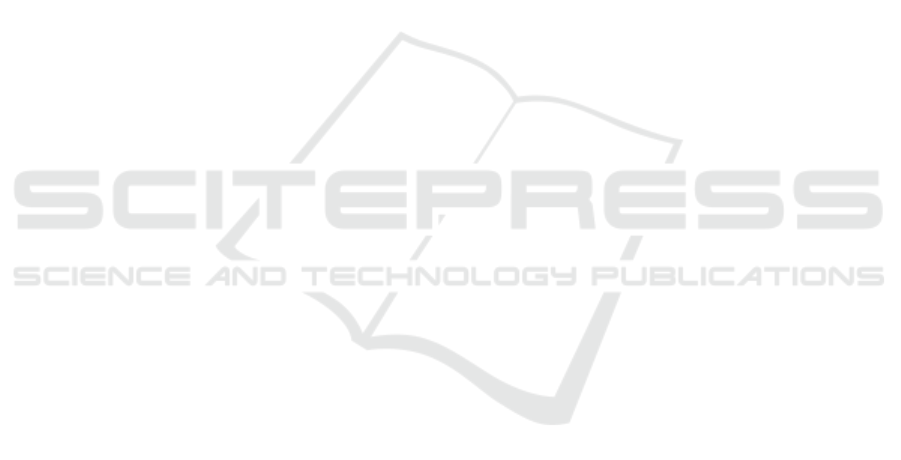
A key constraint is the focus on single-session
recommendations, whereas real-world users interact
with music over time. Temporal models would be
necessary to track evolving preferences, and collab-
orative filtering could enhance recommendations by
incorporating community-driven insights. Address-
ing these challenges would improve scalability, ro-
bustness, and user satisfaction.
Despite these limitations, this study represents a
significant step forward in hybrid music recommen-
dation systems, effectively balancing personalization
and diversity while maintaining computational effi-
ciency. These findings establish a foundation for fu-
ture research, enabling more adaptive and enriched
user experiences in music recommendation.
ACKNOWLEDGEMENTS
This paper was financed in part by the Coordenac¸
˜
ao
de Aperfeic¸oamento de Pessoal de N
´
ıvel Superior -
Brazil (CAPES) - Finance Code 001, Fundac¸
˜
ao de
Amparo a Ci
ˆ
encia e Tecnologia do Estado de Pernam-
buco (FACEPE), the Conselho Nacional de Desen-
volvimento Cient
´
ıfico e Tecnol
´
ogico (CNPq) - Brazil-
ian research agencies.
REFERENCES
Aldi, F., Hadi, F., Rahmi, N. A., and Defit, S. (2023). Stan-
dardscaler’s potential in enhancing breast cancer accu-
racy using machine learning. JAETS, 5(1):401–413.
Bagal, V., Aggarwal, R., Vinod, P. K., and Priyakumar,
U. D. (n.d.). Liggpt: Molecular generation using a
transformer-decoder model.
Borges, H. B. (n.d.). Reduc¸
˜
ao de dimensionalidade em
bases de dados de express
˜
ao g
ˆ
enica. Technical report
or unpublished work.
Cui, M. (n.d.). Introduction to the k-means clustering algo-
rithm based on the elbow method.
Defferrard, M., Benzi, K., Vandergheynst, P., and Bresson,
X. (2017). Fma: A dataset for music analysis. arXiv.
Dehak, N., Dehak, R., Glass, J., Reynolds, D., and Kenny,
P. (n.d.). Cosine similarity scoring without score nor-
malization techniques.
Department of Computer Science and Engineering, The
Maharaja Sayajirao University of Baroda, India
(2019). Research advances in computer science and
engineering. International Journal of Computer Sci-
ence. et al.
Domingues, M. A., Trevisani, P. L., Duarte, F. M. N., and
Cunha, A. M. (2012). Combining usage and content
in an online music recommendation system for mu-
sic in the long-tail. In Proceedings of the 21st Inter-
national Conference on World Wide Web, pages 925–
930, Lyon, France. ACM.
Godinho, A. and Vasconcelos, F. (n.d.). Recomendac¸
˜
ao mu-
sical para grupos baseada em modelo h
´
ıbrido.
Goto, M. (n.d.). Rwc music database: Music genre database
and musical instrument sound database.
Hartigan, J. A. and Wong, M. A. (1979). Algorithm as 136:
A k-means clustering algorithm. Applied Statistics,
28(1):100.
Huang, L., Qin, J., Zhou, Y., Zhu, F., Liu, L., and Shao,
L. (2020). Normalization techniques in training dnns:
Methodology, analysis and application. arXiv.
Pandya, M. (2024). Spotify tracks dataset. Accessed on
December 8, 2024.
Patro, S. G. K. and Sahu, K. K. (2015). Normalization:
A preprocessing stage. International Advanced Re-
search Journal in Science, Engineering and Technol-
ogy, 2(3):20–22.
Pola, G., Bujorianu, M. L., Lygeros, J., and Di Benedetto,
M. D. (2003). Stochastic hybrid models: An overview.
IFAC Proceedings Volumes, 36(6):45–50.
Roberts, A., Brooks, R., and Shipway, P. (2014). Internal
combustion engine cold-start efficiency: A review of
the problem, causes and potential solutions. Energy
Conversion and Management, 82:327–350.
Sharma, L. and Gera, A. (2013). A survey of recommenda-
tion system: Research challenges. International Jour-
nal of Engineering Trends and Technology.
Song, Y., Dixon, S., and Pearce, M. (2012). A survey of mu-
sic recommendation systems and future perspectives.
In Proceedings of the International Society for Mu-
sic Information Retrieval Conference (ISMIR), pages
395–400.
Vogels, T. P., Rajan, K., and Abbott, L. F. (2005). Neural
network dynamics. Annual Review of Neuroscience,
28(1):357–376.
Wu, L., Wang, J., Xie, Y., and He, X. (2024). A survey on
large language models for recommendation. arXiv.
Yoshii, K., Goto, M., Komatani, K., Ogata, T., and Okuno,
H. G. (2008). An efficient hybrid music recommender
system using an incrementally trainable probabilis-
tic generative model. IEEE Transactions on Audio,
Speech, and Language Processing, 16(2):435–447.
ICEIS 2025 - 27th International Conference on Enterprise Information Systems
342