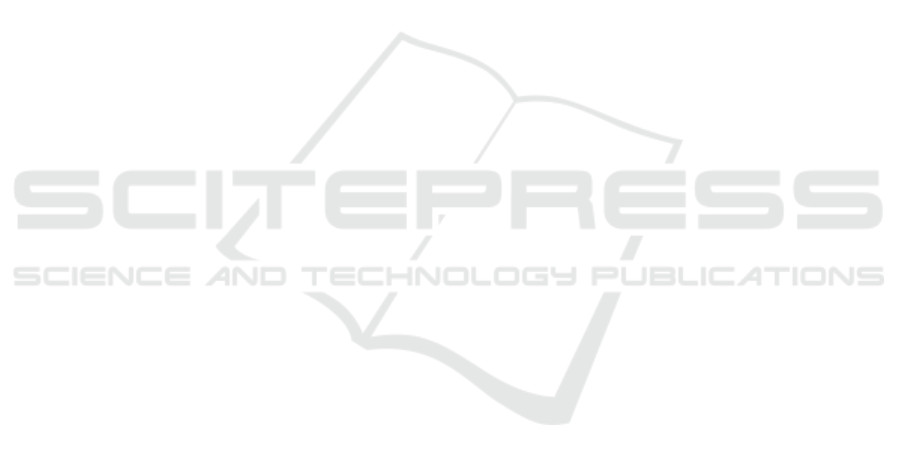
Detroja, K., Bhensdadia, C., and Bhatt, B. S. (2023). A
survey on relation extraction. Intelligent Systems with
Applications, 19:200244.
Devlin, J., Chang, M.-W., Lee, K., and Toutanova, K.
(2018). Bert: Pre-training of deep bidirectional trans-
formers for language understanding. arXiv preprint
arXiv:1810.04805.
Dumas, M., La Rosa, M., Mendling, J., Reijers, H. A., et al.
(2018). Fundamentals of business process manage-
ment, volume 2. Springer.
Dymora, P., Koryl, M., and Mazurek, M. (2019). Process
discovery in business process management optimiza-
tion. Information, 10(9):270.
Epure, E. V., Mart
´
ın-Rodilla, P., Hug, C., Deneck
`
ere, R.,
and Salinesi, C. (2015). Automatic process model dis-
covery from textual methodologies. In 2015 IEEE 9th
International Conference on Research Challenges in
Information Science (RCIS), pages 19–30. IEEE.
Ferreira, R. C. B., Thom, L. H., and Fantinato, M. (2017). A
semi-automatic approach to identify business process
elements in natural language texts. In International
Conference on Enterprise Information Systems, vol-
ume 2, pages 250–261. SCITEPRESS.
Fionda, V. and Guzzo, A. (2020). Control-flow modeling
with declare: Behavioral properties, computational
complexity, and tools. IEEE Transactions on Knowl-
edge & Data Engineering, 32(05):898–911.
Friedrich, F., Mendling, J., and Puhlmann, F. (2011). Pro-
cess model generation from natural language text. In
Advanced Information Systems Engineering: 23rd In-
ternational Conference, CAiSE 2011, London, UK,
June 20-24, 2011. Proceedings 23, pages 482–496.
Springer.
Grohs, M., Abb, L., Elsayed, N., and Rehse, J.-R. (2023).
Large language models can accomplish business pro-
cess management tasks. In International Conference
on Business Process Management, pages 453–465.
Springer.
Honkisz, K., Kluza, K., and Wi
´
sniewski, P. (2018). A con-
cept for generating business process models from nat-
ural language description. In Knowledge Science, En-
gineering and Management: 11th International Con-
ference, KSEM 2018, Changchun, China, August 17–
19, 2018, Proceedings, Part I 11, pages 91–103.
Springer.
Klievtsova, N., Benzin, J.-V., Kampik, T., Mangler, J., and
Rinderle-Ma, S. (2023). Conversational process mod-
elling: State of the art, applications, and implications
in practice. arXiv preprint arXiv:2304.11065.
Kourani, H., Berti, A., Schuster, D., and van der Aalst,
W. M. (2024). Process modeling with large language
models. In International Conference on Business Pro-
cess Modeling, Development and Support, pages 229–
244. Springer.
Lample, G., Ballesteros, M., Subramanian, S., Kawakami,
K., and Dyer, C. (2016). Neural architectures
for named entity recognition. arXiv preprint
arXiv:1603.01360.
Li, J., Sun, A., Han, J., and Li, C. (2020). A survey on deep
learning for named entity recognition. IEEE transac-
tions on knowledge and data engineering, 34(1):50–
70.
L
´
opez, H. A., Strømsted, R., Niyodusenga, J.-M., and Mar-
quard, M. (2021). Declarative process discovery:
Linking process and textual views. In Nurcan, S.
and Korthaus, A., editors, Intelligent Information Sys-
tems, pages 109–117, Cham. Springer International
Publishing.
Obendorf, H. (2009). Minimalism: designing simplicity.
Springer Science & Business Media.
Pennington, J., Socher, R., and Manning, C. D. (2014).
Glove: Global vectors for word representation. In
Proceedings of the 2014 conference on empirical
methods in natural language processing (EMNLP),
pages 1532–1543.
Qian, C., Wen, L., Kumar, A., Lin, L., Lin, L., Zong, Z.,
Li, S., and Wang, J. (2020). An approach for pro-
cess model extraction by multi-grained text classifica-
tion. In Advanced Information Systems Engineering:
32nd International Conference, CAiSE 2020, Greno-
ble, France, June 8–12, 2020, Proceedings 32, pages
268–282. Springer.
Sch
¨
utzenmeier, N., K
¨
appel, M., Petter, S., and Jablonski,
S. (2021). Upper-bounded model checking for declar-
ative process models. In The Practice of Enterprise
Modeling: 14th IFIP WG, pages 195–211. Springer.
van der Aa, H., Carmona Vargas, J., Leopold, H., Mendling,
J., and Padr
´
o, L. (2018). Challenges and opportunities
of applying natural language processing in business
process management. In COLING 2018: The 27th
International Conference on Computational Linguis-
tics: Proceedings of the Conference: August 20-26,
2018 Santa Fe, New Mexico, USA, pages 2791–2801.
Association for Computational Linguistics.
Van der Aa, H., Di Ciccio, C., Leopold, H., and Reijers,
H. A. (2019). Extracting declarative process mod-
els from natural language. In Advanced Information
Systems Engineering: 31st International Conference,
CAiSE 2019, Rome, Italy, June 3–7, 2019, Proceed-
ings 31, pages 365–382. Springer.
Yuan, A., Ippolito, D., Nikolaev, V., Callison-Burch, C.,
Coenen, A., and Gehrmann, S. (2021). Synthbio: A
case study in faster curation of text datasets. In Thirty-
fifth Conference on Neural Information Processing
Systems Datasets and Benchmarks Track (Round 2).
Zhao, X., Deng, Y., Yang, M., Wang, L., Zhang, R., Cheng,
H., Lam, W., Shen, Y., and Xu, R. (2024). A com-
prehensive survey on relation extraction: Recent ad-
vances and new frontiers. ACM Computing Surveys,
56(11):1–39.
ICEIS 2025 - 27th International Conference on Enterprise Information Systems
782