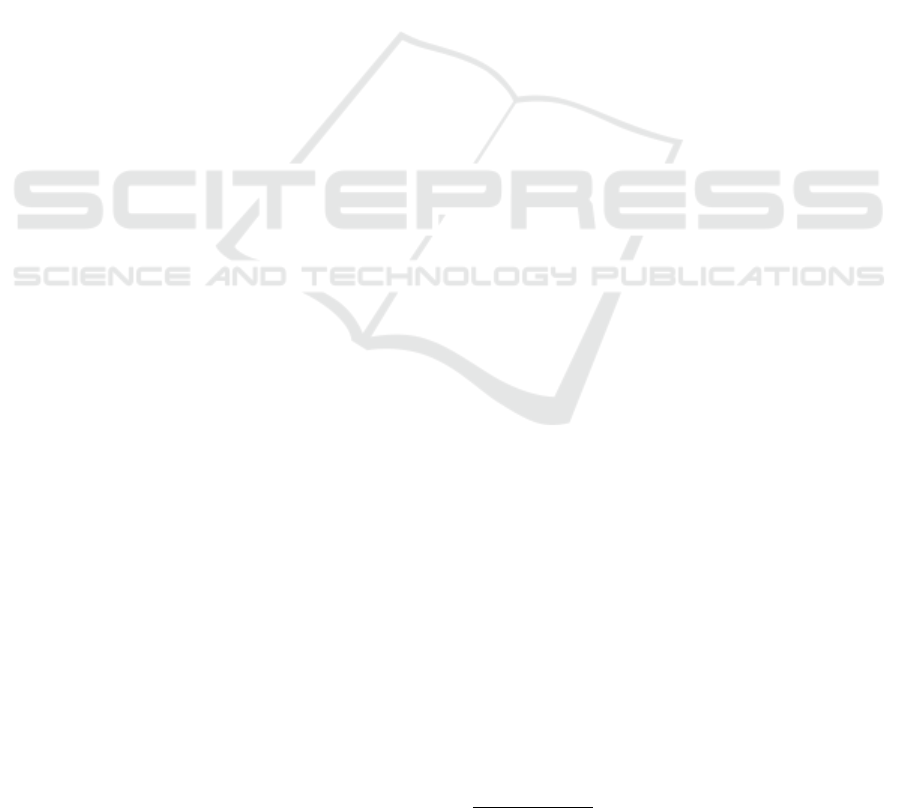
3.6 Education Framework
The game’s design is grounded in experiential learn-
ing theory, which posits that individuals learn best
by transforming theoretical knowledge into practical
skills, thereby enhancing the likelihood of real-world
application (Kolb, 1984). The narrative-driven design
of RWR maps seamlessly onto Kolb’s (Kolb, 1984)
Experiential Learning Cycle. Players are introduced
to realistic scam scenarios (Concrete Experience), en-
couraged to reflect on their choices through directed
feedback (Reflective Observation), equipped with ab-
stract principles for scam detection via in-game con-
tent (Abstract Conceptualization), and challenged to
apply these strategies in progressively complex situ-
ations (Active Experimentation). This alignment en-
sures that the learning experience is not only engag-
ing but also theoretically grounded, promoting deeper
knowledge retention and real-world applicability. Re-
search has further shown the efficacy of experiential
learning in educational settings, with a study suggest-
ing that experiential learning activities have signifi-
cantly improved student’s ability to apply theoreti-
cal concepts in practice (Sarah Yardley and Dornan,
2012). Similarly, a meta-analysis has demonstrated
that interactive learning methods, such as simula-
tions and role-playing, effectively enhance learners’
critical thinking and problem-solving skills (Rogers,
2015). In the context of scam prevention, interactive
learning tools have been shown to increase aware-
ness and preparedness. With academic literature on
game design framework revealing that mobile games
designed to teach phishing awareness led to a signifi-
cant improvement in users’ ability to identify fraudu-
lent emails (Arachchilage and Love, 2013).
The following were implemented to achieve the
learning objectives:
1. Concise, Static Newspaper Content. In the
Newspaper of the Main Scene, the team has in-
cluded extremely concise information about dif-
ferent types of scams. By focusing only on: (i)
What are these scams; (ii) How they manifest; and
(iii) How to avoid them; Players can read about
scams and refer to this for gameplay aid.
2. Scam Detection as Core Gameplay. The core
gameplay mechanic replicates real-life scams, al-
lowing players to train their “scam detection” in-
stincts; this lets them respond to actual scams bet-
ter. The game is also intentionally fast-paced, to
replicate real life conditions - where a player is
often presented with scams in their hectic lives.
Lastly, the game also simulates for a player what
happens in reality should they fall for a scam -
they lose more money than they’ve earned.
3. Directed, Instantaneous Feedback. The game
also provides directed, informative feedback im-
mediately after the player makes a decision, i.e.
if a player falls for a scam, the game explains
to the player in the post-decision prompt about
why the Email or SMS was a scam and how they
could have detected it. This directed feedback al-
lows players to be more aware of the scams they
are likely to fall for, and how they can detect and
avoid it in the future.
4 EVALUATION FRAMEWORK
The evaluation framework assesses RWR’s effective-
ness in educating players on scam awareness and pre-
vention strategies. This section outlines the key met-
rics and the methodology used to gauge the educa-
tional impact of the game.
4.1 Evaluation Metrics
To measure RWR’s impact quantitatively, our team
has developed 45 key metrics (shown in Table 1l) that
aligns with both educational and interactive learning
goals in three broad categories:
1. Scam Detection Skills, which focuses on evaluat-
ing players’ abilities to recognize and respond to
scams. These include the accuracy of scam iden-
tification, frequency of legitimate emails correctly
identified, the different platforms (e-mail or SMS)
being engaged, and the total number of emails and
SMS sent within an in-game day;
2. Time Element, which analyzes the time taken for
player to respond to an email or SMS event gener-
ated, number of in-game days that the player has
spent within the game (as retention rate), and time
taken to respond correctly. These provide insights
into how time constraints influence learning re-
tention and decision-making speed, which com-
plements with the Scam Detection Skills category
metrics and is essential in real-life scam preven-
tion where quick decisions are needed;
3. Overall Gameplay, which evaluates the broader
aspects of player engagement by tallying whether
the player has been able to achieve the goal objec-
tive easily with the UI/UX design and scenario de-
velopment. These metrics ensure that the game re-
mains user-friendly and engaging, as a high score
indicates that the game has provided an effective
and enjoyable learning experience for players.
1
Available at https://metaverse.comp.nus.edu.sg/projects/rwr.
CSEDU 2025 - 17th International Conference on Computer Supported Education
584