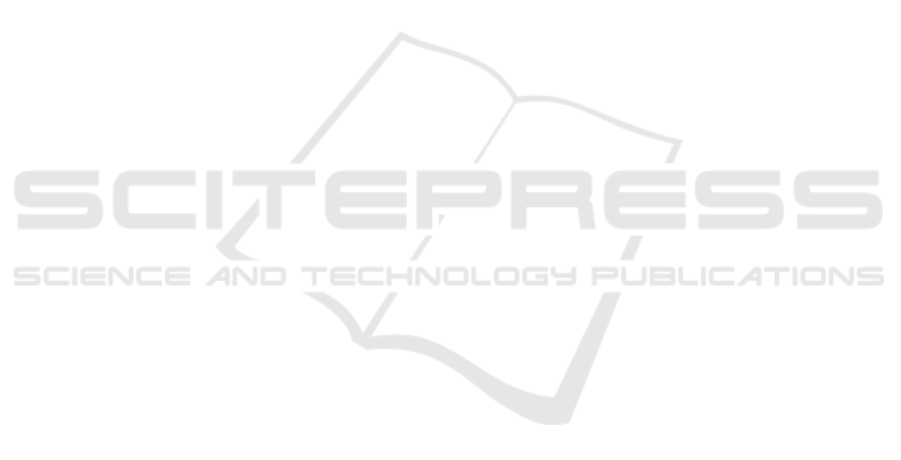
REFERENCES
Allan, E. G. and Driscoll, D. L. (2014). The three-fold ben-
efit of reflective writing: Improving program assess-
ment, student learning, and faculty professional de-
velopment. Assessing Writing, 21:37–55.
Alnajashi, A. (2024). Investigating the accuracy of large
language models ’chatgpt-4’ in grading students’ writ-
ing according to a specific rubric.
Awidi, I. T. (2024). Comparing expert tutor evaluation of
reflective essays with marking by generative artificial
intelligence (ai) tool. Computers and Education: Ar-
tificial Intelligence, 6:100226.
Bhojan, A. and Hu, Y. (2024). Play testing and reflec-
tive learning ai tool for creative media courses. In
Proceedings of the 2024 CSEDU, pages 146–158. IN-
STICC, SciTePress.
Chan, C. and Lee, K. (2021). Reflection literacy: A
multilevel perspective on the challenges of using re-
flections in higher education through a comprehen-
sive literature review. Educational Research Review,
32:100376.
Chen, P. P. and Bonner, S. M. (2020). A framework for
classroom assessment, learning, and self-regulation.
Assessment in Education: Principles, Policy & Prac-
tice, 27(4):373–393.
Davis, J., Van Bulck, L., Durieux, B. N., and Lindvall, C.
(2024). The temperature feature of chatgpt: Modify-
ing creativity for clinical research. JMIR Hum Fac-
tors, 11:e53559.
Ekin, S. (2023). Prompt engineering for chatgpt: A quick
guide to techniques, tips, and best practices.
Gee, J. (2003). What video games have to teach us about
learning and literacy. Computers in Entertainment,
1:20.
Hackl, V., M
¨
uller, A. E., Granitzer, M., and Sailer, M.
(2023). Is gpt-4 a reliable rater? evaluating consis-
tency in gpt-4’s text ratings. Frontiers in Education,
8.
Han, J., Yoo, H., Myung, J., Kim, M., Lim, H., Kim,
Y., Lee, T. Y., Hong, H., Kim, J., Ahn, S., and
Oh, A. (2024). Llm-as-a-tutor in efl writing educa-
tion: Focusing on evaluation of student-llm interac-
tion. KAIST, South Korea.
Hattie, J. and Timperley, H. (2007). The power of feedback.
Review of Educational Research, 77(1):81–112.
Jacobsen, L. and Weber, K. (2023). The promises and pit-
falls of chatgpt as a feedback provider in higher ed-
ucation: An exploratory study of prompt engineering
and the quality of ai-driven feedback.
Jukiewicz, M. (2024). The future of grading programming
assignments in education: The role of chatgpt in au-
tomating the assessment and feedback process. Think-
ing Skills and Creativity, 52:101522.
Kember, D., McKay, J., Sinclair, K., and Wong, F. K. Y.
(2008). A four-category scheme for coding and as-
sessing the level of reflection in written work. Assess-
ment & Evaluation in Higher Education, 33(4):369–
379.
Kolb, D. (1984). Experiential Learning: Experience As The
Source Of Learning And Development, volume 1.
Lee, G.-G., Latif, E., Wu, X., Liu, N., and Zhai, X. (2024a).
Applying large language models and chain-of-thought
for automatic scoring. Computers and Education: Ar-
tificial Intelligence, 6:100213.
Lee, Y., Son, K., Kim, T. S., Kim, J., Chung, J. J. Y.,
Adar, E., and Kim, J. (2024b). One vs. many: Com-
prehending accurate information from multiple erro-
neous and inconsistent ai generations. FAccT ’24,
page 2518–2531. ACM.
Masikisiki, B., Marivate, V., and Hlophe, Y. (2024). Inves-
tigating the efficacy of large language models in re-
flective assessment methods through chain of thought
prompting. pages 44–49.
Meyer, J., Jansen, T., Schiller, R., Liebenow, L. W., Stein-
bach, M., Horbach, A., and Fleckenstein, J. (2024).
Using llms to bring evidence-based feedback into
the classroom: Ai-generated feedback increases sec-
ondary students’ text revision, motivation, and posi-
tive emotions. Computers and Education: Artificial
Intelligence, 6:100199.
Mohamad, S. K. and Tasir, Z. (2023). Exploring how
feedback through questioning may influence reflective
thinking skills based on association rules mining tech-
nique. Thinking Skills and Creativity, 47:101231.
Playfoot, D., Horry, R., and Pink, A. E. (2024). What’s the
use of being nice? characteristics of feedback com-
ments that students intend to use in improving their
work. Assessment and Evaluation in Higher Educa-
tion, pages 1–12.
Prilop, C., Weber, K., and Kleinknecht, M. (2019).
Entwicklung eines video- und textbasierten Instru-
ments zur Messung kollegialer Feedbackkompetenz
von Lehrkr
¨
aften, pages 153–163.
Quek, S. L. (2024). Play testing and reflective learning tool
with ai/ml based automated feedback for creative me-
dia courses. B. Comp. Dissertation, Project Number
H1352410, 2024/2025.
Sadler, D. R. (1989). Formative assessment and the de-
sign of instructional systems. Instructional Science,
18(2):119–144.
Stahl, M., Biermann, L., Nehring, A., and Wachsmuth, H.
(2024). Exploring llm prompting strategies for joint
essay scoring and feedback generation. Leibniz Uni-
versity Hannover.
Tan, K. Q. J. (2022). Playtesting and reflective learning tool
for creative media courses. B. Comp. Dissertation,
Project Number H1352060, 2021/2022.
Tsingos, C., Bosnic-Anticevich, S., Lonie, J. M., and Smith,
L. (2015). A model for assessing reflective practices
in pharmacy education. American Journal of Pharma-
ceutical Education, 79(8):124.
Vygotsky, L. S. (1978). Mind in society: The development
of higher psychological processes, volume 86. Har-
vard university press.
Yuan, W., Liu, P., and Gall’e, M. (2024). Llmcrit: Teaching
large language models to use criteria. In Annual Meet-
ing of the Association for Computational Linguistics.
Yvdal, A. and Bergstr
¨
om, O. (2024). Chatgpt-4’s effective-
ness in providing feedback on argumentative writing
in higher education : A case study.
CSEDU 2025 - 17th International Conference on Computer Supported Education
394