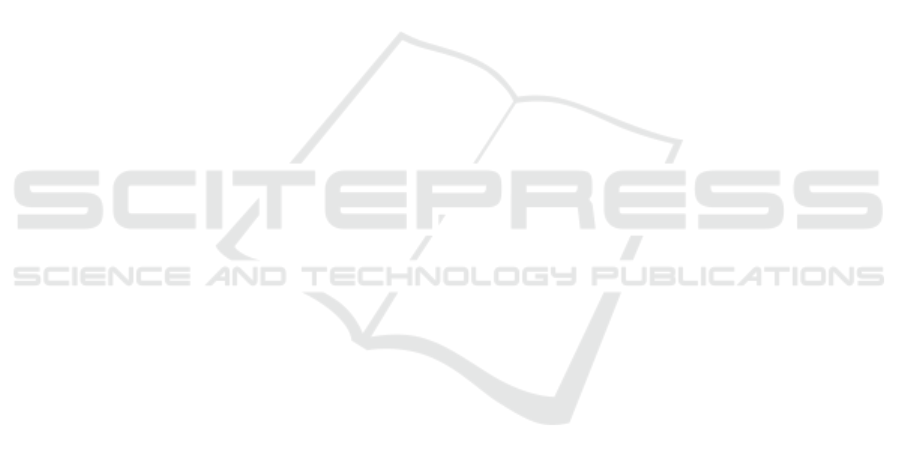
Kwon, H., and Cuzzocrea, A., editors, 2020 IEEE In-
ternational Conference on Big Data and Smart Com-
puting, BigComp 2020, Busan, Korea (South), Febru-
ary 19-22, 2020, pages 71–74. IEEE.
Fahad, L. G. and Rajarajan, M. (2015). Anomalies detection
in smart-home activities. In 2015 IEEE 14th interna-
tional conference on machine learning and applica-
tions (ICMLA), pages 419–422. IEEE.
Fernandes, E., Jung, J., and Prakash, A. (2016). Secu-
rity analysis of emerging smart home applications. In
IEEE Symposium on Security and Privacy, SP 2016,
San Jose, CA, USA, May 22-26, 2016, pages 636–654.
IEEE Computer Society.
Fu, C., Zeng, Q., Chi, H., Du, X., and Valluru, S. L. (2022).
Iot phantom-delay attacks: Demystifying and exploit-
ing iot timeout behaviors. In 52nd Annual IEEE/IFIP
International Conference on Dependable Systems and
Networks, DSN 2022, Baltimore, MD, USA, June 27-
30, 2022, pages 428–440. IEEE.
Fu, C., Zeng, Q., and Du, X. (2021). Hawatcher:
Semantics-aware anomaly detection for appified smart
homes. In Bailey, M. D. and Greenstadt, R., edi-
tors, 30th USENIX Security Symposium, USENIX Se-
curity 2021, August 11-13, 2021, pages 4223–4240.
USENIX Association.
Guan, Z., Lu, X., Wang, N., Wu, J., Du, X., and Guizani, M.
(2020). Towards secure and efficient energy trading in
iiot-enabled energy internet: A blockchain approach.
Future Gener. Comput. Syst., 110:686–695.
Guo, Y., Liao, W., Wang, Q., Yu, L., Ji, T., and Li, P.
(2018). Multidimensional time series anomaly de-
tection: A gru-based gaussian mixture variational au-
toencoder approach. In Zhu, J. and Takeuchi, I.,
editors, Proceedings of The 10th Asian Conference
on Machine Learning, ACML 2018, Beijing, China,
November 14-16, 2018, volume 95 of Proceedings of
Machine Learning Research, pages 97–112. PMLR.
Hnat, T. W., Srinivasan, V., Lu, J., Sookoor, T. I., Dawson,
R., Stankovic, J. A., and Whitehouse, K. (2011). The
hitchhiker’s guide to successful residential sensing de-
ployments. In Liu, J., Levis, P. A., and R
¨
omer, K.,
editors, Proceedings of the 9th International Confer-
ence on Embedded Networked Sensor Systems, SenSys
2011, Seattle, WA, USA, November 1-4, 2011, pages
232–245. ACM.
Hochreiter, S. and Schmidhuber, J. (1997). Long short-term
memory. Neural Comput., 9(8):1735–1780.
Hoffner, Y., Kaufman, E., Amir, A., Yovel, E., and Harel,
F. (2024). Automation of smart homes with multi-
ple rule sources. In Kobusinska, A., Jacobsson, A.,
and Chang, V., editors, Proceedings of the 9th Inter-
national Conference on Internet of Things, Big Data
and Security, IoTBDS 2024, Angers, France, April 28-
30, 2024, pages 40–52. SCITEPRESS.
Hsieh, R., Chou, J., and Ho, C. (2019). Unsupervised on-
line anomaly detection on multivariate sensing time
series data for smart manufacturing. In 12th IEEE
Conference on Service-Oriented Computing and Ap-
plications, SOCA 2019, Kaohsiung, Taiwan, Novem-
ber 18-21, 2019, pages 90–97. IEEE.
Hundman, K., Constantinou, V., Laporte, C., Colwell,
I., and S
¨
oderstr
¨
om, T. (2018). Detecting spacecraft
anomalies using lstms and nonparametric dynamic
thresholding. In Guo, Y. and Farooq, F., editors,
Proceedings of the 24th ACM SIGKDD International
Conference on Knowledge Discovery & Data Mining,
KDD 2018, London, UK, August 19-23, 2018, pages
387–395. ACM.
Jiang, C., Fu, C., Zhao, Z., and Du, X. (2022). Effective
anomaly detection in smart home by integrating event
time intervals. Procedia Computer Science, 210:53–
60. The 13th International Conference on Emerg-
ing Ubiquitous Systems and Pervasive Networks (EU-
SPN) / The 12th International Conference on Current
and Future Trends of Information and Communication
Technologies in Healthcare (ICTH-2022) / Affiliated
Workshops.
Kieu, T., Yang, B., Guo, C., and Jensen, C. S. (2019). Out-
lier detection for time series with recurrent autoen-
coder ensembles. In Kraus, S., editor, Proceedings
of the Twenty-Eighth International Joint Conference
on Artificial Intelligence, IJCAI 2019, Macao, China,
August 10-16, 2019, pages 2725–2732. ijcai.org.
Li, D., Chen, D., Jin, B., Shi, L., Goh, J., and Ng, S.
(2019). MAD-GAN: multivariate anomaly detection
for time series data with generative adversarial net-
works. In Tetko, I. V., Kurkov
´
a, V., Karpov, P., and
Theis, F. J., editors, Artificial Neural Networks and
Machine Learning - ICANN 2019: Text and Time Se-
ries - 28th International Conference on Artificial Neu-
ral Networks, Munich, Germany, September 17-19,
2019, Proceedings, Part IV, volume 11730 of Lecture
Notes in Computer Science, pages 703–716. Springer.
MacQueen, J. B. (1967). Some methods for classification
and analysis of multivariate observations. In Cam, L.
M. L. and Neyman, J., editors, Proc. of the fifth Berke-
ley Symposium on Mathematical Statistics and Prob-
ability, volume 1, pages 281–297. University of Cali-
fornia Press.
Manevitz, L. M. and Yousef, M. (2001). One-class svms for
document classification. J. Mach. Learn. Res., 2:139–
154.
Markou, M. and Singh, S. (2003). Novelty detection: a re-
view—part 1: statistical approaches. Signal Process-
ing, 83(12):2481–2497.
McLachlan, G. J. and Basford, K. E. (1988). Mixture mod-
els: Inference and applications to clustering. Marcel
Dekker, New York.
Park, D., Hoshi, Y., and Kemp, C. C. (2017). A mul-
timodal anomaly detector for robot-assisted feeding
using an lstm-based variational autoencoder. CoRR,
abs/1711.00614.
Ramapatruni, S., Narayanan, S. N., Mittal, S., Joshi, A.,
and Joshi, K. (2019). Anomaly detection models for
smart home security. In 2019 IEEE 5th Intl Confer-
ence on Big Data Security on Cloud (BigDataSecu-
rity), IEEE Intl Conference on High Performance and
Smart Computing, (HPSC) and IEEE Intl Conference
on Intelligent Data and Security (IDS), pages 19–24.
Shen, L., Li, Z., and Kwok, J. (2020). Timeseries anomaly
detection using temporal hierarchical one-class net-
IoTBDS 2025 - 10th International Conference on Internet of Things, Big Data and Security
162