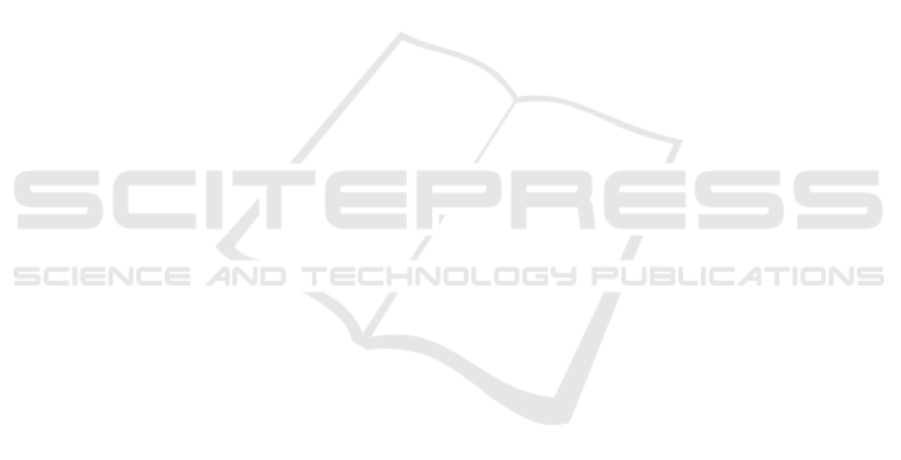
REFERENCES
Abujayyab, S. K., Almajalid, R., Wazirali, R., Ahmad, R.,
Tas¸o
˘
glu, E., Karas, I. R., and Hijazi, I. (2023). In-
tegrating object-based and pixel-based segmentation
for building footprint extraction from satellite images.
Journal of King Saud University-Computer and Infor-
mation Sciences, 35(10):101802.
Afaq, S. and Rao, S. (2020). Significance of epochs on
training a neural network. Int. J. Sci. Technol. Res,
9(06):485–488.
Akyel, C. and Arıcı, N. (2022). Linknet-b7: noise re-
moval and lesion segmentation in images of skin can-
cer. Mathematics, 10(5):736.
ANEEL (2025). Energy loss reports - light in the tariff.
Accessed: January 3, 2025.
Archana, R. and Jeevaraj, P. E. (2024). Deep learning mod-
els for digital image processing: a review. Artificial
Intelligence Review, 57(1):11.
Atas¸,
˙
I. (2023). Performance evaluation of jaccard-dice co-
efficient on building segmentation from high resolu-
tion satellite images. Balkan Journal of Electrical and
Computer Engineering, 11(1):100–106.
Avdan, U. and Jovanovska, G. (2016). Algorithm for
automated mapping of land surface temperature us-
ing landsat 8 satellite data. Journal of sensors,
2016(1):1480307.
Batchkala, G. and Ali, S. (2021). Real-time polyp segmen-
tation using u-net with iou loss.
Chaurasia, A. and Culurciello, E. (2017). Linknet: Exploit-
ing encoder representations for efficient semantic seg-
mentation. In 2017 IEEE visual communications and
image processing (VCIP), pages 1–4. IEEE.
EPE (2024). Balanc¸o Energ
´
etico Nacional 2024. Acesso
em: 30 set. 2024.
Flores, J. L. O. et al. (2023). Consumo de energia e cresci-
mento econ
ˆ
omico no brasil.
Gonzalez, R. C. and Woods, R. E. (2000). Processamento
de imagens digitais. Editora Blucher.
Gorelick, N. (2013). Google earth engine. In EGU gen-
eral assembly conference abstracts, volume 15, page
11997. American Geophysical Union Vienna, Austria.
He, Z., Yao, W., Shao, J., and Wang, P. (2024). Ub-finenet:
Urban building fine-grained classification network for
open-access satellite images. ISPRS Journal of Pho-
togrammetry and Remote Sensing, 217:76–90.
Jiwani, A., Ganguly, S., Ding, C., Zhou, N., and Chan,
D. M. (2021). A semantic segmentation network
for urban-scale building footprint extraction using rgb
satellite imagery. arXiv preprint arXiv:2104.01263.
Khan, A. and Sudheer, M. (2022). Machine learning-based
monitoring and modeling for spatio-temporal urban
growth of islamabad. The Egyptian Journal of Remote
Sensing and Space Science, 25(2):541–550.
Li, S. and Liu, X. (2022). Multi-type road extraction and
analysis of high-resolution images with d-linknet50.
In 2022 3rd International Conference on Geology,
Mapping and Remote Sensing (ICGMRS), pages 244–
248. IEEE.
Montazerolghaem, M., Sun, Y., Sasso, G., and Haworth, A.
(2023). U-net architecture for prostate segmentation:
the impact of loss function on system performance.
Bioengineering, 10(4):412.
Rani, K. P., Rao, K. S., Sreedevi, P., Subbaraju, G. V.,
Dastagiriah, C., and Padmavathi, J. (2024). Land
cover extraction from satellite imagery using u-net
model. In Proceedings of the 11th International Con-
ference on Computing for Sustainable Global Devel-
opment, pages 1361–1367.
Rosa, M., Shimbo, J. Z., and Azevedo, T. (2019).
Mapbiomas-mapeando as transformac¸
˜
oes do territ
´
orio
brasileiro nas
´
ultimas tr
ˆ
es d
´
ecadas. VIII Simp
´
osio de
Restaurac¸
˜
ao Ecol
´
ogica, pages 95–100.
Sirko, W., Kashubin, S., Ritter, M., Annkah, A., Bouchareb,
Y. S. E., Dauphin, Y., Keysers, D., Neumann, M.,
Cisse, M., and Quinn, J. (2021). Continental-scale
building detection from high resolution satellite im-
agery. arXiv preprint arXiv:2107.12283.
Solomon, C. and Breckon, T. (2013). Fundamentos de
processamento digital de imagens-Uma abordagem
pr
´
atica com exemplos em MatLab.
Ullah, S., Qiao, X., and Abbas, M. (2024). Addressing the
impact of land use land cover changes on land surface
temperature using machine learning algorithms. Sci-
entific Reports, 14(1):18746.
Zhou, L., Zhang, C., and Wu, M. (2018). D-linknet: Linknet
with pretrained encoder and dilated convolution for
high resolution satellite imagery road extraction. In
Proceedings of the IEEE conference on computer vi-
sion and pattern recognition workshops, pages 182–
186.
´
Agton de Oliveira, P. (2020). Deep learning na segmentac¸
˜
ao
autom
´
atica de imagens de sat
´
elite. 24 pages. Tra-
balho de Conclus
˜
ao de Curso (Graduac¸
˜
ao) - Engen-
haria Agr
´
ıcola e Ambiental.
ICEIS 2025 - 27th International Conference on Enterprise Information Systems
936