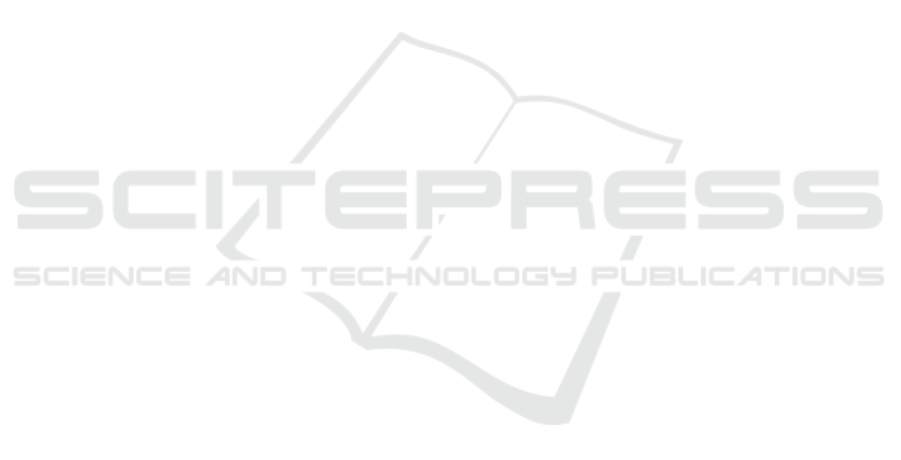
quantity when constructing training sets for anomaly
detection models.
Ultimately, this research contributes insights to
the field of fault detection in industrial systems, chal-
lenging conventional notions and paving the way
for more nuanced and effective anomaly detection
methodologies. The findings encourage a shift to-
ward a more adaptive and context-aware approach in
implementing anomaly detection techniques for di-
verse industrial applications. These insights provide
a strong foundation for future studies aimed at refin-
ing anomaly detection strategies and validating them
in real-world manufacturing settings.
ACKNOWLEDGEMENTS
The authors would like to thank the research team
GPCDA (Grupo de Pesquisa em Ci
ˆ
encia de Dados e
Analytics) at PPGEC-UPE for all the invaluable con-
tributions to the discussions and for their support. We
need also to thank the huge contribution of Meka-
tronik’s Team for sharing the simulation data and for
the opportunity to work on methodology and exper-
iment execution. And to thank Stellantis Goiana for
all the support and incentive. Gratitude is extended to
CAPES and CNPq for their financial support, without
which this work would not have been possible.
This paper was financed in part by the
Coordenac¸
˜
ao de Aperfeic¸oamento de Pessoal de
N
´
ıvel Superior - Brazil (CAPES) - Finance Code
001, Fundac¸
˜
ao de Amparo a Ci
ˆ
encia e Tecnologia do
Estado de Pernambuco (FACEPE), the Conselho Na-
cional de Desenvolvimento Cient
´
ıfico e Tecnol
´
ogico
(CNPq) - Brazilian research agencies.
REFERENCES
Ahmad, R. and Kamaruddin, S. (2012). An overview of
time-based and condition-based maintenance in in-
dustrial application. Computers &industrial engineer-
ing.
Albuquerque Filho, J. E. D., Brand
˜
ao, L. C. P., Fernandes,
B. J. T., and Maciel, A. M. A. (2022). A review of
neural networks for anomaly detection. IEEE Access,
10:112342–112367.
Ali, M. (2020). PyCaret: An open source, low-code ma-
chine learning library in Python. PyCaret version 2.3.
Buitinck, L., Louppe, G., Blondel, M., Pedregosa, F.,
Mueller, A., Grisel, O., Niculae, V., Prettenhofer, P.,
Gramfort, A., Grobler, J., Layton, R., VanderPlas, J.,
Joly, A., Holt, B., and Varoquaux, G. (2013). API de-
sign for machine learning software: experiences from
the scikit-learn project. In ECML PKDD Workshop:
Languages for Data Mining and Machine Learning,
pages 108–122.
Dalenogare, L., Benitez, G., Ayala, N., and ... (2018). The
expected contribution of industry 4.0 technologies for
industrial performance. International Journal of . . . .
Huang, J., Wen, J., Yoon, H., Pradhan, O., Wu, T., and
Neill, Z. O. (2022). Energy & Buildings Real vs
. simulated : Questions on the capability of simu-
lated datasets on building fault detection for energy
efficiency from a data-driven perspective. Energy &
Buildings, 259:111872.
Kang, Z., Catal, C., and Tekinerdogan, B. (2020). Machine
learning applications in production lines : A system-
atic literature review. Computers & Industrial Engi-
neering, 149(August):106773.
Kriegel, H., Schubert, M., and Zimek, A. (2008). Angle-
based outlier detection in high-dimensional data. Pro-
ceedings of the 14th ACM . . . .
Leite, D., Andrade, E., Rativa, D., and Maciel, A. M.
(2025). Fault Detection and Diagnosis in Industry 4.0:
A Review on Challenges and Opportunities. Sensors,
25(1):1–34.
Leite, D., Martins, A. J., Rativa, D., Oliveira, J. D., and
Maciel, A. (2022). An automated machine learning
approach for real-time fault detection and diagnosis.
Sensors.
Ramesh, P., Dutta, S., Neog, S., Baishya, P., and ... (2020).
Implementation of predictive maintenance systems in
remotely located process plants under industry 4.0
scenario. . . . Engineering: In Honor of . . . .
Sakib, N. and Wuest, T. (2018). Challenges and opportuni-
ties of condition-based predictive maintenance: a re-
view. Procedia cirp.
Tsui, K., Chen, N., Zhou, Q., Hai, Y., and Wang, W. (2015).
Prognostics and health management: A review on data
driven approaches. Mathematical Problems in . . . .
Zonta, T., Costa, C. D., da Rosa Righi, R., and ... (2020).
Predictive maintenance in the industry 4.0: A system-
atic literature review. Computers &Industrial . . . .
ICEIS 2025 - 27th International Conference on Enterprise Information Systems
928