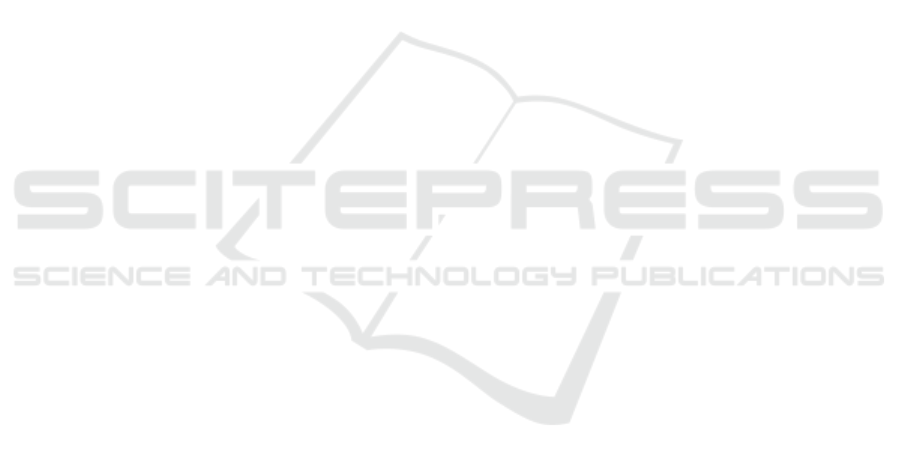
results show that with time the pattern changes, thus
reducing the capabilities of the obsolete model to cor-
rectly assess the predictions. This indicates the need
for constant updates on model training to optimize re-
call.
Consistent analysis identifies customer inactivity
as a strong predictor of churn. Long periods without
logins (F11), job postings (F15), or positive interac-
tions (F31) strongly indicate higher churn risk.
Addressing B2B churn in HR Tech is challeng-
ing. To our knowledge, there are few studies specifi-
cally on HR Tech churn, highlighting the novelty and
importance of our work. Our findings offer valuable
insights into HR Tech churn factors, enabling tailored
retention strategies. By focusing on proactive engage-
ment and addressing inactivity, HR Tech companies
can improve customer retention.
6 CONCLUSIONS AND FUTURE
WORKS
This study investigated churn prediction in a B2B
context using biweekly data and a SVM model with a
linear kernel. Feature selection through SFFS, com-
bined with interpretability analysis based on the co-
efficient values of each feature, enabled the identifi-
cation of key churn drivers and the development of
actionable insights for retention strategies.
The model’s accuracy stability across the analyzed
biweekly periods demonstrates its robustness to tem-
poral data variations, a crucial aspect in dynamic envi-
ronments like B2B. Additionally, the consistent mini-
mization of false positives in four out of five biweekly
periods reduces the cost of unnecessary interventions,
optimizing retention resources.
The coefficient analysis of each feature revealed
valuable insights into customer behavior and the
factors influencing churn. Platform inactivity, ex-
tended periods without creating new job postings, and
the absence of positive occurrences emerged as the
strongest predictors of churn. These findings align
with existing literature emphasizing the importance
of customer engagement and positive service experi-
ences for retention. The analysis also highlighted the
influence of other features, such as inactivity in the
engineering functionality and profile creation, point-
ing to the need for further investigation into the rela-
tionship between evaluation frequency and churn.
Despite stable accuracy, our results indicate that
the recall falls over customer necessities. Because
of this, it may be necessary to periodically retrain
the model for more consistent results. The discrep-
ancy suggests potential overfitting in the traditional
approach and underscores the importance of temporal
validation for a more realistic assessment of model
performance.
This study contributes to B2B churn prediction by
identifying key churn drivers, demonstrating the value
of coefficient-based feature analysis for model inter-
pretability, and proposing targeted retention strate-
gies, such as encouraging frequent logins, promoting
key functionalities, and fostering positive platform in-
teractions. For future research, exploring alternative
machine learning models, including ensemble meth-
ods (Random Forest, Gradient Boosting) and Deep
Learning approaches, could provide valuable perfor-
mance comparisons with SVM. Additionally, incor-
porating class balancing techniques, such as Class
Weight adjustments, SMOTE, and Random Over and
Under-Sampling, may improve model effectiveness
by addressing data imbalance. Further investigation
of new predictive features, such as detailed behavioral
metrics, contextual company characteristics, and sea-
sonal factors, could enhance model accuracy and pro-
vide deeper insights into customer retention dynam-
ics.
REFERENCES
A., S. and D., C. (2016). A Survey on Customer Churn Pre-
diction using Machine Learning Techniques. Interna-
tional Journal of Computer Applications, 154:13–16.
Athanassopoulos, A. D. (2000). Customer satisfaction cues
to support market segmentation and explain switching
behavior. Journal of Business Research, 47(3):191–
207.
Cheng, Q., Varshney, P. K., and Arora, M. K. (2006). Lo-
gistic regression for feature selection and soft classifi-
cation of remote sensing data. IEEE Geoscience and
Remote Sensing Letters, 3(4):491–494.
Cortes, C. and Vapnik, V. (1995). Support-vector networks.
Machine Learning, 20(3):273–297.
Coussement, K. and Van den Poel, D. (2008). Churn predic-
tion in subscription services: An application of sup-
port vector machines while comparing two parameter-
selection techniques. Expert Systems with Applica-
tions, 34(1):313–327.
Drummond, C. and Holte, R. (2003). C4.5, class imbalance,
and cost sensitivity: Why under-sampling beats over-
sampling. Proceedings of the ICML’03 Workshop on
Learning from Imbalanced Datasets.
Gordini, N. and Veglio, V. (2017). Customers churn pre-
diction and marketing retention strategies. An appli-
cation of support vector machines based on the AUC
parameter-selection technique in B2B e-commerce in-
dustry. Industrial Marketing Management, 62:100–
107.
Kamakura, W., Mela, C. F., Ansari, A., Bodapati, A., Fader,
P., Iyengar, R., Naik, P., Neslin, S., Sun, B., Verhoef,
ICEIS 2025 - 27th International Conference on Enterprise Information Systems
580