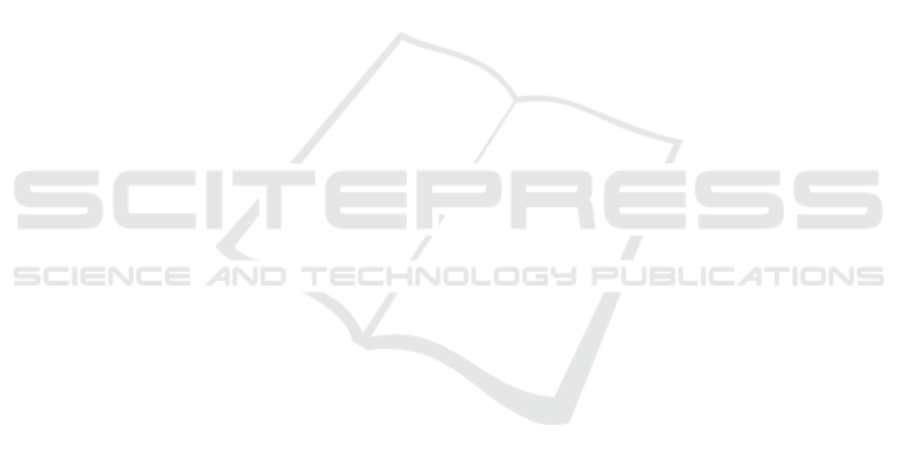
connection between target motion characteristics, re-
gional information, and target intentions so as to
test the ability of intelligent algorithms to recog-
nize multi-dimensional intentions in complex envi-
ronments. In addition, the dynamic time window
adaptation proposed in this paper dynamically ad-
justs the time window of the BiLSTM network based
on motion rules and the principle of reserving suf-
ficient disposal time while ensuring rapid intention
recognition of high-threat targets and accurate inten-
tion recognition of medium-threat and low-threat tar-
gets. Finally, based on a multi-dimensional intention
dataset for complex decision-making environments,
window division and intention recognition were per-
formed based on BiLSTM and dynamic time window
adaptation, verifying that the dynamic time window
can be dynamically processed while ensuring the ac-
curacy of intention recognition.
In future work, we would like to take the drones’
types and features that can be obtained in reality to
expand the feature space set. By establishing time
window division rules and threat ranking indicators
for different types of drones, we can make full use of
detectable information for faster and more accurate
intention recognition. How to dynamically adjust the
time window division and feature selection according
to the comprehensive motion state of all targets is also
an important direction for future work. In addition,
we would like to expand the intention space set based
on more realistic complex decision-making environ-
ments, using manual reasoning and system-derived
methods so that the system can more accurately iden-
tify the intentions of multiple types of targets in more
real scenarios.
REFERENCES
Cai, Z., Wang, Y., Jiang, Q., Gui, G., and Sha, J. (2024). To-
ward intelligent lightweight and efficient uav identifi-
cation with rf fingerprinting. IEEE Internet of Things
Journal.
Fraser, B., Perrusqu
´
ıa, A., Panagiotakopoulos, D., and Guo,
W. (2023). A deep mixture of experts network for
drone trajectory intent classification and prediction us-
ing non-cooperative radar data. In 2023 IEEE Sym-
posium Series on Computational Intelligence (SSCI),
pages 1–6. IEEE.
Kaza, K., Mehta, V., Azad, H., Bolic, M., and Mantegh, I.
(2024). An intent modeling and inference framework
for autonomous and remotely piloted aerial systems.
arXiv preprint arXiv:2409.08472.
Liang, J., Ahmad, B. I., Jahangir, M., and Godsill,
S. (2021). Detection of malicious intent in non-
cooperative drone surveillance. In 2021 Sensor Signal
Processing for Defence Conference (SSPD), pages 1–
5. IEEE.
Lof
`
u, D., Di Gennaro, P., Tedeschi, P., Di Noia, T., and
Di Sciascio, E. (2023). Uranus: Radio frequency
tracking, classification and identification of unmanned
aircraft vehicles. IEEE Open Journal of Vehicular
Technology.
Siami-Namini, S., Tavakoli, N., and Namin, A. S. (2019).
The performance of lstm and bilstm in forecasting
time series. In 2019 IEEE International Conference
on Big Data (Big Data), pages 3285–3292.
Teng, F., Guo, X., Song, Y., and Wang, G. (2021). An air
target tactical intention recognition model based on
bidirectional gru with attention mechanism. IEEE Ac-
cess, 9:169122–169134.
Wang, C., Meng, L., Gao, Q., Wang, T., Wang, J., and
Wang, L. (2024). A target sensing and visual track-
ing method for countering unmanned aerial vehicle
swarm. IEEE Sensors Journal.
Xue, C., Li, T., and Li, Y. (2024). Radio frequency based
distributed system for noncooperative uav classifica-
tion and positioning. Journal of Information and In-
telligence, 2(1):42–51.
Yang, Y., Yang, F., Sun, L., Xiang, T., and Lv, P. (2023).
Echoformer: Transformer architecture based on radar
echo characteristics for uav detection. IEEE Sensors
Journal, 23(8):8639–8653.
Yi, P., Yang, Y., and Lin, Z. (2024). Cooperative opponent
intention recognition based on trajectory analysis by
distributed fractional bayesian inference. In 2024 6th
International Conference on Electronic Engineering
and Informatics (EEI), pages 1551–1555. IEEE.
Yun, J., Anderson, D., and Fioranelli, F. (2023a). Estima-
tion of drone intention using trajectory frequency de-
fined in radar’s measurement phase planes. IET Radar,
Sonar & Navigation, 17(9):1327–1341.
Yun, J., Shin, H.-S., and Tsourdos, A. (2023b). Perime-
ter intrusion prediction method using trajectory fre-
quency and naive bayes classifier. In 2023 23rd In-
ternational Conference on Control, Automation and
Systems (ICCAS), pages 1610–1615. IEEE.
Zhang, H., Li, T., Li, Y., Li, J., Dobre, O. A., and Wen,
Z. (2023). Rf-based drone classification under com-
plex electromagnetic environments using deep learn-
ing. IEEE Sensors Journal, 23(6):6099–6108.
ICEIS 2025 - 27th International Conference on Enterprise Information Systems
920