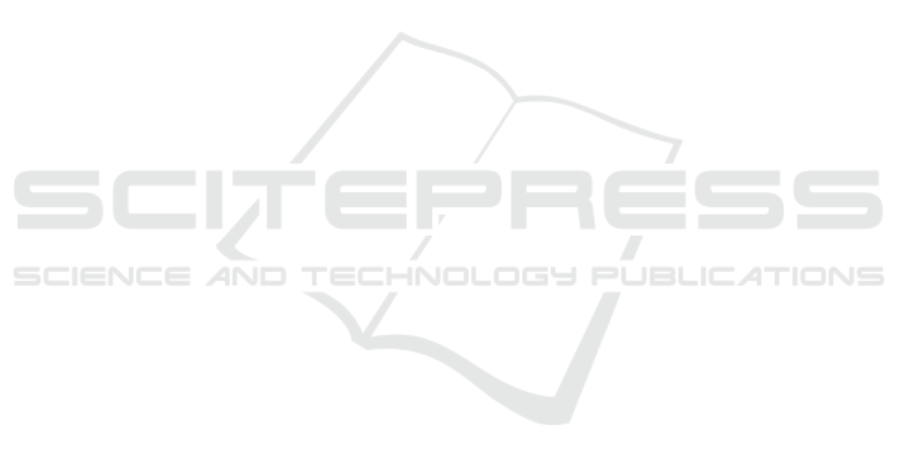
handling NULL values and outliers in univariate data.
Data quality is paramount in machine learning appli-
cations, as unreliable or unclean data can lead to poor
model performance. Our research aimed to overcome
these challenges by introducing a systematic method
that ensures cleaner, more reliable datasets, particu-
larly in applications like soil moisture sensors.
We classified outliers into three main types:
global, contextual, and collective. Each type re-
quires a different approach for detection and removal,
which is why traditional methods often fall short. Our
multi-phase approach integrates s-SVR (split-SVR)
and trend-based segmentation, followed by Loess and
regression analysis to remove outliers at each stage
of processing. By using s-SVR, we successfully re-
moved global and most contextual outliers while pre-
serving the integrity of the data. The segmentation
and regression steps handled the remaining outliers,
ensuring a comprehensive cleaning process.
The advantage of this approach is its ability to au-
tomate much of the data cleaning process, minimiz-
ing the need for manual intervention. Compared to
standard techniques like Loess or ARIMA, which ei-
ther underfit or overfit the data, our method provides
superior accuracy in removing anomalies without dis-
torting the original dataset. This makes it particularly
suitable for univariate time series data, where trends
and patterns must be preserved for reliable analysis.
4.1 Impact on Machine Learning
Models
The impact of this method on machine learning mod-
els is significant. Clean, structured data ensures that
models perform better, with more accurate predic-
tions and fewer biases. In real-world applications,
such as soil moisture monitoring, having reliable data
leads to better decision-making and more efficient re-
source management. Additionally, by removing out-
liers and imputing missing values, the model’s abil-
ity to generalize is improved, leading to better perfor-
mance in various predictive tasks.
4.2 Future Directions
While this research focuses on univariate data, there
is room to expand this technique to more com-
plex datasets. Future work could involve adapting
the multi-phase process for multivariate data, which
would open up new applications in fields such as
healthcare, telecommunications, and finance, where
data variability is high. Moreover, integrating this
approach into real-time systems and large-scale IoT
environments could prove invaluable for industries
requiring continuous monitoring and quick response
times.
Another promising area for future exploration is
the application of this method in deep learning and
large language models (LLMs). As these models
heavily depend on large, clean datasets, automating
data cleaning at this scale would greatly enhance
model performance, particularly in domains like natu-
ral language processing (NLP) and image recognition.
4.3 Conclusion
In conclusion, the multi-phase data cleaning method
we have proposed offers a comprehensive and auto-
mated solution to the critical challenge of handling
outliers and missing values in univariate datasets. By
removing global, contextual, and collective outliers
in successive stages, this approach significantly en-
hances the quality of data used in machine learning
models. The method’s scalability and adaptability
make it applicable across various industries that rely
on univariate time series data.
Improving data quality is vital to ensuring the ac-
curacy and reliability of machine learning models, es-
pecially in fields where precision is critical. Investing
in data cleaning processes, as demonstrated in this re-
search, leads to better predictive models and more in-
formed decision-making, thus benefiting a wide range
of applications.
ACKNOWLEDGEMENTS
The authors would like to express their gratitude to
Dr. Rajan Nikam, whose expertise in mathematics
was invaluable in developing the mathematical frame-
work for this research. Their insights and guidance
significantly contributed to the success of this work.
REFERENCES
Aggarwal, C. C. (2015). Outlier Analysis. Springer, Berlin,
1st edition.
Box, G. E. P. and Jenkins, G. M. (1976). Time Series Analy-
sis: Forecasting and Control. Holden-Day, San Fran-
cisco, 2nd edition.
Deng, Y.-F., Jin, X., and Zhong, Y.-X. (2005). Ensemble
svr for prediction of time series. In 2005 Interna-
tional Conference on Machine Learning and Cyber-
netics. IEEE.
Mahdavinejad, M. S., Rezvan, M., Barekatain, M., Adibi,
P., Barnaghi, P., and Sheth, A. P. (2018). Machine
learning for internet of things data analysis: A survey.
In Digital Communications and Networks. Elsevier.
IoTBDS 2025 - 10th International Conference on Internet of Things, Big Data and Security
368