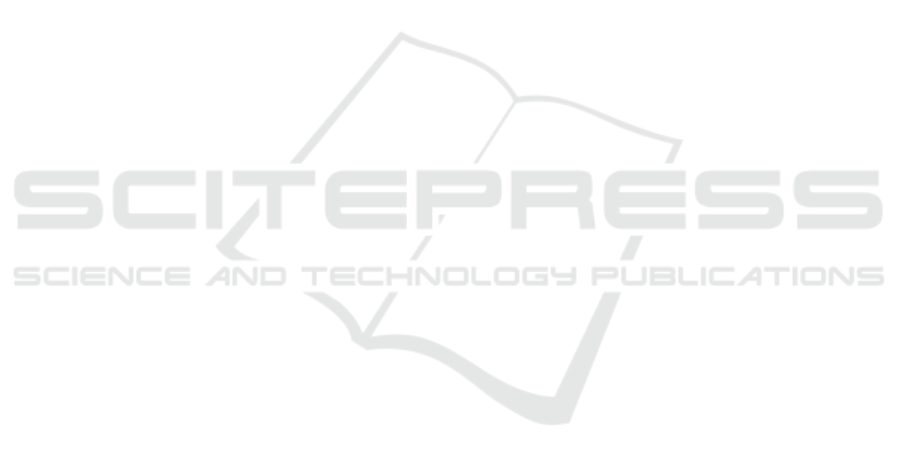
REFERENCES
Abbas, M., Jam, F. A., and Khan, T. I. (2024). Is it harmful
or helpful? examining the causes and consequences of
generative ai usage among university students. Inter-
national Journal of Educational Technology in Higher
Education, 21(1):10.
Budde, J., Tobor, J., and Friedrich, J. (2024). K
¨
unstliche
Intelligenz. Wo stehen die deutschen Hochschulen?
Hochschulforum Digitalisierung.
Chang, D. H., Lin, M. P.-C., Hajian, S., and Wang, Q. Q.
(2023). Educational design principles of using ai chat-
bot that supports self-regulated learning in education:
Goal setting, feedback, and personalization. Sustain-
ability, 15(17):12921.
Christensen, R. and Knezek, G. (1999). Stages of adop-
tion for technology in education. Computers in New
Zealand Schools, 11(3):25–29.
Dakshit, S. (2024). Faculty perspectives on the potential
of rag in computer science higher education. ACM
SIGITE.
Dong, C., Chen, K., Cheng, S., and Wen, C. (2023). How
to build an ai tutor using llms and rag. Unpublished
Manuscript.
Doosthosseini, A., Decker, J., Nolte, H., and Kunkel, J. M.
(2024). Chat ai: A seamless slurm-native solution for
hpc-based services.
Fan, W., Ding, Y., Ning, L., Wang, S., Li, H., Yin, D., Chua,
T.-S., and Li, Q. (2024). A survey on rag meeting llms:
Towards retrieval-augmented large language models.
In Proceedings of the 30th ACM SIGKDD Conference
on Knowledge Discovery and Data Mining, KDD ’24,
page 6491–6501, New York, NY, USA. Association
for Computing Machinery.
Kahl, S., L
¨
offler, F., Maciol, M., Ridder, F., Schmitz,
M., Spanagel, J., Wienkamp, J., Burgahn, C., and
Schilling, M. (2024). Enhancing ai tutoring in
robotics education: Evaluating the effect of retrieval-
augmented generation and fine-tuning on large lan-
guage models. Autonomous Intelligent Systems
Group.
Knezek, G. and Christensen, R. (2015). The will, skill, tool
model of technology integration: Adding pedagogy as
a new model construct. International Association for
Development of the Information Society.
Lewis, P., Perez, E., Piktus, A., Petroni, F., Karpukhin,
V., Goyal, N., K
¨
uttler, H., Lewis, M., tau
Yih, W., Rockt
¨
aschel, T., Riedel, S., and Kiela,
D. (2020). Retrieval-augmented generation for
knowledge-intensive NLP tasks. In Proceedings of
the 34th Conference on Neural Information Process-
ing Systems (NeurIPS 2020), Vancouver, Canada.
Liu, R., Zenke, C., Liu, C., Holmes, A., Thornton, P., and
Malan, D. J. (2024). Teaching cs50 with ai: leveraging
generative artificial intelligence in computer science
education. In Proceedings of the 55th ACM Techni-
cal Symposium on Computer Science Education V. 1,
pages 750–756.
Maynez, J., Narayan, S., Bohnet, B., and McDonald, R.
(2020). On faithfulness and factuality in abstractive
summarization. In Jurafsky, D., Chai, J., Schluter,
N., and Tetreault, J., editors, Proceedings of the 58th
Annual Meeting of the Association for Computational
Linguistics, pages 1906–1919, Online. Association
for Computational Linguistics.
Medienp
¨
adagogischer Forschungsverbund S
¨
udwest (2024).
Jim-Studie. Jugend, Information,(Multi) Media. Ba-
sisstudie zum Medienumgang.
Mullins, E. A., Portillo, A., Ruiz-Rohena, K., and Piplai, A.
(2024). Enhancing classroom teaching with llms and
rag. In Proceedings of SIGITE 2024, El Paso, TX.
ACM.
Ng, D. T. K., Tan, C. W., and Leung, J. K. L. (2024). Em-
powering student self-regulated learning and science
education through chatgpt: A pioneering pilot study.
British Journal of Educational Technology.
Ouyang, F. and Jiao, P. (2021). Artificial intelligence in
education: The three paradigms. Computers and Ed-
ucation: Artificial Intelligence, 2:100020.
Perez, F. and Ribeiro, I. (2022). Ignore previous prompt:
Attack techniques for language models. In Proceed-
ings of the Workshop on Machine Learning Safety at
NeurIPs.
Petroni, F., Rockt
¨
aschel, T., Riedel, S., Lewis, P., Bakhtin,
A., Wu, Y., and Miller, A. (2019). Language models
as knowledge bases? In Inui, K., Jiang, J., Ng, V.,
and Wan, X., editors, Proceedings of the 2019 Con-
ference on Empirical Methods in Natural Language
Processing and the 9th International Joint Conference
on Natural Language Processing (EMNLP-IJCNLP),
pages 2463–2473, Hong Kong, China. Association for
Computational Linguistics.
Reimann, P. (2010). Design-based research. In Method-
ological choice and design: Scholarship, policy and
practice in social and educational research, pages 37–
50. Springer.
Shuster, K., Poff, S., Chen, M., Kiela, D., and Weston, J.
(2021). Retrieval augmentation reduces hallucination
in conversation. In Moens, M.-F., Huang, X., Specia,
L., and Yih, S. W.-t., editors, Findings of the Associ-
ation for Computational Linguistics: EMNLP 2021,
pages 3784–3803, Punta Cana, Dominican Republic.
Association for Computational Linguistics.
S
¨
uße, T. and Kobert, M. (2023). Generative ai at school-
insights from a study about german students’ self-
reported usage, the role of students’ action-guiding
characteristics, perceived learning success and the
consideration of contextual factors. Zenodo.
Th
¨
us, D., Malone, S., and Br
¨
unken, R. (2024). Explor-
ing generative ai in higher education: A rag system to
enhance student engagement with scientific literature.
Frontiers in Psychology, 15.
Velazquez, C. M. (2006). Cross-cultural validation of the
will, skill, tool model of technology integration. Uni-
versity of North Texas.
Yu, J., Wu, Y., Shu, D., Jin, M., and Xing, X. (2023). As-
sessing prompt injection risks in 200+ custom gpts.
arXiv preprint arXiv:2311.11538.
CSEDU 2025 - 17th International Conference on Computer Supported Education
378