workloads with Machine Learning and Infrastructure as Code
59