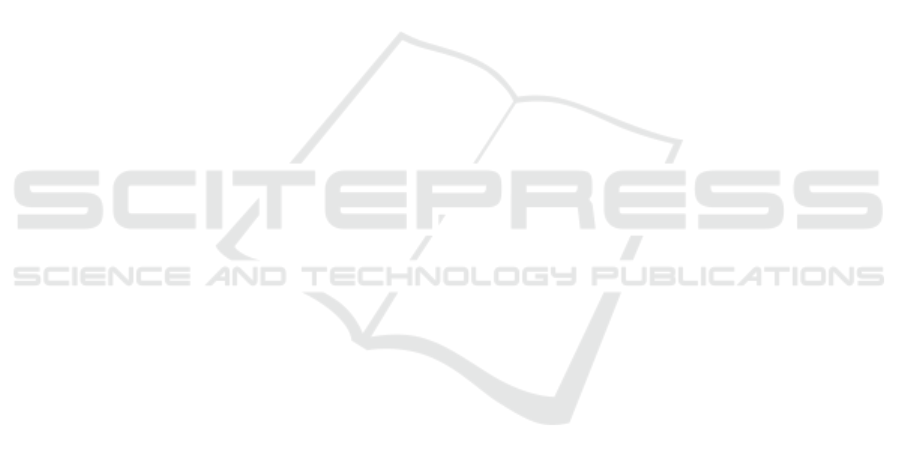
challenges would validate the framework’s practical-
ity and provide opportunities to refine its design and
functionality based on operational feedback.
In conclusion, DIRA-LDT represents a signif-
icant advancement in resource allocation for pro-
grammable data centers, combining the predictive
power of LLMs with the real-time insights of Dig-
ital Twins. Its ability to outperform existing meth-
ods across multiple metrics underscores its potential
to address the challenges of modern cloud-native en-
vironments. As data centers evolve, the adaptability
and intelligence of DIRA-LDT provide a strong foun-
dation for future innovation, paving the way for more
efficient, sustainable, and intelligent resource man-
agement solutions.
ACKNOWLEDGEMENT
This work was supported by the Sao Paulo Research
Foundation (FAPESP), grant 2023/00794-9. This
study was partially funded by CAPES, Brazil - Fi-
nance Code 001.
REFERENCES
Abdullah, M., Lu, K., Wieder, P., and Yahyapour, R. (2017).
A heuristic-based approach for dynamic vms consoli-
dation in cloud data centers. Arabian Journal for Sci-
ence and Engineering, 42:3535–3549.
Al-Debagy, O. and Martinek, P. (2018). A comparative re-
view of microservices and monolithic architectures. In
2018 IEEE 18th International Symposium on Compu-
tational Intelligence and Informatics (CINTI), pages
000149–000154.
Alanezi, K. and Mishra, S. (2023). Towards a scalable
architecture for building digital twins at the edge.
In 2023 IEEE/ACM Symposium on Edge Computing
(SEC), pages 325–329.
Bai, R. and Song, X. (2024). Research on information sys-
tem architecture based on microservice architecture.
In 2024 5th International Conference on Information
Science, Parallel and Distributed Systems (ISPDS),
pages 606–610.
Buchaca, D., Berral, J. L., Wang, C., and Youssef, A.
(2020). Proactive container auto-scaling for cloud
native machine learning services. In 2020 IEEE
13th International Conference on Cloud Computing
(CLOUD), pages 475–479.
Daradkeh, T. and Agarwal, A. (2022). Cloud workload and
data center analytical modeling and optimization us-
ing deep machine learning. Network, 2(4):643–669.
Deng, S., Zhao, H., Huang, B., Zhang, C., Chen, F., Deng,
Y., Yin, J., Dustdar, S., and Zomaya, A. Y. (2024).
Cloud-native computing: A survey from the perspec-
tive of services. Proceedings of the IEEE, 112(1):12–
46.
Hongyu, Y. and Anming, W. (2023). Migrating from mono-
lithic applications to cloud native applications. In
2023 8th International Conference on Computer and
Communication Systems (ICCCS), pages 775–779.
Kim, H. and Ben-Othman, J. (2023). Eco-friendly low re-
source security surveillance framework toward green
ai digital twin. IEEE Communications Letters,
27(1):377–380.
Li, H., Wang, S. X., Shang, F., Niu, K., and Song, R. (2024).
Efficient resource allocation in cloud computing envi-
ronments using ai-driven predictive analytics. Applied
and Computational Engineering, 82:17–23.
Li, H., Zhang, T., and Huang, Y. (2021). Digital twin tech-
nology for integrated energy system and its applica-
tion. In 2021 IEEE 1st International Conference on
Digital Twins and Parallel Intelligence (DTPI), pages
422–425.
Lombardo, A., Morabito, G., Quattropani, S., and Ricci,
C. (2022). Design, implementation, and testing of a
microservices-based digital twins framework for net-
work management and control. In 2022 IEEE 23rd In-
ternational Symposium on a World of Wireless, Mobile
and Multimedia Networks (WoWMoM), pages 590–
595.
Mishra, R., Jaiswal, N., Prakash, R., and Barwal, P. N.
(2022). Transition from monolithic to microservices
architecture: Need and proposed pipeline. In 2022
International Conference on Futuristic Technologies
(INCOFT), pages 1–6.
Mohammad, A. F., Clark, B., Agarwal, R., and Summers, S.
(2023). Llm/gpt generative ai and artificial general in-
telligence (agi): The next frontier. In 2023 Congress
in Computer Science, Computer Engineering, & Ap-
plied Computing (CSCE), pages 413–417.
Morichetta, A., Pusztai, T., Vij, D., Pujol, V. C., Raith,
P., Xiong, Y., Nastic, S., Dustdar, S., and Zhang,
Z. (2023). Demystifying deep learning in predic-
tive monitoring for cloud-native slos. In 2023 IEEE
16th International Conference on Cloud Computing
(CLOUD), pages 1–11.
Nguyen, N. T. and Kim, Y. (2022). A Design of Resource
Allocation Structure for Multi-Tenant Services in Ku-
bernetes Cluster.
Peng, K., Huang, H., Bilal, M., and Xu, X. (2022). Dis-
tributed incentives for intelligent offloading and re-
source allocation in digital twin driven smart indus-
try. IEEE Transactions on Industrial Informatics,
19(3):3133–3143.
Raghunandan, A., Kalasapura, D., and Caesar, M. (2023).
Digital twinning for microservice architectures. In
ICC 2023 - IEEE International Conference on Com-
munications, pages 3018–3023.
Shabka, Z. and Zervas, G. (2021). Resource allocation in
disaggregated data centre systems with reinforcement
learning. arXiv preprint arXiv:2106.02412.
Sharma, V., Sharma, K., and Kumar, A. (2024). Ai and
digital twins transforming healthcare iot. In 2024 14th
CLOSER 2025 - 15th International Conference on Cloud Computing and Services Science
70