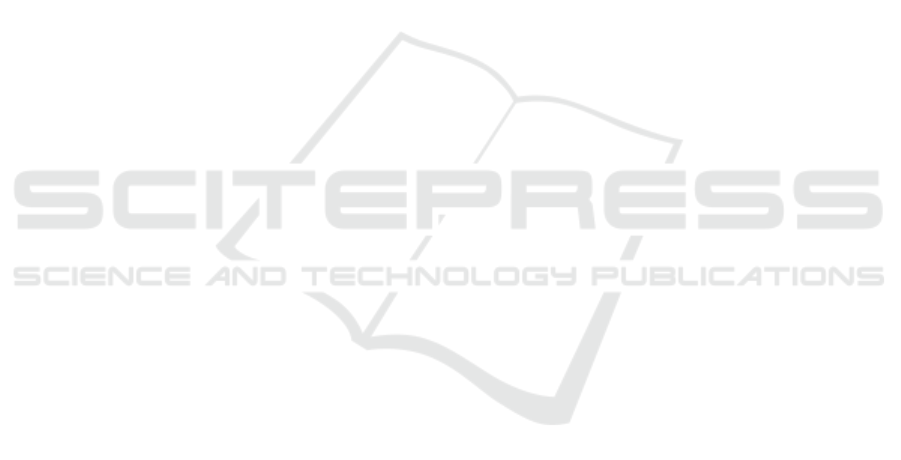
ing throughout workflows. Additionally, we will ex-
tend our case study to a larger-scale assembly setting
to demonstrate the scalability of our approach. We
plan to conduct a broader evaluation of the transfer-
ability from goal models to BPMNs in more complex,
industry-relevant scenarios, such as in the automotive
or aerospace industries, where task dependencies in
HRCA are more intricate. Finally, we plan to auto-
mate the overall framework using model-driven de-
velopment methods.
During the evaluation of our truck assembly, it
was realized that conclusions can be drawn not only
from the goal models to the BPMN, but also from the
refinement of the BPMN to a redesign of the goal
model. This creates a bidirectional iterative process
where both goal models and BPMN are continuously
improved. By expanding this methodology, we aim
to enhance the efficiency and effectiveness of HRCA,
ensuring that human operators and robots collaborate
more seamlessly to achieve the goals of modern man-
ufacturing.
REFERENCES
Amyot, D., Akhigbe, O., Baslyman, M., Ghanavati, S.,
Ghasemi, M., Hassine, J., Lessard, L., Mussbacher,
G., Shen, K., and Yu, E. (2022). Combining goal mod-
elling with business process modelling: Two decades
of experience with the user requirements notation
standard. Enterprise Modelling and Information Sys-
tems Architectures (EMISAJ), 17:2–1.
B
¨
ohm, B., B
¨
ohm, W., Daun, M., Hayward, A., Kranz, S.,
Regnat, N., Schr
¨
ock, S., Stierand, I., Vogelsang, A.,
Vollmar, J., Voss, S., Weyer, T., and Wortmann, A.
(2021). Engineering of collaborative embedded sys-
tems. In B
¨
ohm, W., Broy, M., Klein, C., Pohl, K.,
Rumpe, B., and Schr
¨
ock, S., editors, Model-Based En-
gineering of Collaborative Embedded Systems, pages
15–48. Springer.
Bourr, K., Corradini, F., Pettinari, S., Re, B., Rossi, L., and
Tiezzi, F. (2021). Disciplined use of bpmn for mission
modeling of multi-robot systems. In Working Confer-
ence on the Practice of Enterprise Modelling.
Chen, F., Sekiyama, K., Sasaki, H., Huang, J., Sun, B.,
and Fukuda, T. (2011). Assembly strategy modeling
and selection for human and robot coordinated cell as-
sembly. In 2011 IEEE/RSJ International Conference
on Intelligent Robots and Systems, pages 4670–4675.
IEEE.
Chen, P. (2007). Goal-oriented business process monitor-
ing. PhD thesis, Citeseer.
Cherubini, A., Passama, R., Crosnier, A., Lasnier, A.,
and Fraisse, P. (2016). Collaborative manufacturing
with physical human–robot interaction. Robotics and
Computer-Integrated Manufacturing, 40:1–13.
Corradini, F., Pettinari, S., Re, B., Rossi, L., and Tiezzi,
F. (2023). A bpmn-driven framework for multi-robot
system development. Robotics and Autonomous Sys-
tems, 160.
Daniel Amyot, O. A. (2022). Combining goal mod-
elling with business process modelling. Enter-
prise Modelling and Information Systems Architec-
tures (EMISAJ).
Daun, M., Manjunath, M., and Jesus Raja, J. (2023). Safety
analysis of human robot collaborations with grl goal
models. In International Conference on Conceptual
Modeling, pages 317–333. Springer.
Daun, M., Stenkova, V., Krajinski, L., Brings, J.,
Bandyszak, T., and Weyer, T. (2019). Goal model-
ing for collaborative groups of cyber-physical systems
with grl: reflections on applicability and limitations
based on two studies conducted in industry. In Pro-
ceedings of the 34th ACM/SIGAPP Symposium on Ap-
plied Computing, pages 1600–1609.
Est
´
evez, E. and Marcos, M. (2011). Model-based validation
of industrial control systems. IEEE Transactions on
Industrial Informatics, 8(2):302–310.
Fernandes, J., Reis, J., Mel
˜
ao, N., Teixeira, L., and Amorim,
M. (2021). The role of industry 4.0 and bpmn in the
arise of condition-based and predictive maintenance:
A case study in the automotive industry. Applied Sci-
ences, 11(8):3438.
Grau, A., Indri, M., Bello, L. L., and Sauter, T. (2020).
Robots in industry: The past, present, and future of a
growing collaboration with humans. IEEE Industrial
Electronics Magazine, 15(1):50–61.
Horkoff, J. and Yu, E. (2011). Analyzing goal models: dif-
ferent approaches and how to choose among them. In
Proceedings of the 2011 ACM Symposium on Applied
Computing, pages 675–682.
Jesus Raja, J., Manjunath, M., and Daun, M. (2024). To-
wards a goal-oriented approach for engineering digital
twins of robotic systems. In ENASE, pages 466–473.
Kem
´
eny, Z., V
´
ancza, J., Wang, L., and Wang, X. V.
(2021). Human–robot collaboration in manufacturing:
A multi-agent view. In Advanced human-robot collab-
oration in manufacturing, pages 3–41. Springer.
Kranz, P., Elbishbishy, S., Jesus Raja, J., and Daun, M.
(2024). Supporting safety assessment in human-
robot collaboration using process models. In ER2024:
Companion Proceedings of the 43rd International
Conference on Conceptual Modeling: ER Forum,
Special Topics,Posters and Demos, October 28-31,
2024, Pittsburgh, Pennsylvania, USA, pages 136–140.
CEUR Workshop Proceedings.
Lestingi, L. and Longoni, S. (2016). Hrc-team: A model-
driven approach to formal verification and deployment
of collaborative robotic applications.
Malik, A. A. and Bilberg, A. (2019). Developing a ref-
erence model for human–robot interaction. Interna-
tional Journal on Interactive Design and Manufactur-
ing (IJIDeM), 13(4):1541–1547.
Manjunath, M., Jesus Raja, J., and Daun, M. (2024). Early
model-based safety analysis for collaborative robotic
systems. IEEE Transactions on Automation Science
and Engineering.
Combining Goal and Process Models for the Specification of Human-Robot Collaborations
227