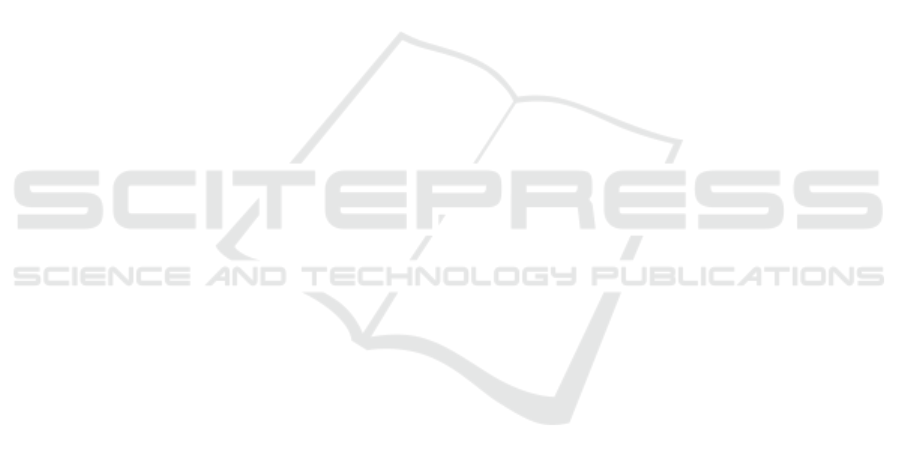
tion, processing, and analysis, streamlining research
workflows from traffic capture to inference tasks. Its
design is based on a four-stage solution structure,
combining reusable software components with future
extensibility. Currently, the platform supports Zig-
bee and 433MHz traffic collection through horizon-
tally scalable Collection Agents, with Elasticsearch
managing data storage and Apache Airflow enabling
workflow automation.
The case study demonstrated PacketZapper’s ef-
fectiveness in automating tasks and supporting data
exploration, successfully addressing the challenges
of IoT traffic collection and automation. While the
platform presents a learning curve, particularly with
Python, Elasticsearch, and Airflow, it offers signif-
icant flexibility and advanced functionality for re-
searchers. Future enhancements, such as support for
additional protocols like Bluetooth and Z-Wave, will
further extend its applicability and relevance in IoT
traffic research.
ACKNOWLEDGEMENT
This work received funding from the Research Coun-
cil of Norway through the SFI Norwegian Centre for
Cybersecurity in Critical Sectors (NORCICS), project
no. 310105.
REFERENCES
Acar, A., Fereidooni, H., Abera, T., Sikder, A. K., Miet-
tinen, M., Aksu, H., Conti, M., Sadeghi, A.-R., and
Uluagac, S. (2020). Peek-a-boo: I see your smart
home activities, even encrypted! In Proceedings of
the 13th ACM Conference on Security and Privacy in
Wireless and Mobile Networks, pages 207–218.
Akestoridis, D.-G., Harishankar, M., Weber, M., and Tague,
P. (2020). Zigator: Analyzing the security of zigbee-
enabled smart homes. In Proceedings of the 13th ACM
Conference on Security and Privacy in Wireless and
Mobile Networks, pages 77–88.
Akestoridis, D.-G., Sekar, V., and Tague, P. (2022). On
the Security of Thread Networks: Experimentation
with OpenThread-Enabled Devices. In Proceedings
of the 15th ACM Conference on Security and Privacy
in Wireless and Mobile Networks, pages 233–244.
Akestoridis, D.-G. and Tague, P. (2021). HiveGuard: A
Network Security Monitoring Architecture for Zigbee
Networks. In 2021 IEEE Conference on Communi-
cations and Network Security (CNS), pages 209–217.
IEEE.
Apthorpe, N., Reisman, D., Sundaresan, S., Narayanan, A.,
and Feamster, N. (2017). Spying on the smart home:
Privacy attacks and defenses on encrypted iot traffic.
arXiv preprint arXiv:1708.05044.
Cook, D., Schmitter-Edgecombe, M., Crandall, A.,
Sanders, C., and Thomas, B. (2009). Collecting and
disseminating smart home sensor data in the CASAS
project. In Proceedings of the CHI workshop on devel-
oping shared home behavior datasets to advance HCI
and ubiquitous computing research, pages 1–7. IEEE.
Farahani, S. (2011). ZigBee wireless networks and
transceivers. newnes.
Gu, T., Fang, Z., Abhishek, A., Fu, H., Hu, P., and Mo-
hapatra, P. (2020a). IoTGaze: IoT security enforce-
ment via wireless context analysis. In IEEE INFO-
COM 2020-IEEE Conference on Computer Commu-
nications, pages 884–893. IEEE.
Gu, T., Fang, Z., Abhishek, A., and Mohapatra, P. (2020b).
Iotspy: Uncovering human privacy leakage in iot net-
works via mining wireless context. In 2020 IEEE 31st
Annual International Symposium on Personal, Indoor
and Mobile Radio Communications, pages 1–7. IEEE.
Hafeez, I., Antikainen, M., Ding, A. Y., and Tarkoma, S.
(2020). IoT-KEEPER: Detecting malicious IoT net-
work activity using online traffic analysis at the edge.
IEEE Transactions on Network and Service Manage-
ment, 17(1):45–59.
He, X., Yang, Y., Zhou, W., Wang, W., Liu, P., and Zhang,
Y. (2021). Fingerprinting mainstream iot platforms
using traffic analysis. IEEE Internet of Things Jour-
nal, 9(3):2083–2093.
Hye Oh, J., Ji Yang, S., and Rag Do, Y. (2014). Healthy,
natural, efficient and tunable lighting: four-package
white leds for optimizing the circadian effect, color
quality and vision performance. Light: Science & Ap-
plications, 3(2):e141–e141.
Karlicek, R. F. (2012). Smart lighting-beyond simple illu-
mination. In 2012 IEEE Photonics Society Summer
Topical Meeting Series, pages 147–148. IEEE.
Lee, M.-C., Lin, J.-C., and Owe, O. (2019). PDS: Deduce
elder privacy from smart homes. Internet of Things,
7:100072.
Lopez-Neira, I., Patel, T., Parkin, S., Danezis, G., and
Tanczer, L. (2019). ‘internet of things’: How abuse
is getting smarter.
LR-WPANs (2011). IEEE Standard for Local and
metropolitan area networks–Part 15.4: Low-Rate
Wireless Personal Area Networks (LR-WPANs).
https://ieeexplore.ieee.org/document/6012487. [On-
line; accessed 02-February-2025].
Ren, J., Dubois, D. J., Choffnes, D., Mandalari, A. M.,
Kolcun, R., and Haddadi, H. (2019). Information
exposure from consumer iot devices: A multidimen-
sional, network-informed measurement approach. In
Proceedings of the Internet Measurement Conference,
pages 267–279.
Shafqat, N., Dubois, D. J., Choffnes, D., Schulman, A.,
Bharadia, D., and Ranganathan, A. (2022). Zleaks:
Passive inference attacks on Zigbee based smart
homes. In International Conference on Applied
Cryptography and Network Security, pages 105–125.
Springer.
ZigBee (2015). ZigBee Specification. https:
//zigbeealliance.org/wp-content/uploads/2019/11/
docs-05-3474-21-0csg-zigbee-specification.pdf.
[Online; accessed 02-February-2025].
PacketZapper: A Scalable and Automated Platform for IoT Traffic Collection and Analysis
377