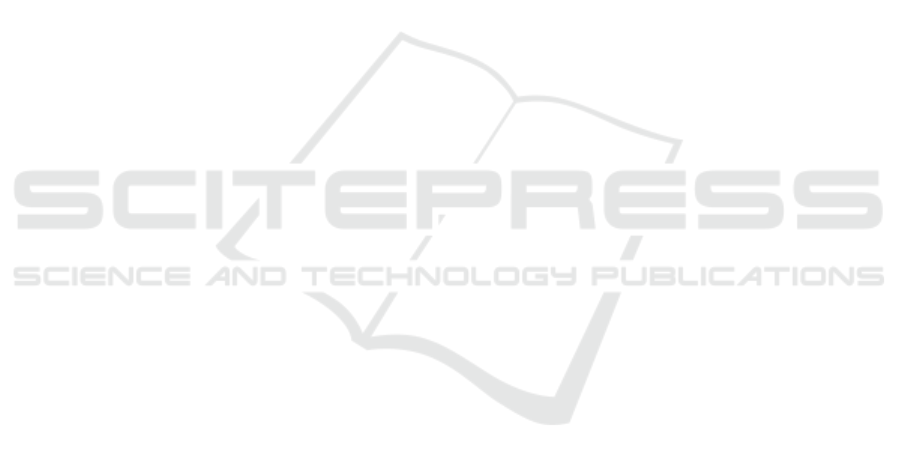
proposed Transformer architecture on generic time
series classification tasks. Additionally, creating a
public time series dataset for customer churn would
facilitate direct comparisons between models.
ACKNOWLEDGEMENTS
This work was partially funded by Telenor Sverige
AB.
REFERENCES
Ahlstrand, J., Boldt, M., Borg, A., and Grahn, H. (2024).
Predicting B2B customer churn using a time series ap-
proach. In 2024 Fifth International Conference on In-
telligent Data Science Technologies and Applications
(IDSTA), pages 44–51.
Ahmadi, H. and Hasheminejad, S. M. H. (2021). A new
time series approach in churn prediction with discrim-
inatory intervals. In 2021 11th International Confer-
ence on Computer Engineering and Knowledge (IC-
CKE), pages 508–513.
Ahn, J., Hwang, J., Kim, D., Choi, H., and Kang, S. (2020).
A survey on churn analysis in various business do-
mains. IEEE Access, 8:220816–220839.
Angelopoulos, A. N. and Bates, S. (2021). A gen-
tle introduction to conformal prediction and
distribution-free uncertainty quantification.
https://arxiv.org/abs/2107.07511v6.
Ascarza, E. (2018). Retention futility: Targeting high-risk
customers might be ineffective. Journal of Marketing
Research, 55(1):80–98.
Ascarza, E. and Hardie, B. G. S. (2013). A joint model
of usage and churn in contractual settings. Marketing
Science, 32(4):570–590.
Boström, H. (2022). crepes: a python package for gener-
ating conformal regressors and predictive systems. In
Johansson, U., Boström, H., An Nguyen, K., Luo, Z.,
and Carlsson, L., editors, Proceedings of the Eleventh
Symposium on Conformal and Probabilistic Predic-
tion and Applications, volume 179 of Proceedings of
Machine Learning Research. PMLR.
Chen, K., Hu, Y.-H., and Hsieh, Y.-C. (2015). Predicting
customer churn from valuable b2b customers in the
logistics industry: a case study. Information Systems
and e-Business Management, 13(3):475–494.
Devriendt, F., Berrevoets, J., and Verbeke, W. (2021). Why
you should stop predicting customer churn and start
using uplift models. Information Sciences, 548:497–
515.
Fawcett, T. (2006). An introduction to ROC analysis. Pat-
tern Recognition Letters, 27(8):861–874.
Gal, Y. and Ghahramani, Z. (2016). Dropout as a bayesian
approximation: Representing model uncertainty in
deep learning. In Balcan, M. F. and Weinberger,
K. Q., editors, Proceedings of The 33rd International
Conference on Machine Learning, volume 48 of Pro-
ceedings of Machine Learning Research, pages 1050–
1059, New York, New York, USA. PMLR.
Gattermann-Itschert, T. and Thonemann, U. W. (2022).
Proactive customer retention management in a non-
contractual b2b setting based on churn prediction with
random forests. Industrial Marketing Management,
107:134–147.
Gür Ali, O. and Arıtürk, U. (2014). Dynamic churn predic-
tion framework with more effective use of rare event
data: The case of private banking. Expert Systems with
Applications, 41(17):7889–7903.
Hutto, C. and Gilbert, E. (2014). VADER: A parsimonious
rule-based model for sentiment analysis of social me-
dia text. In Proceedings of the International AAAI
Conference on Web and Social Media, volume 8,
pages 216–225. Number: 1.
Islam, S., Elmekki, H., Elsebai, A., Bentahar, J., Drawel,
N., Rjoub, G., and Pedrycz, W. (2024). A com-
prehensive survey on applications of transformers for
deep learning tasks. Expert Systems with Applications,
241:122666.
Janssens, B., Bogaert, M., Bagué, A., and Van den Poel,
D. (2022). B2boost: instance-dependent profit-driven
modelling of b2b churn. Annals of Operations Re-
search, 341(1):267–293.
Kumar, S., Mariyasagayam, N., and Nonaka, Y. (2024).
Evaluating the effect of leading indicators in customer
churn prediction. In Borah, M. D., Laiphrakpam,
D. S., Auluck, N., and Balas, V. E., editors, Big Data,
Machine Learning, and Applications, Lecture Notes
in Electrical Engineering, pages 327–340. Springer
Nature.
Mena, C. G., De Caigny, A., Coussement, K., De Bock,
K. W., and Lessmann, S. (2019). Churn pre-
diction with sequential data and deep neural net-
works. a comparative analysis. arXiv:1909.11114,
http://arxiv.org/abs/1909.11114.
Mena, G., Coussement, K., De Bock, K., De Caigny, A.,
and Lessmann, S. (2024). Exploiting time-varying
RFM measures for customer churn prediction with
deep neural networks. Annals of Operations Research,
339(1):765–787.
Neslin, S. A., Gupta, S., Kamakura, W., Lu, J., and Mason,
C. H. (2006). Defection detection: Measuring and un-
derstanding the predictive accuracy of customer churn
models. Journal of Marketing Research, 43(2):204–
211.
Park, W. and Ahn, H. (2022). Not all churn customers are
the same: Investigating the effect of customer churn
heterogeneity on customer value in the financial sec-
tor. Sustainability, 14(19):12328.
Paszke, A., Gross, S., Massa, F., Lerer, A., Bradbury, J.,
Chanan, G., Killeen, T., Lin, Z., Gimelshein, N.,
Antiga, L., Desmaison, A., Kopf, A., Yang, E., De-
Vito, Z., Raison, M., Tejani, A., Chilamkurthy, S.,
Steiner, B., Fang, L., Bai, J., and Chintala, S. (2019).
PyTorch: An imperative style, high-performance deep
learning library. In Advances in Neural Information
Processing Systems, volume 32. Curran Associates,
Inc.
ICEIS 2025 - 27th International Conference on Enterprise Information Systems
570