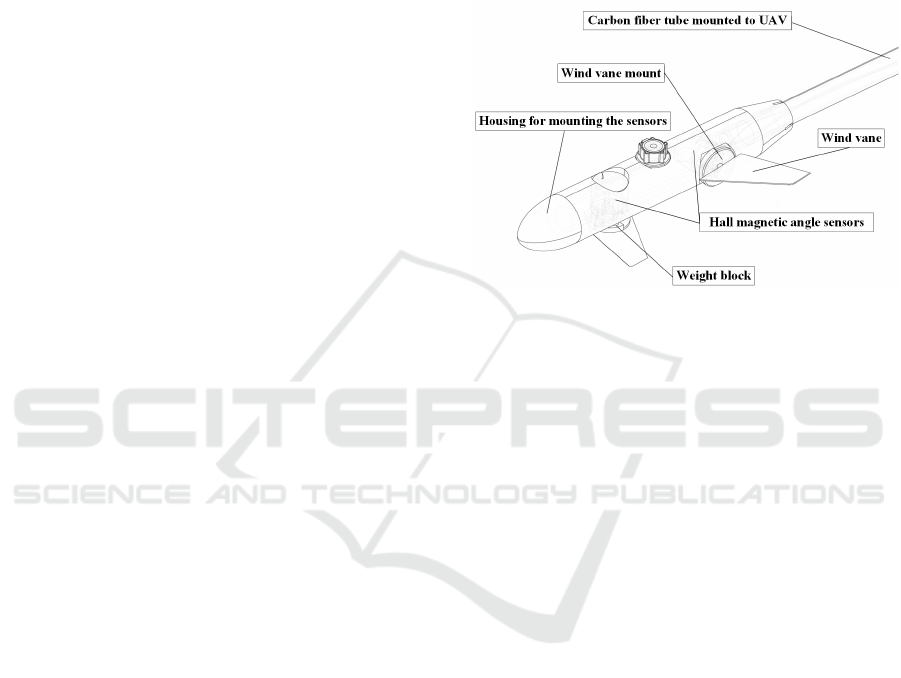
egorized into three aspects: (1) Motivated by (Liu
et al., 2024), a novel measurement method was de-
veloped for small fixed-wing UAVs, integrating data
acquisition using wind vane-based sensors with esti-
mation via the UKF algorithm; (2) For small fixed-
wing UAVs equipped with front-mounted propellers,
a detailed analysis was conducted to identify potential
factors impacting the measurement accuracy of sen-
sors installed beneath the wing; (3) To address inaccu-
racies in sensor measurements, an AOA/AOS fusion
method based on the adaptive network-based fuzzy
inference system (ANFIS) was proposed, leveraging
UKF-estimated values for compensation.
The remainder of this paper is structured as fol-
lows: Section 2 presents the design of the wind vane-
based sensor and the UKF-based AOA/AOS esti-
mation method. Section 3 investigates potential fac-
tors influencing the measurement accuracy of sen-
sors mounted beneath the wing and proposes an
AOA/AOS fusion method utilizing ANFIS. Section 4
presents the flight test conducted for data collection
and the simulation experiments designed to validate
the proposed data fusion approach. Finally, Section 5
provides a comprehensive summary of the study.
2 PRELIMINARY
Two approaches are available for recording AOA and
AOS. The first involves using the low-cost wind vane-
based AOA and AOS sensors. The second relies on
estimating AOA and AOS through the UKF algorithm
from inertial data.
2.1 Low-Cost AOA/AOS Sensors
A set of AOA and AOS sensors based on the rotation
of wind vane blades was employed to measure the α
and β angle (also known as AOA and AOS) during
the flight. The sensor housing features a streamlined
design and is fabricated using 3D printing with alu-
minum alloy. Inside the sensor, two brushless Hall
magnetic angle sensors are positioned perpendicular
to one another at a 90
◦
. Two carbon fiber wind vane
blades are affixed to the rotational axes of these sen-
sors, respectively. Additionally, a balancing block is
mounted on the symmetrical side of the wind vane to
ensure weight balance. The total weight of the sen-
sor assembly is approximately 25 g, with an encoder
resolution of 0.088
◦
.
When subjected to the incoming flow, the wind
vane blades drive the rotational axes of the angle sen-
sors. The phase and intensity of the rotational mag-
netic field are detected by monitoring the Hall ele-
ment array, enabling the measured values of the α
and β to be converted into digital signals and di-
rectly output via I2C communication. To mitigate
high-frequency noise resulting from engine vibration,
structure vibration, and mechanical nonlinearity dur-
ing sensor installation, the collected data are filtered
using the finite impulse response (FIR) method (Su-
laiman et al., 2022).
Figure 2: Structure of AOA/AOS sensors.
The sensor is connected to the UAV via a mount-
ing carbon tube that passes through the structural
housing. For UAVs with rear-mounted propellers, the
sensor can be installed on the nose of the UAV, provid-
ing direct access to undisturbed free airflow. This po-
sitioning helps avoid the effects of propeller-induced
airflow disturbances, thereby ensuring high data mea-
surement accuracy. However, for UAVs with front-
mounted propellers, the nose position becomes un-
suitable for sensor installation.
A feasible solution is to install the sensor beneath
one side of the wing, as illustrated in Fig. 3b. How-
ever, due to airflow disturbances caused by the pro-
peller and obstruction from the fuselage, this installa-
tion position inevitably affects the sensor’s measure-
ments and may even result in missing data.
2.2 AOA/AOS Estimation Method
In addition to the measurement from the AOA and
AOS sensors, the α and β can be estimated by fusing
inertial data collected by the flight controller. This
estimation process remains unaffected by the opera-
tional status of AOA/AOS sensors, making it a reli-
able and effective redundancy solution.
As an improvement of the extended Kalman filter,
the UKF offers superior accuracy for nonlinear distri-
bution statistics (Julier and Uhlmann, 1997). In this
paper, an estimation method based on the UKF was
adopted to estimate α and β using the inertial data.
The state estimation model for predicting the state at
An Adaptive Neuro-Fuzzy Inference Approach of AOA/AOS Data Fusion for Small Fixed-Wing UAV
907